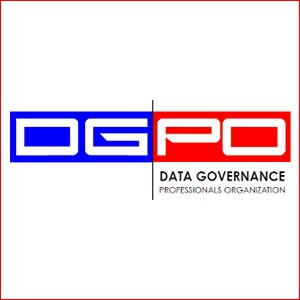
There is an ever-increasing awareness of concerns about data privacy, corporate data breaches, increasing demands for regulatory compliance. There are also emerging concerns about the ways that big data analytics potentially influence and bias automated decision-making.
Individuals are starting to pay attention to organizational vulnerabilities that compound risks associated with managing, protecting, and enabling access to information, ranging from poor data quality, insufficient methods of protecting against data breaches, inability to auditably demonstrate compliance with numerous laws and regulations, in addition to customer concerns about ethical and responsible corporate use of personal data.
And as organizations expand their data management footprints across an increasingly complex hybrid multicloud environments, there has never been a greater need for systemic information risk management.
In a recent webinar for the Data Governance Professionals Organization (DGPO), I covered a number of aspects of information risk management. In this article, we propose a working definition for information risk, provide some examples, describe a set of categories of information risk, and then suggest that incorporating a framework for information risk management that augments and expands an existing data governance program will deliver value to your organization.
According to Wikipedia,[1] there are a number of definitions of risk, including
- “Risk is the potential for uncontrolled loss of something of value.” and
- “(Exposure to) the possibility of loss, injury, or other adverse or unwelcome circumstance; a chance or situation involving such a possibility.”
In general, “risk” affects the way that a business operates in a number of ways. At the most fundamental level, it inhibits quality excellence. However, exposure to risks not only has an effect on project objectives, but it also poses threats of quantifiable damage, injury, loss, liability, or other negative occurrence that may be avoided through preemptive action. Using the Wikipedia definition as a start, we can define information risk as “the potential for loss of value due to issues associated with managing information.”
Information risk is often used to refer to data protection, particularly with respect to data breaches and regulatory compliance. This makes perfect sense, as both have significant organizational impact. For example, in the 2019 “Cost of a Data Breach” report done by the Ponemon Institute and IBM,[2]
- The
global average cost of a data breach was $3.92 million.
- The average size of a data breach was 25,575 records.
- The average cost per record was $150.
- The average time to identify and contain a data breach was 279 days.
Some recent examples of costly data exposures include the 2018 hacking of Marriott’s reservation system that exposed private data of over half a billion customers, the 2019 incident involving First American in which personal data was accessible via public servers, and the 2020 Russian hacking in which multiple US agencies were attacked. The vulnerabilities allowing a corporate data breach pose operational financial risks (costs of detection and system remediation and notification), the risk of brand erosion that can cause customer attrition and lost new business, and long-term costs related to post-breach customer identity theft monitoring.
Although protection of an individual’s personal and private data is certainly a critical facet of information risk management, it is valuable to consider a broader perspective of different categories of information risk, such as:
- Data Loss. Data lossfocuses on those
scenarios in which information contained within data assets is no longer available
or accessible. The risks include:
- The inability to use services that access a data asset,
- Damage or corruption of electronic data assets or to the physical asset used to store the data,
- Inadvertent data deletion,
- Communication or network failures that compromise transmitted data,
- Inability to access data stored in a format for which no hardware is available.
- Data Retention. Data retention policies define the length of time that the organization needs to retain information. There are two types of retention risks. The first is that the data sets are not retained for a long enough time may lead to concerns about auditability and compliance. The second is that data sets are retained for too long, which can not only lead to increased data management costs, but also the potential for increased legal exposure during a discovery phase of litigation
- Data Awareness. Data awareness is the enablement of data consumers across the organization to know what data assets are managed, what information those assets contain, and data asset availability and accessibility. When there is limited data awareness, the organization may be subject to inadvertent data replication and correspondingly, inconsistency among data sets. Furthermore, in the absence of data awareness, individuals may not know whether certain data sets are available, or where critical data assets are managed.
- Data Usability. Data usability encompasses conformance to standards and meeting data quality expectations. Some of the vulnerabilities include operational failures, missed revenue opportunities, and failure to properly execute data-dependent business processes.
- Data Exposure. The risks of exposure of sensitive data to data consumers that are not authorized to view that data include failure to comply with a wide range of data privacy laws, as well as loss of reputation and brand erosion, leading to loss of strategic advantage and inability to compete.
- Compliance. More generally, because more and more regulations and industry standards are data-dependent, there is a compliance risk that involves the inability to auditably demonstrate that the organization is complying with externally-imposed regulatory, legal, or contractual requirements. Aside from the expected failure to comply with privacy protection laws, there are different types of compliance requirements for managing information chain of custody or producing required reports in a timely manner.
- Administrative Risks. Administrative risks are associated with the governance processes for information risk management. Inadequate training about information risk management can lead to increased operational and management costs when there are violations of information policies, particularly when the absence of data awareness increases the difficulty of incident management and remediation.
Recognizing the types of information risks is the first step in establishing policies and procedures that can broaden the enterprise-wide perspectives on good information management practices. This is one of the types of knowledge and skills that one would learn at University of Maryland’s certificate program in Information Risk, Privacy, and Security (CIRPS). Instituting an information risk management framework adds value to the organization by:
- Simplifying reporting through auditable processes for data asset assessment and classification simplifies compliance reporting,
- Building trust by demonstrating auditable processes for protecting personal and private data builds trust with your customers,
- Enabling automated monitoring using specifications of data sensitivity that allow for automated application of data protection policies,
- Reducing corporate risk by expanding knowledge of the data landscape to apply data protection applications (such as encryption and masking), and
- Raising data awareness by sharing knowledge in the “hidden” areas of the data landscape and gaining insight into corporate operations and business opportunities.
[1] See: https://en.wikipedia.org/wiki/Risk
[2] Downloaded from: https://www.all-about-security.de/fileadmin/micropages/Fachartikel_28/2019_Cost_of_a_Data_Breach_Report_final.pdf
This quarter’s column was written by David Loshin, President of Knowledge Integrity, Inc., (www.knowledge-integrity.com), a recognized thought leader and expert consultant in the areas of data management and business intelligence. David is a prolific author regarding business intelligence best practices as the author of numerous books and papers on data management, including Big Data Analytics: From Strategic Planning to Enterprise Integration with Tools, Techniques, NoSQL, and Graph and The Practitioner’s Guide to Data Quality Improvement, with additional content provided at www.dataqualitybook.com. David is a frequent invited speaker at conferences, web seminars, and sponsored websites and channels. David is also the Program Director for the Master of Information Management, the Certificate in Information Risk, Privacy, and Security, and the Game, Entertainment, and Media Analytics programs at the University of Maryland’s College of Information Studies. David can be reached at dloshin@umd.edu.