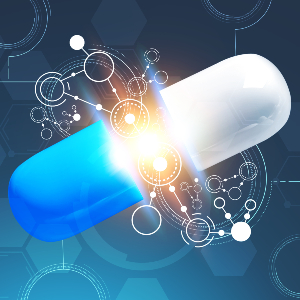
Data supply chains in pharma and life sciences are generally long and complex. They typically involve multiple business units, both inside and outside a given enterprise. This impacts reference data in particular because its management is very distributed, leading to the increased need for downstream integration as well as overall redundancy. Although it might seem the answer is to centralize reference data, this is not always practical. There are other ways in which distributed reference data can be managed successfully. We acknowledge that this federated approach can be a challenge and will be successful only with correspondingly federated governance organizations.
This article is based on our previous white paper “Managing Data as a Product with Distributed Reference & Master Data,” which contains more detail on the approach we are going to describe here.
Reference Data is Managed in Many Distributed Sources
Reference data in pharma is created in many different places and with varied functions. The creation of information happens in different institutions and along the translational value chain, going through early research, development, clinical studies, regulatory processes, production, marketing, and finally the observation of evidence in the real world. Reference data in early research is usually based on public literature and information created by scientific institutions. Partnering entities, such as Contract Research Organizations (CROs), are involved in early research and clinical studies. As part of the process, clinical study and regulatory affairs data are legally required to be based on reference data from authorities (such as the EMA in Europe and the FDA in the USA). Ultimately, the foundational workflows that eventually lead to a life science product involve the collection and reuse of data by many different systems.
The functions that fulfill specific tasks along these workflows are often oblivious to the need for using specific reference data. Depending on the function, creating the same or similar reference data values (such as a new indication) might happen in a strictly regulated environment, as well as in environments where IDs and labels for data can be freely chosen. Thus, it is necessary for the particular function to recognize that it is handling shared and distributed reference data. This involves opening up for sharing labels and codes with other systems, or ingesting and reusing codes from other functions and even from external organizations.
Although functions handle the same kind of data – such as records on regions and countries, species, indications, and drugs – this information is used in different contexts and often requires different levels of detail or granularity. Thus, data entities are viewed from different perspectives and have different roles, depending on the function and workflow (this requires different attributes and metadata). In this context, it is crucial for different data producers and users to align on the workflows for the creation of data (and on the conditions of data use) along the value chain.
It is absolutely vital to split data silos between the different functions. It minimizes costs and efforts when using interfaces for transferring data between functions. Satisfying the demands of regulatory authorities is another chief concern, and this may involve multiple functions in the product’s value chain.
The Three Top Reference Data Challenges in Pharma
All of this adds up to a number of challenges. We consider the top three to be:
Missing Awareness and Redundancies
Internal and external functions are not aware of existing reference data and reference data standards. An so, alignment is necessary in order to enable integration and translation (mapping) between the sources of reference data, if multiple standards are required. This lack of awareness leads to reference data being reinvented, which in turn unintentionally creates data silos. In the worst case, functions are aware of other systems, but claim to provide the reigning reference data while ignoring other data or standards. Examples of this are the different functions inside the enterprise, such as R&D, production, Regulatory Affairs, Real World Evidence, as well as the work with CROs.
External Authorities
Authorities require particular detailed information from functions, which is often unavailable due to a lack of sharing and alignment of reference data. Thus, such demands may result in massive data wrangling and integration efforts along the value chain. Diversity of regulatory requirements in different regions and product categories add to the complexity of this scenario.
Workflow Integration
Since many different functions need to adapt, extend, and enhance information, it is crucial to align and maintain reference data in a way that enables the subsequent orchestration of data distribution and sharing workflows. This must happen without impeding processes that also require the reference data in other functions.
By comparison, master data entities, such as “product” or “study,” are managed in a distributed manner; this is not going to change. It happens because people work in their specific business applications when capturing or processing data. In this case, switching to another system, or requesting the creation of a new master entity through a managed service, is often not feasible for business users. Moreover, such a system is often not available to them, nor they do not have access to it.
How Can We Deal with Distributed Reference Data?
Nowadays, many enterprise data strategies aim to centralize reference and master data managementin order to regain control. Though there are legitimate reasons and interests for centralization, the reality is that centralized management alone is too slow to accommodate the fast-changing IT and data landscape. Pure centralized approaches fail to support the perspectives of different business units and the corresponding incompatibilities found in large organizations. Nevertheless, both aspects are increasingly important in an era of digitalization and increased need for collaboration across enterprise boundaries.
The answer is to create a registry for distributed reference and master data. This can serve as a discovery solution and provides reliable access for anyone in an organization to interact with reference data managed in different applications. What kind of functions should such a registry have? We believe they must include:
- Global lookup service that allows searching for any term or code used within the enterprise. This will allow data stewards to find the preferred terminologies for a given domain, encouraging re-use instead of re-creation.
- Persistent Identifiers that can be generated to provide long-term stable and resolvable IDs that function as data references that users and applications can rely on – even when the location where the reference data is managed changes. Persistent IDs are required for FAIR data (FAIR is a standard and conceptual approach to data management that is very significant in pharma).
- Matching reference data. It is important for aligning existing terminologies that are already in use. This is something that would have to be implemented via technology, but it has been implemented in Master Data Management products for years. Admittedly, reference data is not master data, but the overall approach would seem to be extendable to reference data. Of course, no technology is perfect and there will be a need for data stewards to review and approve potential matches before such mappings become available. The opportunity for machine learning (ML) in this is obvious.
- Public standard terminologies. They already exist (examples include the Gene Ontology or the NCBI taxonomy and are easily accessible. Therefore, there is no obstacle to including these also in a central registry, so that all internal consumers are using the same up-to-date and validated version.
These are four key functions that will enable distributed reference data to be managed. They will require some advancement in technologies to manage reference data, but we already see these functions implemented in other metadata tools. So, it is probably just a matter of time before they are utilized for reference data management. Successfully addressing distributed reference data will remove a major headache for pharma and life science and open to the door to increased data-driven innovation in these industries.