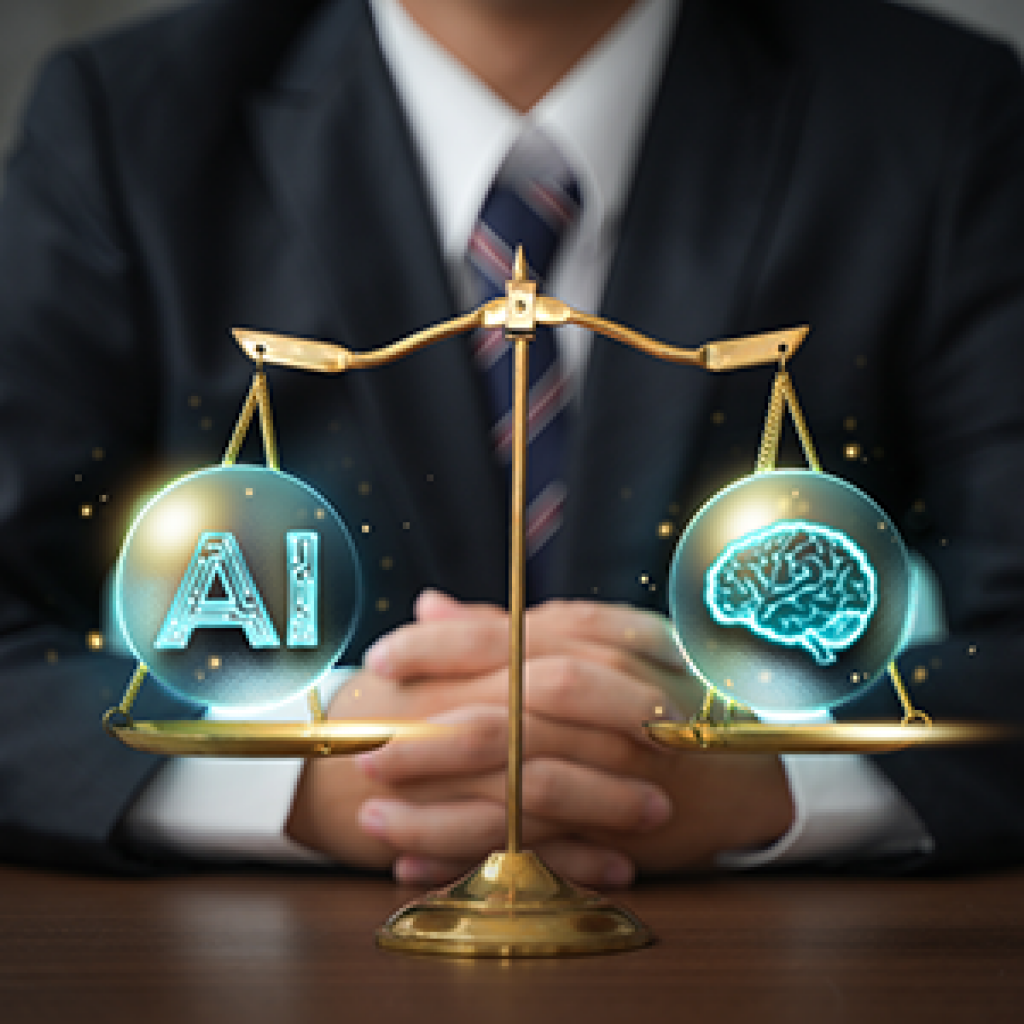
AI governance has become a critical topic in today’s technological landscape, especially with the rise of AI and GenAI. As CEOs express concerns regarding the potential risks with these technologies, it is important to identify and address the biggest risks.
Implementing effective guardrails for AI governance has become a major point of discussion, with a focus on determining the most important measures to ensure safety and compliance. Furthermore, the potential for AI to enhance data quality and governance cannot be overlooked, as creating data trust remains a significant opportunity. Lastly, the role of GenAI in enabling self-service business intelligence raises questions about balancing data accessibility and privacy, particularly regarding personally identifiable information (PII).
Biggest Risks
In recent surveys, CEOs share their concerns about the risks associated with AI and GenAI. The most significant risk, according to experts, is deploying AI without integrity, which can lead to undetected errors in business processes. Incorrect data or models result in wrong outcomes and can damage a brand’s reputation. This damage can be difficult to repair after the fact.
Other critical risks include AI not being reviewed for regulatory compliance and biases introduced through upstream data or the model training process. Effective AI governance must encompass technology, processes, people, and an overarching alignment with organizational values. I spoke with data professionals at #CIOChat to learn more.
BDO security practice leader Wayne Anderson, says, “The biggest risk is deploying AI without integrity that leads to undetected wrong inputs to business processes. Wrong data or wrong model leads to wrong outcome. Brand impact is hard to rebuild. Right behind that are risks like AI not being reviewed for suitability to regulation, or undetected bias from bias in the upstream data or model training process. AI governance should be about tech and process and people. The AI experience of large organizations like healthcare and financial services regard early experiences in AI governance as a differentiating/competitive advantage. The competence curve is real — and surrounding curiosity, nurturing it, directing it, can also be a real growth advantage for CIOs well positioned on incubation and rewarding that innovation in safe ways.”
The experience of large organizations, such as healthcare networks, insurers, and financial services companies, shows that early AI governance can create competitive advantage. However, the competence curve in AI is steep, and nurturing curiosity while directing it towards safe innovation is crucial. Challenges include difficulties in inspecting AI integrity, cybersecurity vulnerabilities, accidental data leaks through public AI, and insufficient budget allocations. Moreover, there is a scarcity of experienced AI professionals, forcing organizations to strike a balance between building and buying AI. Another significant risk is the lack of clear data usage principles and governance. Isaac Sacolick, former BusinessWeek and McGraw Hill Construction CIO, says, “We’re lacking AI/data usage principles and defining easy-to-understand governance for a safe middle ground between complete self-organization and strict lockdowns. When the board / CEO want competitive capabilities faster while many employees are happy to tinker with the latest AI gadget, it creates conditions where small decisions can have significant consequences. We’re starting to see AI governance tools that help automate some of the guardrails. I think what separates AI is from other innovation and emerging tech concerns is it creates serious competitive threats.”
There is a need for a middle ground between total self-organization and strict lockdowns. Boards and CEOs pushing for rapid competitive capabilities while employees experiment with the latest AI tools can lead to small decisions with significant consequences. Emerging AI governance tools should aim to automate some safeguards, but the competitive threats posed by AI are unique compared to other technologies. New Zealand CIO, Anthony McMahon, says, “Inexperienced staff trying to take shortcuts will be the biggest risk. Specifically, how they use the tools, what data they load in to create output, and how they interpret the responses.”
The primary risks of AI and GenAI identified include public tools leaking confidential information, poor data quality leading to incorrect results, and security issues. Additionally, the lack of experience in enterprises and the fear of being left behind exacerbate these risks. Key concerns are the absence of rigorous guidelines on data ownership and usage rights, biases, and dirty data pipelines for machine learning models. Inexperienced staff attempting shortcuts pose a considerable risk, particularly in how they use AI tools, the data they input, and their interpretation of outputs. CEOs’ concerns about AI risks generally revolve around competitive threats, regulatory issues, reputational damage, privacy and intellectual property worries, loss of control, cybersecurity threats, and dependence on AI vendors. Specific technical risks include impersonation, dynamic malware, Trojan horses, feature management, reproducibility, cyber risks in GenAI code, operational support, data quality, application quality, failure to verify outputs, governance challenges, agentic team control, and job security.
Addressing biases is critical, as people may trust the output of GenAI tools without verifying accuracy, potentially leading to the spread of false information. Effective AI governance, rigorous guidelines, and experienced professionals are essential to mitigate these risks and harness AI’s full potential while safeguarding against its pitfalls. Carrie Shumaker, CIO University of Michigan Dearborn, says “Expounding on biases a bit, folks believing the output of GenAI tools to be accurate when it may assert things to be true that are not.”
Implementing Appropriate Guardrails
Implementing effective guardrails for AI governance is crucial to ensuring the safe and ethical use of AI technologies. One of the most important aspects is establishing a clear process for reviewing and funding AI ideas, ensuring that all tools used are designated and controlled to prevent shadow AI from causing security breaches. Data security protocols, including cloud security, must be strictly applied to AI applications, whether they are productized or customized foundational models. Regular reviews of data pipelines for lineage, rights, and privacy are essential, along with predictable and deliberate initial release reviews and ongoing post-release evaluations for new standards.
Automation plays a vital role in safeguarding AI governance. Tools that scan prompts and large language model (LLM) results for confidential and privacy data leakage are increasingly available and should be integrated into AI platforms. This should include platforms that offer built-in privacy and data security measures that align with governance intents. Additionally, organizations must monitor their web traffic to detect unauthorized use of public AI endpoints, such as employees pasting sensitive data into tools like ChatGPT, which can lead to data leaks. Sacolick asks for “Automation to scan prompts and LLM results for confidential and privacy data leakage. Several solutions in the market do this now.”
Constellation Research VP Dion Hinchcliffe adds, “It’s vital to have consistent, effective guardrails for AI.” Without question, the most critical guardrails to implement within AI governance include robust cybersecurity measures, strict access controls, comprehensive data and IP protection, safety mechanisms, accuracy checks, and ethical controls. These elements, coupled with education on appropriate data input and the importance of fact-checking AI outputs, form a strong foundation for responsible AI use. Furthermore, addressing feature management is essential to prevent misuse and ensure that AI applications align with organizational goals and values.
McMahon argues as well, “Because of its potential to be an enterprise tool, the guardrails should be built around the lowest common denominator. Be open about what tools can be used, and what can’t. Educate on what data can be inputted. Encourage fact-checking and proofreading of output. Perhaps most importantly, don’t overcook them. GenAI can be another angle of shadow IT and the harder you make it for people to use, the more they’ll find ways around.”
Creating Data Trust
Creating data trust through AI holds significant potential for enhancing data quality and governance. AI can play a critical role in data cleaning and categorization, offering a real opportunity to improve both aspects. AI’s ability to recognize variability and anomalies in any data type is particularly valuable. It can rapidly surface issues like duplication, which might otherwise go unnoticed by data teams. However, the risk of eroding data quality remains if AI tools are misused or if those using them lack the necessary expertise. McMahon says, “The potential for rapidly surfacing anomalies in data including duplication, being carried out by anyone rather than the data team is high. The potential to further erode data quality because people don’t know what they are doing is equally as high.” Sacolick agrees and says, “I am finding more examples for data governance because of GenAI. Tools for GenAI to easy data governance/quality, not as much. Yet.”
To mitigate these risks, it is essential for CIOs to manage AI access and the re-use of personally identifiable information (PII) effectively. Controls on both input and outcomes must align with the obligations for PII. While AI can be a powerful tool for policing and checking data quality, it is ultimately a highly probabilistic tool with limitations in self-governance. Therefore, additional tools and governance mechanisms are necessary to support AI in this role. Historical data governance efforts often failed due to a lack of care, but with AI presenting a competitive advantage, there is now a greater impetus for organizations to prioritize and refine their data governance strategies. Capgemini Executive Vice President Steve Jones says, “because AI will cause massive damage if you don’t. Most historical data governance efforts failed because nobody really cared. If AI is a competitive advantage, people care.” Anderson argues, “CIOs need to manage AI access and re-use of PII — but that’s not excluding using it to synthesize outcomes. It’s making sure the controls on the input and the outcome are appropriate to the obligations for the PII.”
Delivering Self Service BI
Delivering self-service BI through GenAI presents significant potential, but it also raises critical questions about handling personally identifiable information (PII). CIOs must ensure that PII is locked down and appropriate access controls are in place, which ideally should have been established well before the advent of GenAI. The degree of access and the specific rules depend on the industry and regulatory environment, emphasizing the importance of education to prevent breaches. Staff need to be aware of what data they can share and why.
Sensitive data can be anonymized and locked down to ensure it can be reused safely. The use case dictates the approach: For instance, in the realm of protected health information and AI for cancer identification, early models required anonymization for development but could not be anonymized in application. This highlights the necessity for robust AI governance and integrity inspection. All data access should be aligned with corporate policies, with delegated authority and propagated identity as the norm, though this is often not the case. Self-service GenAI demands the same stringent protection of PII as other IT systems. While security models from AI vendors increasingly address these needs, the challenge of maintaining good AI governance remains. Investing in getting this right is crucial to mitigate risks and leverage the full potential of GenAI in delivering self-service BI. Sacolick asks, “Should CIOs lock down PII or make sure appropriate access exists?” Uh yeah. They should have done this well before GenAI.” Manufacturing CIO Joanne Friedman goes further and says, “Lock it down and anonymize any sensitive data regardless of its origin so it becomes reusable. Jones agrees and concludes, “Everything should be locked down and matched against corporate policies. Delegated authority and propagated identity should be the norm. Sadly, it’s normally system accounts all the way down.”
Parting Words
Implementing self-service BI through GenAI offers great potential but necessitates stringent data governance. CIOs must lock down PII and establish robust access controls, tailored to industry regulations. Education on data privacy is critical to prevent breaches. Sensitive data should be anonymized to ensure safe reuse. Effective AI governance requires consistent policies and delegated authority. Despite advances in AI vendor security models, protecting PII remains a challenge. Investing in proper governance and security measures is essential to harness GenAI’s benefits while safeguarding data integrity.