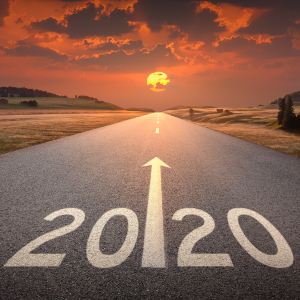
2019 was a major year for AI— from innovations in design and ethical advancements to the increased demand for AI solutions. So, what lies ahead in 2020?
Fractal Analytics is one of the largest AI providers in the world, with clients like Visa, Colgate Palmolive, Kimberly-Clark, and more.
Some of their top AI experts have compiled a list of predictions for 2020 – see below! They share their insights on everything from the Industrial Internet of Things (IIoT), to combatting “deep fake” technology, to the creation of more people-centric AI.
– – – – – – – – – –
Eugene Roytburg
Managing Partner, Strategic Center
- There have been numerous AI (or actually, ML) related use-cases in 2019 and lots of lessons learned. One of these is that we are still at very early stages of ML usage, especially in business applications, and that we need to treat this phase as we did with many new methodologies that came before – identify the right problem, test a new approach, identify its applicability, iterate until clear advantage (vs. prior methods) is established.
- AI (ML) will be more clearly defined as a part of broader analytics and will have better-defined application areas and value creation. Many companies have been confused about the two, particularly how and if they are connected. While the companies pushed for many ML tests and use-cases mostly driven by “artificial” pressure, the “traditional” analytics development took a back seat.
- Lots of individual ML use-cases will give ground to better, well thought-out usages of “traditional” advanced analytics vs. ML.
- In 2020, most companies will come to their senses and consider the AI “craze” not as a singular development, but more connected to overall analytics strategy and transformation to optimize their existing analytics efforts while setting up the right infrastructure and governance to expand AI potential.
- Analytics quality and value metrics will become the mainstream KPIs to guide future analytics/AI development and investment.
- RPA and Cognitive Process Mining will become even more important for both back office (accounting) and functions (sales, marketing, product development) because it will drive more efficient process execution and also optimize and even totally change how these processes will function in the future.
- Analytics/AI activation/adoption: Convincing employees of why they should use analytics/AI will become the main focus and the center of all analytics/AI development and investment.
- A true human/machine interaction: Leveraging both objective data and analytics-driven insights, while at the same time incorporating human intuition and experience to learn from both worlds.
Soudip Roy Chowdhary
CEO, Eugenie.ai
- The world economy is going through a pivotal moment with the introduction of Industrial 4.0 and associated technologies. Artificial intelligence, the Industrial Internet of Things (IIoT), will continue to play an important role in this new revolution while associated technology, like 5G or blockchain, would act as catalysts for its wide adoption across different domains and functions.
- Cyber-physical systems and digital twins would make both remote monitoring and control possible for physical assets. Advancement in hardware (sensors and smart chips) and software/middleware (edge computing) would make such digital transformation implementation feasible within enterprises.
- Design continues to play an important role in ensuring the wide adoption of intelligent systems to augment and extend human capabilities within organizations. 2020 is going to see a new era of the human-machine confluence with further progress in the areas of deep learning, especially in the reinforcement learning and generic adversarial networks or remote process automation.
- Adding to it, the adoption of the AI techniques research community would continue to be invested in finding out new techniques by which they can explain the algorithmic decisions – transforming the black-box approach of AI to glass-box.
- Privacy would continue to remain a concern for consumer-facing AI applications. We would see a substantial rise in research efforts related to building a privacy-aware AI ecosystem and enabling fairness in AI algorithms.
- Partner-centric businesses are going to be the new norm of business and technical advancement. ISVs and technical partners who would provide off-the-shelf niche solutions on top of existing platforms (hardware, computing, storage, etc.) would see a steeper adoption curve within enterprises. They would influence the new technology norms within industries.
John LaRocca
Managing Director, Europe/NA Operations
- The demand for AI solutions will continue to outpace the availability of AI talent, and businesses will adapt by enabling more applications to be developed by non-AI professionals, resulting in the socialization of the process. Non-AI practitioners, such as knowledge workers and analysts (who are not skilled AI practitioners but have great domain expertise), will start to develop rudimentary applications aided by automated AI engines. The onus will be on corporate training programs to retrain/upskill these new practitioners and on IT to enable them with automated AI environments that use AI itself (e.g., machine learning apps to help develops train models without having to write code). This is not unlike the historical lifecycle of analytics, and it will similarly benefit everyone in the ecosystem – businesses will expand their capacity to develop and benefit from AI apps, AI experts will be working on truly leading-edge applications and tie newly upskilled non-AI practitioners who will contribute more and have more marketable skills.
- Consumers will gain ground in the fight for their right to own and monetize their personal information. They are already being rewarded to some degree in the form of discounts, premium services and other perks from sharing their personal information, but, for the most part, they have few rights in the process and certainly no ownership of their data. The future will see them test the waters to take more control of the process and receive even direct monetary compensation in exchange for the data they provide. This will disrupt the mega-billion-dollar consumer data marketing industry, which will have to figure out how to deal with the consumer getting a part of that pie and fuel the birth of intermediary businesses that facilitate the exchange of data for compensation between the consumers and the businesses that want to access and use their data.
Suraj Amonkar
Fellow, AI @ Scale, Machine Vision and Conversational AI
- Building better AI: The AI community will continue to make progress in building algorithms that train faster, train with lesser data, and generalize better. The use of newer algorithms for data augmentation, few-shot/zero-shot learning will make the cumbersome, deep-learning training process easier and developments in feature representations and generative networks will work towards making models more generalizable. The use of complex/hybrid series of algorithms to achieve tasks will help build models that scale for complex “real-world” scenarios. The use of self-supervised methods will hasten the progress of generic models. The availability of generic “out-of-the-box” models in machine-vision and NLP will continue to evolve fast – but the need for building customized models for real-world challenges would remain. The use of multi-agent systems would evolve with the need to move towards more generic intelligence capabilities.
- Making AI really work: The use of both cloud-based and edge-based technologies would expand, and both these ecosystems would cater to use-cases that make sense for them. The use of micro-services frameworks, auto-scaling, and containerization would continue to deliver scalable AI ecosystems, and the use of edge devices would help bring real-time use-cases alive. Hybrid deployment models would be required in solving many of these complex deployments, and the use of in-memory and distributed storage/processing frameworks would continue to power AI systems increasingly.
- Built-for-AI hardware: The increase in the development of special-purpose AI chips and hardware would allow tighter integration on the system. The use of built-for-AI hardware would open vast possibilities to the amount of processing power that AI algorithms can leverage and would provide a major leap towards next-generation algorithms and systems.
- Safe, governed, ethical AI: The AI community will continue to debate and progress on the challenge of governance, privacy, safety and ethics in AI. Work on privacy-preserving algorithms and similar techniques might provide some technical answers to these tricky questions. The evolution of some open standards or systems to tackle these issues might accelerate in the near future.
Sandeep Dutta
Chief Practice Officer, APAC
- 2019 saw a clear shift in enterprise resources towards getting the data strategy right before launching large AI projects. Many companies focused on creating enterprise data lakes on the cloud that can help get good, reliable quality data sets in place. We are expecting to see this trend accentuate in 2020, with many centralized analytics CoEs first focusing on getting the data right.
- More and more analytics CoEs will be challenged to prove the ROI and impact of the solutions they create. Organizations will look at incorporating design thinking to ensure that the user is in the middle and problems that are important for the business get prioritized, thereby improving the adoption of the AI and analytics solutions. The operationalization of the solution will be as important as solution development.
- 2020 will see a renewed focus on innovating brain-computer interface with a focus on thought-controlled machines. As better, less intrusive sensors emerge for capturing electrical signals when neurons communicate, AI & ML will play a big role in deciphering these into thoughts and actions that a machine can interpret and perform.
- The debate on data standards and regulatory framework around AI will intensify. In January 2020, the CCPA will come into force and we can expect that other states in the US will follow with their versions of privacy laws. We can also expect regulatory guidelines to start coming from other regions in the world. The AI industry will need to rally around and create self-regulation that establishes guidelines ahead of the regulators swinging into action.
- Deep fake technology will continue to evolve very rapidly and will emerge as a big challenge for everything from the entertainment industry to politics. Apps like “Zao” from China, have already demonstrated the rapid pace at which deep fake is progressing, and this will pick up even more speed in 2020. The AI industry needs to fight back with better algorithms to detect deep fakes.
Parameswaran Venkataraman
Chief Design Officer
- People-centric AI: Until now, the narrative about AI has largely been about how AI will replace human beings. In reality, several applications of AI are focused on augmenting and improving the way human beings work, rather than replacing their work. And therefore, focusing on the ‘people’ (not just ‘users’) who will use or be impacted directly / indirectly through the applications of AI, will become more important. This will mean focusing a lot more on understanding people, their current scenarios, behavior and needs, and how AI can help them in their goals. This will also mean designing AI solutions that are simple, intuitive, and delightful in their experience.
- Ethics in the design of AI: While there has been a lot of talk around the role of ethics in the way AI is being used and delivered in various contexts, more often than not, these conversations are post-facto. In the next year, organizations will start using ‘Ethics in AI’ to drive the way new applications of AI are conceptualized and designed from scratch. Given people’s awareness and expectations from issues related to how they use (or are used by) technology, designing for ethical AI will become the norm eventually.