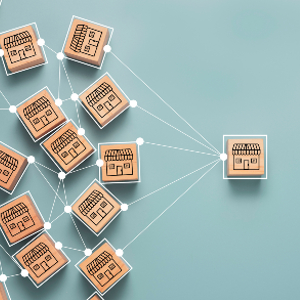
Something has been bothering me about data products. It’s the point at which the consumer of a data product immediately switches to also be a producer. We talk a lot about the binary roles of either consumer or producer when in reality the vast majority of people are both at the same time.
I know data product and data mesh purists may argue that data products are more formal and it should not be possible for someone to easily create a derivative set of data from original sources and call it a data product. There are some solid and valid points in that argument, but the problem is, we don’t live in a pristine world and my focus is meeting organizations where they are and designing a path forward.
Data Reality
Where are most organizations? I contend that most are at a point where their general population is becoming more capable and the pace and volume of their data use are skyrocketing. That plays out in many ways. For example, querying data, plopping it in a spreadsheet, manipulating it, and then immediately sharing it with colleagues. Replace query with ‘get it out of a BI report’, replace spreadsheet manipulation with ‘notebook’, and replace spreadsheet with a zillion tools. The point is that data product derivatives are being created constantly whether we like it or not and whether we approve of them as ‘official’ data products or not.
If we are honest with ourselves, we will admit that this is part of a growing, unintentional data subculture. I define an unintentional data subculture as the organic and informal socialization and sharing of data knowledge across the organization. The subculture is not formally recognized or led.
Interestingly, it’s happening at the same time data and analytic leaders are trying to do the opposite and intentionally create a data culture. It’s not completely clear how to do that, but the data mesh approach, of decentralizing responsibility for data product creation to domains, is one way we can try to flip the script.
One of my goals is to be able to identify, empathize, and help different personas who are playing this dual role of producer and consumer in this subculture. In order to accomplish that, I first had to think more about how they interact with data.
Breaking it Down to the Basics
Often when I have a problem to solve, I revert to the basics we all learned in high school English. The who, what, why, when, and how.
For the problem of the data subculture and the dual role people play, I teased out the following:
- Who = Personas/roles such as business manager, data scientist, data analyst, executive, etc.
- What = Data and data-related assets personas produce and consume. The ‘what’ also includes the business process to which the data assets pertain.
- Why = This is the business benefits and goal behind the creation of and use of specific data assets.
- When = Frequency and lifecycle of asset creation and use.
- How = This is the access, provisioning, tooling, deployment, and distribution of assets (both created and consumed).
Planting this seed of understanding, I believe we can start to identify the core processes and supporting tooling/platforms that accelerate people’s work. I see these are the backbone of a formal data culture.
Introduction to the Data Consumption & Production Cycle (DCPC©)
I readily admit that the definition above of the who, what, why, when, and how is abstract. In fact, after I stepped away from it for a few days and returned, I found myself confused about how I would use it in a practical way. What I decided I needed was to codify it as a detailed framework that would aid my thinking. I call the framework, the Data Consumption & Production Cycle (DCPC©).
DCPC© represents the stages that personas move through, either consciously or unconsciously, with data in order to get their work done.
Before I move into its definition, I do want to confess that I am the first to lose patience with the number of superfluous and self-serving frameworks we have in the data world. The last thing I wanted to do was pile on with something that was not adding value. So, I’ve checked myself numerous times.
Mostly I thought about existing asset lifecycle models and frameworks. Plenty of good ones exist for both physical and digital assets. Is DCPC© different from them or redundant?
The conclusion I reached is that asset lifecycle models focus on the treatment of the ‘thing’, whereas DCPC is focused on the activities of people. In other words, DCPC© is more about understanding the process that people go through so we can identify with it and try to figure out how to support and optimize it.
DCPC© Definition
The definition of DCPC© is pretty straightforward. It’s a series of stages as defined below. The little dashed line represents the logical starting point.
Stage | Description |
Trigger Event | These are compelling events or reasons that trigger the need for people to work with data. These can be: – Recurring and expected such as quarterly reporting. – Exceptions such as poor manufacturing production performance – Speculative such as the desire to increase customer cart volume with complimentary product promotions |
Seek/Find | People search for data that fits the requirements of the triggered event. This includes things like looking in the file share, emailing a friend, querying a database, looking through documentation, and searching a catalog. |
Understand | People try to understand if the data they located is complete, authoritative, and trustworthy. Hopefully, they have easy access to things like lineage, quality scoring, and certification. Most of the time this does not exist so people ask colleagues who they trust. |
Improve | Directly improving data and descriptive metadata or communicate with someone with the authority to do so. |
Access | Gaining access to data. This can be as trivial as opening a spreadsheet or non-trivial, such as a formal request requiring explicit approval and provisioning. |
Create/Develop | People use data to create sets of derived data or a view or representation of the same data. For instance, business managers can create a new spreadsheet by combining data. Data engineers may use a pipeline to transform data. Data and its descriptive metadata can be classified as a variety of abstract types such as a report, spreadsheets, and data products. |
Test | Testing occurs for everything that is created. Some testing is formal and used by teams. Other testing is informal and may not even be thought of as testing by an individual. For instance, a data scientist who creates a new notebook will make sure it’s working as intended even if it’s only for their use. |
Share | Data is often created with the intent to share it with others. Even when it is not proactively shared, it will often be shared on request. |
Maintain | Maintenance of a data asset is dependent on its trigger event. If its use is expected to recur it’s more likely that it will be maintained and held to a measurable standard. |
Learn | What a person learns from the data often leads to another trigger event (exception, speculation) and the cycle begins again. |
The cycle is, well, circular, and appears sequential. But of course, in real life, it’s not. People will cut across skipping stages, repeat stages, etc. That is one of the really exciting things about using DCPC© to analyze what people in the data subculture are actually doing.
Wrapping It Up (For Now)
It’s a bit unfair to stop now because knowing what it is, is only half of the equation. Knowing how to use it is the other half. Watch for part deux, where I will explain how you can apply it to user journeys and data culture archetypes.