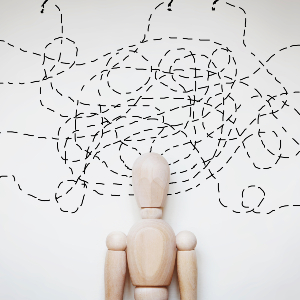
Increased data generation requires modern businesses to manage vast volumes of information. All this data holds immense potential for insights and informed decision-making, but its value depends on effective utilization. Without the right tools, frameworks, and strategies, even established companies risk being overwhelmed by data overload.
Let’s take a closer look at data overload and how to identify and address it.
What Causes Data Overload?
Data overload occurs when an organization ingests massive quantity of data, making it difficult to process or analyze it. This happens when the volume, variety, and velocity of data exceed the ability of a company’s data infrastructure to effectively process it.
Signs an Organization Is Experiencing Data Overload
Recognizing the signs of data overload early is key to avoiding bottlenecks, ensuring efficient data handling, and maintaining analysis accuracy. Here are some common indicators:
- Inconsistent or conflicting reports: Different teams produce reports with mismatched metrics or conclusions due to fragmented data sources.
- Slow decision-making: Leadership struggles to make timely decisions because extracting actionable insights from data takes too long. This can lead to missed opportunities and the business losing its competitive edge.
- High storage costs: The organization spends excessively on storing redundant or irrelevant data without utilizing it effectively.
- Missed insights: Important trends or patterns are overlooked because the sheer volume of data obscures critical information.
- Frequent system slowdowns: Overburdened data systems experience performance issues, delaying processes and increasing downtime.
The Impact of Data Overload
The consequences of data overload extend beyond just slow decision-making and inefficiency. Some additional impacts include:
Reduced Employee Morale
Data overload often shifts employees’ focus from strategic work to repetitive tasks like data cleansing, sorting, and validation. The additional workload consumes time and adds to frustration, especially when the tools provided are inadequate for managing such tasks efficiently. Over time, this can lead to burnout, disengagement, and higher turnover rates, creating additional challenges for the organization.
Increased Risk of Errors
Managing excessive data manually or with outdated systems significantly heightens the likelihood of errors, such as incorrect entries, missed updates, or flawed analyses. These mistakes can lead to costly financial consequences, such as inaccurate billing or reporting, and operational setbacks, like delays in decision-making or supply chain disruptions. Left unchecked, such errors can decrease trust in the organization’s data processes and overall efficiency.
Inability to Scale
As data volumes expand, organizations lacking a reliable data management infrastructure find it increasingly difficult to scale their operations effectively. Inefficient systems and fragmented data pipelines create bottlenecks, slowing down processes like analytics, reporting, and decision-making. This hampers the organization’s ability to innovate, respond quickly to market demands, and adopt new technologies, ultimately stifling growth and leaving them vulnerable to more agile competitors.
Addressing Data Overload: Strategies for Success
Despite being a serious challenge, data overload isn’t insurmountable. With the right strategies and tools, organizations can regain control over their data and leverage it properly.
Here are a few ideas:
1. Implement Data Management Solutions
Investing in a reliable data management system is always a smart choice. By implementing a central repository or data warehouse, organizations can consolidate their data in one place, making it easier to access and manage. These systems ensure that data is stored in an organized manner and is easily retrievable when needed.
Moreover, automated data management solutions eliminate the need for manual sorting and processing. For example, an end-to-end data management platform can automatically extract, collect, clean, classify, and process data from different sources before storing it in a data warehouse, reducing the time and effort needed for data handling.
2. Adopt Data Analytics Tools
Organizations should consider using advanced data analytics tools to help process large datasets and extract meaningful insights. These tools use artificial intelligence (AI) to identify trends, make predictions, and highlight areas for improvement in real time.
Data analytics tools also help automate decision-making processes, providing actionable insights and recommendations. These tools can reduce data overload while improving the accuracy and speed of decision-making.
3. Invest in Intelligent Document Processing (IDP)
Another effective way to address data overload is by incorporating intelligent document processing (IDP). IDP systems can help organizations automate the extraction and processing of data from documents such as invoices, contracts, and forms.
IDP utilizes machine learning algorithms to recognize and classify information from unstructured documents, transforming it into structured data that can be easily stored and analyzed. This reduces the manual effort involved in data extraction, improves data accuracy, and significantly speeds up document processing. By automating this critical part of data management, organizations can handle a higher volume of information without succumbing to overload.
4. Create a Data Governance Framework
To prevent data overload, organizations must establish a strong data governance framework. This framework should outline data ownership, access policies, and procedures for data quality management. By setting clear guidelines for how data is collected, stored, and shared, organizations can ensure that their data remains manageable and valuable.
Moreover, implementing data governance best practices, such as regular data audits, ensures that the organization stays compliant with regulations and maintains data integrity. A solid governance framework also prevents data silos from forming, which is a common cause of data overload in large organizations.
Additional measures can supplement effective data governance. Some best practices include ensuring that data collection is purpose-driven instead of arbitrary, enforcing clear data retention policies, and performing regular data cleanups to get rid of irrelevant data.
5. Prioritize Data Quality Over Quantity
Gathering data in the hopes of uncovering insights leaves an organization prone to data overload. Businesses should instead create a well-defined data collection strategy that aligns with their specific goals. Clearly identifying what data is needed and why ensures that only relevant and actionable information is collected.
Establishing strict guidelines for data collection can also help minimize unnecessary data. For example, setting clear parameters for data types, sources, and formats can prevent the accumulation of redundant or irrelevant data. Regular reviews of data collection practices further ensure that an organization adapts to changing needs and avoids collecting excess data that doesn’t serve its objectives.
Summing Up
Companies can regain control of their data by identifying the signs of data overload early and adopting effective strategies. The key is to integrate automated tools into their data stack, prioritizing streamlined data management. By improving data access, integration, and analysis, these tools help maximize efficiency and empower better decision-making.