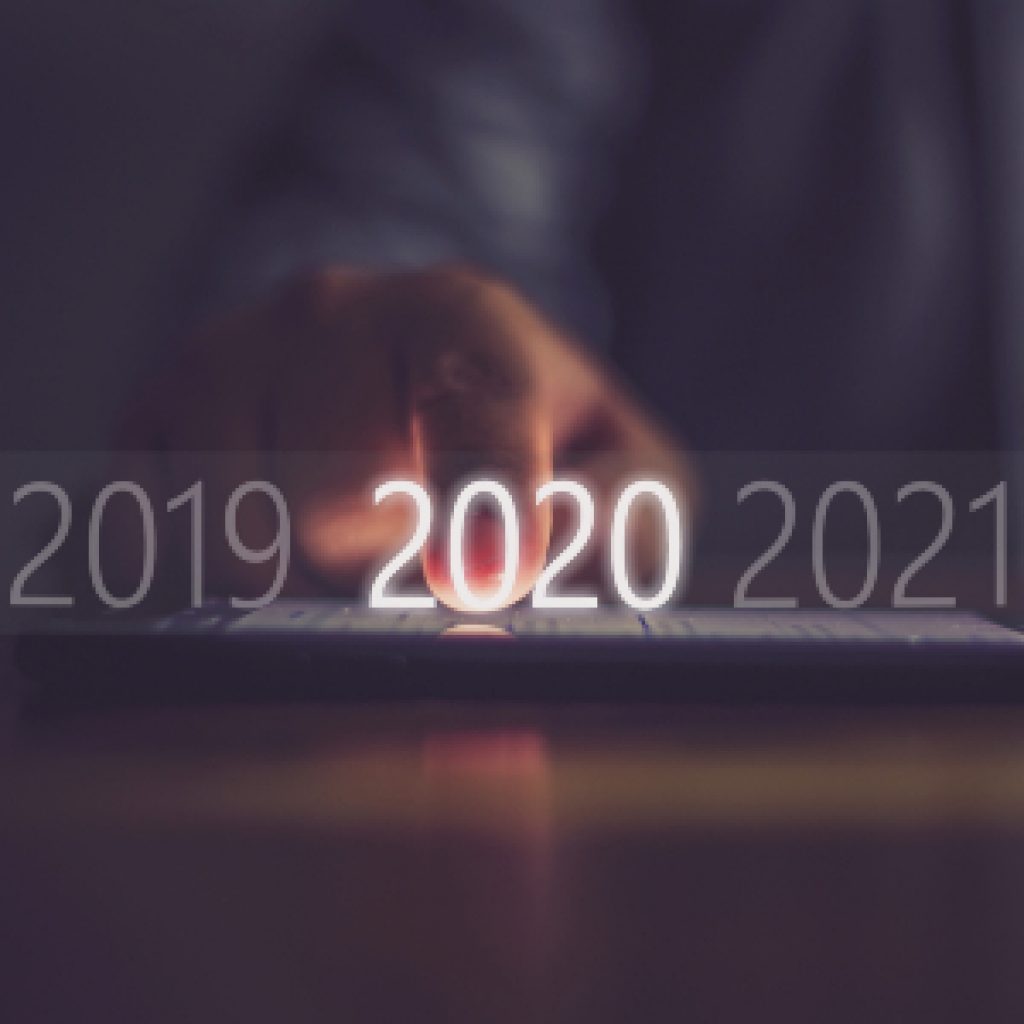
It was the best of times.
It was the worst of times.
Has there ever been a time where more people were talking about data and writing about data than now, as 2020 and a new decade begins?
Data analytics is hot. Every company wants to be data driven and every business and individual is trying to figure out what the California data privacy law means to them.
—————————————–
It’s easy to find headlines like the following:
“Data Front and center at the NSF [National Science Foundation] in 2020.”
“California rings in 2020 with landmark data privacy law.”
“The 10 Hot Data Analytics Companies To Watch In 2020.”
“Cars now run on the new oil — your data.”
This should be an exciting time to be a data governance practitioner. There is certainly more data than ever to govern. We’ve all seen the numbers and they are impossible to comprehend: Nicole Martin, Digital Marketing Manager, Sonic Healthcare USA, wrote in “How Much Data Is Collected Every Minute Of The Day”(Forbes, August 7 2019), that, per software firm DOMO’s annual report, “Overall, Americans use 4,416,720 GB of internet data including 188,000,000 emails, 18,100,000 texts and 4,497,420 Google searches every single minute.”
That’s just Americans!
There’s not just quantity. There’s variety – structured data, semi-structured data, unstructured data, and social media data. There’s distributed data and centralized data, data in Clouds and blockchains, and graph databases. Data streaming, data encryption, data scientists…and most important, data analytics, and that droning mantra for companies to be “data-driven.”
Surely, having data governance to assure data quality, compliance with regulation, and adherence to data policies and standards has to be critically important. Companies are certainly recruiting heavily for data governance roles, include senior positions.
And yet…
Ryan Gross, Vice President, Emerging Technology at Pariveda Solutions, published an excellent article in May 2019 in Medium, “The Rise of DataOps (from the ashes of Data Governance)”. It is extremely perceptive – plus it has some striking images, like a tombstone with the epitaph “Data Governance – 1998-2016 – We Always Needed You but Never Wanted You”.
Having spent the last 10 years in Data Governance leadership roles in financial services, my first reaction was “Ouch!”. Nothing against Ryan – he is brilliant, and he and I had a great discussion a few months back about this topic. And I do think DataOps is very promising and will write more about it later.
But in any case, after I recovered, I realized that the title aligned with thoughts I’d been having over the past year. DevOps had been introduced at my last place of employment and senior management suggested watching the Spotify squad framework YouTube videos. All fascinating stuff – “squads”, “tribes”, self-forming teams…but hardly a mention of data, let alone data governance.
CI (Continuous Integration) and CD (Continuous Delivery) drastically decrease the lifespan of software development from months to minutes…but what does that mean to governance? How can we keep up with data lineage, and data quality, when everything changes so quickly?
Then there’s unstructured data, the creation of which surely outpaces that of structured data. How do we even think of those venerable data quality dimensions – accuracy, completeness, consistency, timeliness, validity, uniqueness, etc. – for emails, documents…let alone Twitter.
When I think about how I approached establishing a data governance framework six or seven years ago, the key milestones – establishing a data governance council, setting standards, identifying data stewards and data custodians, determining CDEs, setting data quality metrics, capturing lineage…these all seem to be relevant. But the deliberate, thoughtful, and time-consuming approach that I and most data governance professionals I know used doesn’t have a place when, to quote DataKitchen, businesses demand analytics at Amazon speed (https://medium.com/data-ops/analytics-at-amazon-speed-the-new-normal-cb9508cc00e6).
So, are we entering a new era for Data Governance? In his excellent article, “A Brief History of Data Governance”, (June 25, 2010), Winston Chen postulates the governance of data went through three distinct periods, the Application Era (1960-1990), the Enterprise Repository Era (1990 – 2010), and the Policy Era (2010- ?). Chen went on to found Voice Dreams, a top-selling app assisting students with learning disabilities.
Briefly summarizing Chen’s insights, during the Application Era, data was treated as a byproduct of transactions, governed to some extent through enterprise data models. In the Enterprise Repository Era, organizations began to value data beyond its role in transactions and sought to exploit data through building large scale repositories. The recognition of the importance of data describing core business entities led to the development of master data management. Gradually, the understanding developed that data governance was critical to success, but governance was typically siloed around individual enterprise repositories.
By the 2010s, the explosion of data had begun, and business’s use of data grew more sophisticated, outrunning the capabilities of enterprise repositories. Companies took a policy-centric approach to data models, data quality standards, data security, and lifecycle management. This made it acceptable to store the same type of data in multiple places as long as the same policies were adhered to. “Enterprise repositories continue to be important, but they’re built on governed platforms integrated with enterprise data policies.” Most importantly, business recognized data as an enterprise asset and took greater responsibility for its content.
This approach certainly helped companies, especially in financial services, address the new concerns of regulators following the financial crisis of 2007-2009. Regulators were particularly focused on data quality and lineage, as well as Know Your Customer, so data governance organizations concentrated on these areas, implementing tools and procedures and often working in tandem with Internal Audit and Compliance to tighten data controls. It’s not coincidental that the position of Chief Data Officer rose to prominence in the early 2010s, especially in the financial sector, in part so the C-suite could visibly demonstrate how critical data was to their organizations.
The Policy Era also provided the framework needed to manage other types of data beyond that which resides in relational database. With data lakes and data streaming, conventional data quality procedures were stretched but the overriding policies did help practitioners provide guidance to developers and users of these innovative technologies. In short, the Policy Era was characterized by tightening controls on data through enterprise-level data governance and the need to leverage the governance framework to understand the exponentially-growing data volume.
Chen’s description of the Policy Era is very consistent with my own experiences. The problem, I believe, is that just as the enterprise repository era was overwhelmed by data proliferation, the data governance frameworks developed during the Policy Era cannot keep up with the speed of technological and data analytic changes wrought by the revolution in software development, Cloud technology, and similar innovations. Regulation, a primary driver of the growth of Data Governance over the past decade, is changing rapidly, racing to keep up with technologies like FinTech, AI, and social media, especially in the area of data privacy.
This plainly requires an innovative approach to building data governance frameworks that are both extremely flexible, even, dare I say, agile, as well as comprehensive. In fact, the challenges are such that I believe data governance will be commencing a new era in response. But in what direction do we proceed? A clue comes from a surprising source – Eliyahu M. Goldratt’s wonderful book, “The Goal”.
I just finished reading this classic, amused to have found it in the Fiction section of a NYC Library branch. Chris Bergh of DataKitchen and Gene Kim, George Spafford and Kevin Behr, authors of “The Phoenix Project”, have all cited “The Goal” as an inspiration for their work in DataOps and DevOps, respectively. For those not familiar with the book, Goldratt using storytelling to develop ideas on applying the scientific method he learned as a physicist to manufacturing. As he writes in his Introduction, the principles of the scientific method, of postulating assumptions and using them to explain phenomena, can be extremely useful outside the traditional sciences to all types of organizations and processes.
Goldratt, as embodied in his book as the protagonist’s physics teacher (the protagonist is a manager trying to avert a plant closing), poses a question, “What is the goal of the manufacturing plant?” The answer Goldratt is looking for is not obvious, but, once the plant manager comes up with it, the goal becomes the hypothesis on which further analysis is based, ultimately leading to a complete revamping of plant processes and measurements (No, I am not going to give away what The Goal is – read the book!).
I read the book while struggling with the question of Data Governance today, as we enter the 2020s. I thought that a good place to begin might be to ask myself the same question Goldratt did about the plant: what is the goal of Data Governance?
Now, I am sure you are thinking that the answer to that can is easy to find, what with the many Data Governance-dedicated websites, and thousands of articles. Indeed, a Google search for “What is the Goal of Data Governance” yields multitudes of results. On the Data Governance Institute’s site, “Goals and Principles of Data Governance”, you can find the following “typical universal goals of a Data Governance Program:”
- Enable better decision-making
- Reduce operational friction
- Protect the needs of data stakeholders
- Train management and staff to adopt common approaches to data issues
- Build standard, repeatable processes
- Reduce costs and increase effectiveness through coordination of efforts
- Ensure transparency of processes
All of these have merit, but if, as Goldratt suggests, we should identify one goal and evaluate actions, processes and metrics in terms of whether they further the goal or not, which of these would we pick? Are any of these unique to data governance, and do any inspire the type of novel thinking needed to reshape Data Governance for its fourth era? As someone with a true passion for data governance, I find none of these inspire my creativity or serve as a call to action.
Goldratt found that his training as a physicist sparked inventive ideas on manufacturing. Although I understand the scientific method, I am not a physicist or trained in any scientific discipline. I distinctly recall that when my father, a physics major in college, talked about the laws of physics, I fell asleep. But if Goldratt found his education and training gave him new perspectives about industrial organizations, perhaps I needed to do the same to explore data governance with fresh eyes.
I studied classical music as an undergraduate. I majored in music performance (cello is my instrument), and pursued music as a full-time career for some years, until I decided having to take whatever musical gig I could made playing the cello too much like work.
What can classical music tell us about Data Governance? When I asked myself Goldratt’s question – what is the goal? – the first word that came to mind was “structure”. Data Governance certainly is all about providing a structure for managing data…but that in itself is not a meaningful goal. But reflecting on structure and considering it from the perspective of my classical music training, led me to, at once, one thought – a particular type of musical structure called a fugue.
More precisely, I thought of a musical work of the famous composer and the greatest writer of fugues ever, J.S. Bach. One of his last compositions, left unfinished when he died, was The Art of the Fugue. It’s a collection of 20 fugues, all based on the same melody, or theme, for an unspecified instrument or instruments.
What in the world does this have to do with data governance? Well, as I hope to show in my next article, Part II of three, “Data Governance and The Art of the Fugue”*, this music represents how a musical structure can provide a way for multiple, disparate streams of notes to be combined in a multitude of complex ways and be understood and enjoyed by listeners. Far from stifling creativity, the structure inspires Bach’s musical invention. I believe the parallels to governing data in today’s world are quite strong.
*I promise to fully explain in clear, laypersons’ terms what a fugue is in my next article, complete with examples. But in the meantime, this article by Stephen Johnson is a very good introduction to the subject: http://www.classical-music.com/article/what-fugue.