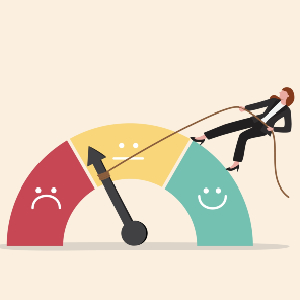
If you look at Google Trends, you’ll see that the explosion of searches for generative AI (GenAI) and large language models correlates with the introduction of ChatGPT back in November 2022. GenAI has brought hope and promise for those who have the creativity and innovation to dream big, and many have formulated impressive and pioneering ideas given this new opportunity. Still, there is a large gulf between having a new, innovative idea and putting it into practice in your organization. You need to get your data AI-ready.
Strategic Goal
It is so exciting that the conversation around AI has turned from sheer possibilities to implementation. The interest has not quit, which suggests that the payout is worth the effort. While the technology, computational power, and access to data that allow for these large language models are new, the mathematics are not. Similarly, the business questions that surround the implementation of this newest “shiny thing” are also not new. GenAI is no different than other data-centric business initiatives. Start with the strategic goal in mind: What is the company set out to accomplish and how may GenAI be of service in accomplishing that goal?
Starting Point
A strategic goal is set and GenAI is integral to the proposed solution. What’s the gap between the end state and the starting point? What are the risks? What are the costs? What’s the expected return? What’s the timeline? What skillset is required? Build in-house or work with a partner? It is only possible to answer these questions by knowing the current state of your data quality. It is only possible to measure the distance from beginning to end by knowing your starting point.
Garbage In, Garbage Out
GenAI can lift your business to new heights but there is a key dependency — it feeds on the input data. Feed it data of poor quality and your output will be poor. Good quality data is an absolute necessity to trust, utilize, and stand on the insights provided by AI.
Here are a few specific data quality dimensions to start with to ensure your data is of good quality and AI-ready. You’ll notice that these are not the top-of-mind dimensions that many consider the bedrock: accuracy, completeness, timeliness. Those dimensions are important to measure, but if you are setting up a new GenAI program, I argue that you must ensure you have even more basics included in your new program.
Definitions from DAMA-NL
You’ll find the italicized definitions for each dimension in DAMA-Netherland’s research paper, “Dimensions of Data Quality (DDQ)” by Peter van Nederpelt and Andrew Black:
Dimensions
Compliance: The degree to which data is in accordance with laws, regulations, or standards. How is the use of your data now changing? Do you need to uphold your data to higher standards and different requirements in these new use cases? Consider also: A true disruptor like GenAI may result in the need for new policy, and therefore, new regulation. Can you anticipate future regulation around AI usage given your industry and data? Measuring, preparing, and improving the data quality to meet anticipated future standards puts you ahead of the game.
Accessibility: The ease with which data can be consulted or retrieved. Scalability and repeatability require consistent accessibility. Is your data reliably accessible by the right people and technologies? How accessible must it be? If your data was temporarily inaccessible, how damaging would that be? What is the acceptable threshold that would ensure your project will succeed?
Access Security: The degree to which access to datasets is restricted. Consider the privileges and permissions to your data and the implications. Are you building an AI tool in-house or are you using a service? Which of your company’s data are you willing to provide third party access to? Ensure that you are not sharing data that you cannot or should not share. What controls do you have in place? Consider also how access security and accessibility intersect. Can you have high accessibility and high access security?
Traceability: The degree to which data lineage is available. Traceability within AI is one of the main critiques of the technology. Don’t add to the problem by introducing data of low traceability. Of all the data you intend to feed to AI, can you communicate how that data came to exist? Is it in the same one-to-one form as its origin? If not, can you speak to the transformations that occurred within the data supply chain?
Interpretability: The degree to which data are in an appropriate language and units of measure. Of course, the expectation is that GenAI will create new insights and so there is a level of acceptance that the insights buried in the data are not fully understood. But don’t let that fool you into believing that low data interpretability is acceptable. If you can’t understand your data before feeding it to AI, there’s no chance you’ll have a better understanding of the outcome and the insights from AI. If you feed coded values to AI, you must also feed the reference materials that interpret those codes into meaningful information. When data interpretability is low, GenAI insights will be exponentially lower. When data interpretability is high, GenAI insights could be ground-breaking, eye-opening, and life-changing.
Coverage: The percentage of units not belonging to a population or missing from the target population. Keep in mind, the data you feed into GenAI will be the basis for your return information. If you only feed information about say, non-profit organizations, can you extrapolate the output to represent all non-profit and for-profit organizations? Consider how you may need to account for classic statistical biases: sampling bias, response bias, survivorship bias, etc. Your input data must have adequate coverage of all types of entities that you expect your output to represent.
The list of integral data quality dimensions could go on and on which accentuates just how important high-quality data is to the success of the implementation of any AI, any data-centric program at your organization.
Not on the List
Completeness: The degree to which all required data values are present. An understanding of every data quality dimension’s measurement is important to some degree and completeness is no exception. It is one of the most often referenced, frequently measured, and easily understood data quality dimensions. You’ll want to meet some minimum threshold to ensure quality output, but if you have a firm basis on other data quality dimensions, AI could be a great tool to account for certain holes in completeness.
What strategic goal will AI help you meet? First and foremost, let your goals dictate which are the most important data quality dimensions to measure. Know your starting point — measure your data quality. This will largely dictate if your AI initiatives will be successful.