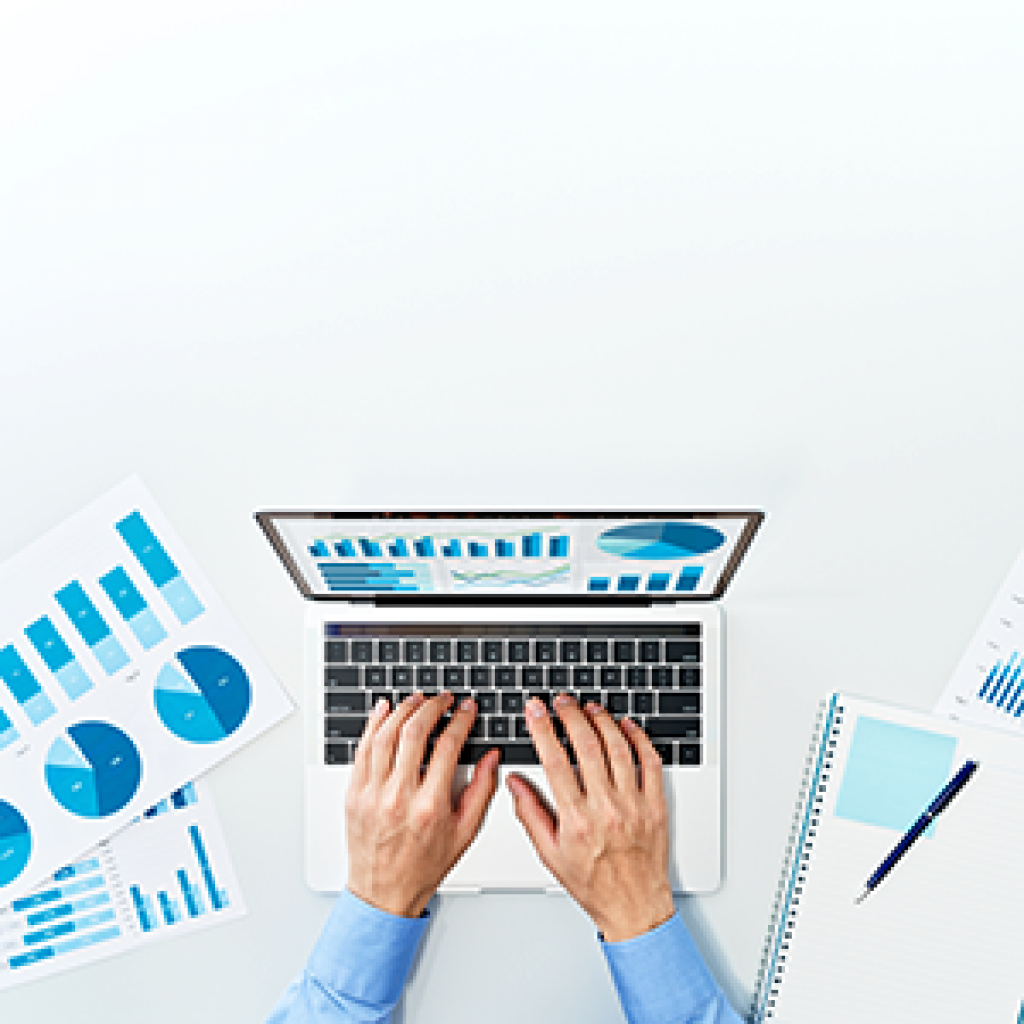
Data often arrives from multiple sources in inconsistent forms, including duplicate entries from CRM systems, incomplete spreadsheet records, and mismatched naming conventions across databases. These issues slow analysis pipelines and demand time-consuming cleanup. Organizations now use machine learning-assisted data preparation to address these challenges, which automatically standardizes formats, detects anomalies, and applies business rules.
Data professionals also apply advanced preparation techniques to streamline collection, cleaning, and analysis. These methods reduce manual work, limit errors, and establish clear paths from raw data to business insights. As a result, companies that adopt structured data preparation methods often halve their analysis cycles and provide analysts, managers, and executives with reliable data for strategic decisions.
Understanding Data Wrangling as a Foundation
Data quality issues cost organizations millions in missed opportunities and flawed decisions, which is why data wrangling is used to boost analytical accuracy across departments and data sources. With methodical consistency, teams clean, combine, and structure information using established protocols that catch errors early and create reliable datasets.
After that, analysis teams can then pull verified data within minutes instead of spending hours checking spreadsheets and reconciling numbers. Understanding the core elements of data wrangling and its role in business intelligence helps teams move from basic spreadsheet management to sophisticated data operations.
Core Components of Data Wrangling
Data wrangling starts with collection and profiling steps that map data characteristics, identify patterns, and spot inconsistencies. The process filters outliers, standardizes formats, and merges related information from different sources. Automated quality checks scan for duplicate entries, validate relationships between fields, and flag potential errors before they affect analysis results.
Advanced data wrangling tools apply machine learning algorithms that learn from past corrections and automatically suggest improvements to data structure and organization. These systems remember common data transformations, propose standardized naming conventions, and maintain detailed logs of all changes for compliance and auditing purposes.
From Raw Data to Business Intelligence
The initial data preparation phase sets up all subsequent analysis work. Clean, consistent data structures speed up queries, reduce false conclusions from bad data, and let analysts focus on finding insights instead of fixing formatting issues. Well-organized data also makes it easier to spot trends and patterns that might remain hidden in messy datasets.
A systematic data wrangling process helps teams collaborate effectively on analysis projects. When everyone works with standardized, verified data, they spend less time reconciling conflicting information and more time testing hypotheses and generating actionable recommendations. This shared foundation of homogenous, reliable data supports faster, more confident decision-making across departments.
Leveraging Automation and Integration
Manual data preparation tasks consume valuable time and introduce human error into analysis workflows. Fortunately, automated tools and their data insights can slash processing time while maintaining quality standards. Recent advances in machine learning have created opportunities for more sophisticated automated data handling that adapts to new information sources and changing business requirements. Both established software solutions and custom implementations now offer practical ways to automate complex data preparation tasks across industries.
Modern Tools for Data Preparation
Python scripts and specialized data preparation applications now handle tasks that once required teams of analysts. These tools detect data types automatically, suggest appropriate cleaning operations, and apply corrections across entire datasets with a single command. Built-in validation rules check for logical inconsistencies and flag potential problems before they affect downstream analysis.
Smart automation tools learn from corrections and adjustments made by data specialists. They build libraries of common data transformations, recognize patterns in how different teams prep their data, and suggest improvements based on past successes. This institutional knowledge speeds up future projects and helps maintain consistency across departments.
Real-World Examples
Organizations across sectors have streamlined their data operations through targeted automation initiatives. Companies report significant improvements in data accuracy and processing speed after implementing automated preparation workflows:
- Manufacturing companies combine production metrics, quality readings, and maintenance records.
- Financial services firms reconcile transaction data across global systems.
- Healthcare providers merge patient records from multiple departments and facilities.
- Retail businesses integrate online and in-store customer interaction data.
- Supply chain operators consolidate shipping, inventory, and vendor information.
- Educational institutions combine enrollment, academic, and administrative data.
The Role of Micro-Content in Data Presentation
Complex datasets often overwhelm decision-makers with excessive detail and competing metrics, which is where micro-content comes in. Breaking data into micro-content helps teams absorb information quickly and act on specific insights. Data professionals now split analysis results into focused segments that address distinct business questions while maintaining connections to the broader context. Success with this method depends on precise data segmentation techniques and understanding how each user group interacts with information.
Breaking Down Complex Data Sets
Data preparation methods now include steps for creating targeted views of large datasets. Analysts identify key performance indicators and design compact visualizations that highlight specific trends or relationships. These focused snapshots give managers exactly what they need without forcing them to wade through extensive reports or multiple dashboard screens.
Small data segments also make it easier to spot data quality issues and unusual patterns. When examining bite-sized portions of information, teams quickly notice outliers, missing values, and inconsistencies that might get lost in larger data views. Taking a granular approach to data quality helps preserve high standards whilst speeding up the review process.
Optimizing Data Visualization for Decision-Making
Well-designed micro-content presents clear choices for decision-makers. Each data segment answers specific questions about business performance, market trends, or operational efficiency. Teams create hierarchies of information that let users drill down from high-level summaries to detailed analyses when needed.
Micro-content also makes data more accessible to different audience types. Technical teams get the needed detailed numbers, while executives see clear summaries with actionable insights. This flexibility helps organizations build data-driven cultures where everyone uses information appropriate to their role.
Final Thoughts
Modern data preparation methods do more than speed up traditional cleaning processes. Automated tools catch errors that manual review might miss, while micro-content approaches make complex information accessible to different audience types. Data teams that upgrade their preparation processes spend less time fixing errors and more time uncovering valuable insights.
Clear, accurate data leads to confident choices about business operations. Advanced data preparation techniques cut analysis time from days to hours, reduce costly errors, and give decision-makers the precise information they need without unnecessary complexity.