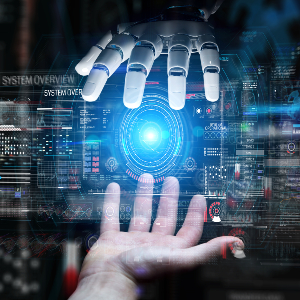
The business case for data governance has been made several times in these pages. There can be no disagreement that every company and every government office must have a data governance strategy in place. Establishing good data governance is not just about avoiding regulatory fines. The data economy is growing, and data-driven organizations will dominate the business landscape.
In response, organizations have moved rapidly to control the structured data contained in databases or used in systems of record such as CRM, ERP, HR, and others. According to industry experts, this accounts for 15-20% of enterprise data. But what about the other 80-85% known as unstructured data?
Unstructured data includes the documents, files, emails, scanned images, and other file types scattered across file shares, local hard drives, web servers, email servers, archives and legacy document management systems. Most organizations simply do not know what is out there: PII in the wild; documents containing company secrets; messages that violate ethical standards; and more. What they don’t know can and often will hurt them.
This problem cannot be solved by simply moving all this data into a cloud document management system. The unstructured data in every organization includes as much as 50% redundant, obsolete and trivial (ROT) data which should never be stored in a system of record. Do you really need to have 1,000’s of invitations to a 2004 Holiday party? Are you sure you want to keep an employment offer letter that contains personal financial information such as wages, stock and other PII to be out in the open? Unstructured data lacks tags and labels – the intelligent metadata which the cloud DM system will require in order to properly manage the content.
The solution to the unstructured data governance problem is to use a content analytics platform to analyze and understand every file and provide notifications of sensitive data that should be under control. Content analytics has been around for over ten years, making use of basic AI methods such as machine learning and natural language processing to classify these files and extract data that can be used for security, governance and compliance. A content analytics platform is most often used as the front-end for file migrations to cloud ECM, for records management systems, and for compliance systems.
New innovations in AI deep learning methods, coupled with cheap and limitless cloud computing power, have led to powerful new unstructured data management solutions that can now go far beyond a reliance on standard metadata or OCR, to actually understand documents and make decisions about their meaning. Deep learning is also being used to automate the training of classification models and greatly reduce the Human in the Loop (HITL) factor. What previously may have required dozens of subject matter experts (SMEs) and months of work to review and label a petabyte of unstructured data can now be accomplished by a few SMEs in a matter of days.
What to Look for in an Advanced Content Analytics Solutions
From our long experience working with end users, we can offer a checklist of key questions to ask as you consider a content analytics platform.
- Does it mix and match AI methods to discover which works best on a specific project? Some solutions lean on machine learning algorithms and OCR while others try deep learning visual methods. Look for a solution that has a toolbox full of AI algorithms and methods, and can intelligently decide which method best suits the data corpus.
- Does it handle both born-digital and scanned documents? Analyzing born-digital text files is reasonably straightforward. However most organizations also have troves of scanned files with no useful metadata and no idea of what sensitive data is locked inside. Look for a solution that is not limited to OCR and deploys visual AI methods to process scanned images with higher accuracy than OCR only products. The solution should use neural network classification with transfer-learning.
- Can it accurately locate the data anywhere in a document? Most analytics software use rigid rules and regular expressions which are limited and will miss data in unstructured documents. Look for a solution that uses AI deep learning visual methods to find sensitive data like PII in any location within a document.
- Does it identify and isolate ROT for disposition? Useless, duplicate and expired files can use up to 50% of your storage costs. Look for a solution with proven ROT identification capabilities.
- Can it accurately extract data from less-than-ideal images? Organizations often find folders full of images that were skewed during scanning, or were faxed, and are very hard to read by traditional OCR methods. Look for a solution that uses AI fuzzy-rules data point detection and extraction and visual anchor points to increase extraction accuracy and reduce human intervention.
- Are the AI results explainable and transparent? There is a legitimate concern surrounding the use of AI to make data decisions. Some AI models are black boxes that hide the decision-making process. How was that decision to decline a loan application made? Why was that group of documents marked as ROT? Look for a solution that can document how each data decision was made by the AI models.
- Does it scale to handle Petabytes of data? It is no longer unusual for us to meet companies with at least one petabyte of unstructured data. Make sure the solution can scale and efficiently run all of its functionality on large data spaces.
With the help of the latest AI innovations, unstructured data is easier than ever to organize and prepare for inclusion in your data governance program. Now is the time to consider your unstructured data governance situation and take action to close the gap.