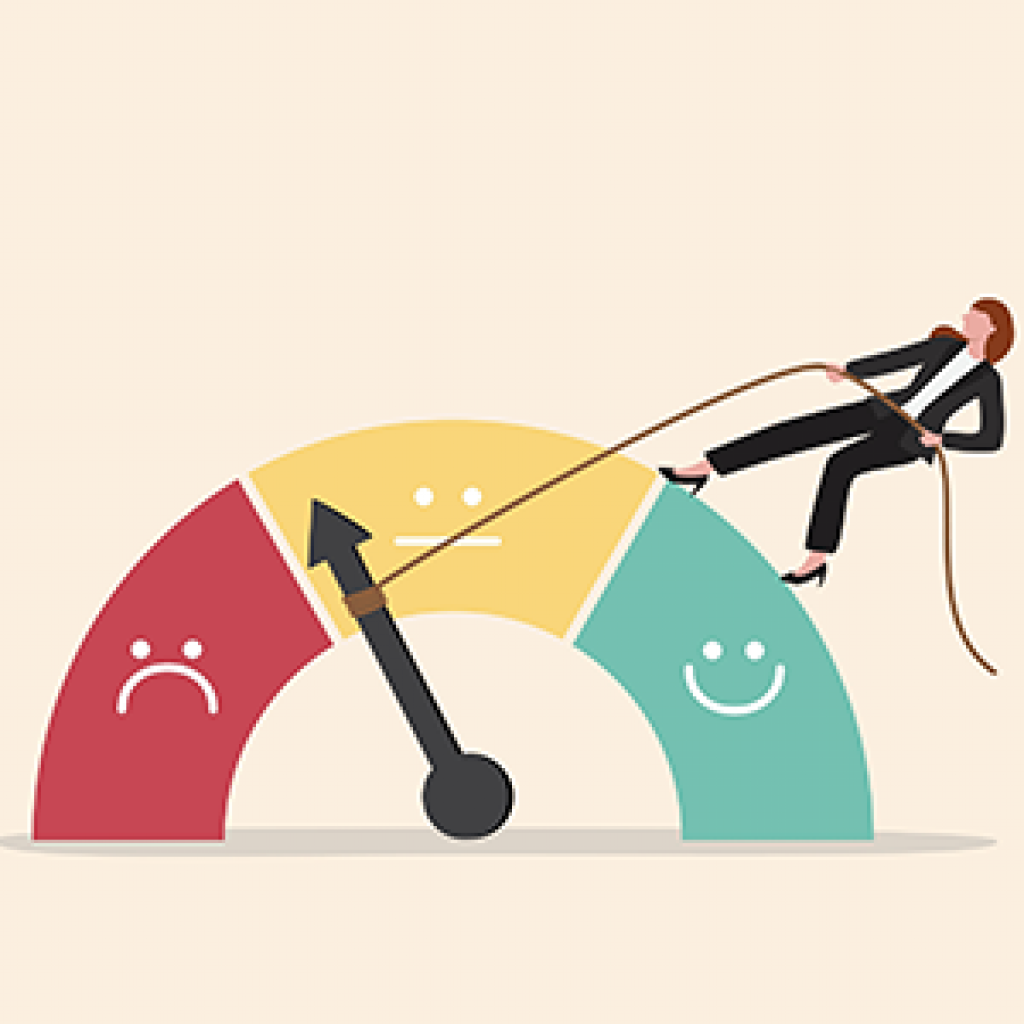
Running a business with dirty data is like trying to drive a car blindfolded — it’s only a matter of time before disaster strikes. Dirty data doesn’t just create inefficiencies, it drains resources at an astonishing rate. Gartner reports that organizations lose an average of $9.7 million annually due to dirty data. In fact, in the United States alone, poor data quality costs businesses a staggering $3 trillion annually.
Even worse, dirty data directly affects customer experience. 93% of consumers report receiving irrelevant marketing communications, and 85% of customers say they are less likely to engage with brands after a poor experience — often caused by inaccurate data. For businesses, the cost isn’t just financial. Dirty data damages relationships, leading to churn and lost trust.
The Scale of the Problem
The Hidden Cost of Inaccurate Customer Data
Dirty data — from manual errors, obsolete information, or disconnected systems — often creeps into business operations without notice until the damage is done. It can lead to wasted resources, lost revenue, and missed opportunities. A recent study shows that sales and marketing departments waste up to 32% of their time dealing with data quality issues instead of focusing on growth.
The cost of inaccurate data is substantial on a financial level. Companies experience a 15-25% revenue loss due to poor data management, with marketing campaigns particularly vulnerable to wasted spending. Furthermore, according to Dun & Bradstreet, organizations suffer from 546 hours of productivity loss each year because of time spent cleaning and fixing data.
Reputation and Trust
Inaccurate data doesn’t just lead to inefficiencies, it has severe consequences for brand trust and customer relationships. For instance, 40% of executives report that poor data quality damages customer satisfaction, driving up churn and reducing long-term loyalty. Customers today expect brands to deliver accurate, personalized communications, and businesses risk alienating their audiences when they don’t.
Common Sources of Dirty Data
Where Does Dirty Data Come From?
The sources of dirty data are often overlooked but are surprisingly common across businesses. Understanding these origins can help companies take the first step toward maintaining clean, reliable information.
Manual Errors
Human error is one of the most frequent sources of dirty data. Whether through simple typos, inconsistent data entry, or lack of standardization, manual errors introduce inaccuracies into systems. For example, an incorrectly entered email address or phone number can prevent businesses from reaching customers, resulting in missed sales opportunities.
Outdated Information
Customer data can quickly become obsolete as people change jobs, relocate, or update their contact preferences. A staggering 30% of data becomes outdated annually, meaning companies could work with highly inaccurate information without regular updates. This can wreak havoc on marketing campaigns where targeting the right audience is crucial.
Siloed Systems
Data silos — where information is stored across disconnected systems without proper integration — are another major culprit. When data isn’t synchronized between departments, it leads to conflicting records and duplicated entries. This lack of integration causes teams to question the validity of their insights, ultimately slowing decision-making processes.
A Playbook for Data Hygiene
The Playbook for Data Hygiene: Prevention and Cure
When tackling dirty data, businesses must combine proactive prevention measures with ongoing efforts to clean and maintain data integrity. Below is a playbook incorporating real-world examples of how these strategies can improve data quality.
Prevention (Building a Culture of Data Hygiene)
- Standardization: Standardization is a critical first step to clean data. Implementing consistent data entry practices across all departments minimizes discrepancies. Companies can reduce manual errors and mismatches by standardizing fields like names, addresses, and contact information.
Real-world example: McKinsey reported that businesses using standardized data processes saw a 35% decrease in data retrieval time and a 40% increase in data accuracy. Uniform data handling allows these companies to improve customer interactions and streamline marketing efforts. - Training employees: Employee training is crucial for maintaining data hygiene. Regular, ongoing education about proper data management ensures that staff consistently follow best data entry and handling practices.
Real-world example: In 2018, a costly data entry error occurred at Samsung Securities when an employee mistakenly issued 1,000 company shares instead of ₩1,000 per share in dividends. This “fat-finger” error resulted in distributing 2.8 billion shares to employees, massively exceeding the company’s total available shares. The mistake cost Samsung around $300 million as they scrambled to recover the shares and mitigate the fallout. This incident underscores how even small data entry mistakes can lead to massive financial losses, emphasizing the importance of clean, accurate data to prevent costly errors. - Data governance plan: A formal data governance framework ensures accountability and consistency in managing data quality across the organization. This includes establishing policies and assigning data stewards to oversee data practices.
Real-world example: Walmart, one of the largest global retailers, leveraged data governance to streamline its supply chain. By standardizing data across its extensive network of suppliers, warehouses, and retail locations, Walmart improved inventory management, minimized stockouts, and enhanced the overall efficiency of its supply chain. Integrating consistent, high-quality data allowed for better coordination between all operation parts, significantly improving product availability and reducing excess inventory.
The Cure (Cleaning Up Existing Data)
- Data audits: Regular audits identify outdated, duplicated, or incorrect data. Audits allow companies to review their databases comprehensively to keep customer information current and accurate.
- Automated tools: Automation tools can significantly improve data hygiene by flagging discrepancies, updating records in real time, and enriching incomplete data sets. Automating these processes reduces the burden on employees, allowing for more efficient and accurate data management.
- Third-party data cleansing: Outsourcing data cleansing to third-party providers can help businesses cross-verify and update customer information more efficiently. These providers often have access to broader databases, improving the accuracy and depth of the data.
- Data deduplication: Using deduplication software helps businesses automatically detect and eliminate redundant records. By consolidating duplicate entries, companies can maintain a streamlined database and avoid inconsistencies that can disrupt operations.
- Real-time data monitoring: Implementing real-time monitoring systems ensures incoming data is instantly validated and corrected. Businesses prevent inaccuracies from accumulating over time by addressing errors as data enters the system.
- Data enrichment: Enriching existing data with third-party sources enhances the accuracy and depth of customer profiles. This approach allows businesses to gain more actionable insights and improve targeting efforts.
- Data recovery plan: Maintaining a robust data recovery plan is critical for organizations that may experience data corruption or loss. By regularly backing up cleansed data, businesses can recover accurate information during system failures or data breaches, avoiding reintroducing old or corrupted data.
The Long-Term Payoff — Why Clean Data Matters
How Clean Data Drives Real Business Impact
Investing in data hygiene goes far beyond fixing short-term inefficiencies, it lays the foundation for long-term sustainable advantages. Clean, well-maintained data profoundly impacts a business’s operations, customer relations, and financial health.
Improved Customer Experience
With clean, accurate data, companies can deliver more personalized and relevant customer experiences. Up-to-date customer records allow businesses to craft targeted messaging and tailored offers that resonate with individual customers, leading to higher engagement and loyalty. Accurate customer insights also reduce friction in customer interactions, whether through faster service or better-targeted marketing campaigns.
Impact: Personalized experiences increase satisfaction and retention rates, helping businesses stay competitive in increasingly customer-centric markets.
Better Decision-Making
Reliable, clean data empowers decision-makers to confidently rely on insights for strategic planning. In contrast, even minor errors in dirty data can misguide business strategies, resulting in poor investments or missed opportunities. Clean data ensures that business intelligence tools provide accurate predictions, helping companies respond more effectively to market changes and customer needs.
Impact: Businesses with clean data can reduce the risk of costly mistakes, improve forecast accuracy, and optimize resource allocation for better long-term results.
Cost Efficiency
Maintaining clean data eliminates inefficiencies caused by inaccurate records, helping businesses cut down on unnecessary expenses. Dirty data leads to waste — whether it’s from misdirected marketing campaigns or duplicated operational tasks. Clean data reduces the likelihood of these errors, streamlining operations and optimizing resource use across departments.
Impact: Companies that maintain high-quality data spend less on corrective measures, such as redoing marketing campaigns or resolving customer complaints, and more on initiatives that drive growth and profitability.
Scalability and Growth
Clean data creates a strong foundation for business growth. As companies expand and integrate new technologies, maintaining data quality ensures their systems can scale without introducing errors or inefficiencies. Clean data also facilitates smoother integration of advanced technologies like artificial intelligence (AI) and machine learning (ML), which rely on accurate data to generate meaningful insights.
Impact: High-quality data allows businesses to scale efficiently and leverage technology for smarter, faster decision-making, driving future growth.
The Future of Data Hygiene and Business Efficiency
As technology evolves, the future of data hygiene is set to benefit from advanced tools and methodologies, especially those that integrate artificial intelligence (AI) and machine learning (ML). These innovations promise to reshape how businesses manage their data, enhancing accuracy, efficiency, and cost savings.
- AI and machine learning for automated cleansing: AI and ML are increasingly used to automate the data cleansing process. These technologies can identify patterns, spot anomalies, and even predict which data points will likely become obsolete. By continuously learning from data, AI-driven systems can make intelligent suggestions for data correction without manual intervention, significantly reducing human error.
- Real-time data validation and correction: Businesses can implement real-time data validation with AI. Machine learning algorithms scan incoming data for inconsistencies, flagging and correcting errors as they arise. This real-time feedback loop ensures that new data is accurate and helps maintain existing datasets by automatically updating or merging records.
- Cost efficiency through automation: Automating data cleansing reduces employees’ time on repetitive tasks, translating into significant labor cost savings. AI-powered tools allow businesses to scale their data management efforts without expanding their workforce, making it a cost-effective solution, especially for companies managing large datasets.
- Enhanced accuracy and decision-making: AI algorithms improve accuracy by identifying deeper insights and detecting trends in data quality that might go unnoticed through manual processes. This boosts the reliability of business decisions and reduces the risks associated with flawed or outdated data, further driving efficiency and revenue growth.
By leveraging AI and machine learning in data hygiene, businesses can unlock new opportunities for cost savings while enhancing the quality of their decision-making processes. The future of data cleansing lies in intelligent automation, where technology simplifies data management while actively improving it.
Take Charge of Your Data Today
Addressing the issue of dirty data is not just a short-term task but an ongoing effort that offers substantial long-term benefits. Clean, reliable data improves decision-making, elevates customer experiences, and boosts operational efficiency. Whether starting with a simple data audit or embracing advanced technologies like AI for data cleansing, the steps businesses take today will pave the way for sustained success.
By proactively investing in data quality, businesses position themselves to thrive in a competitive, data-driven environment. It’s time to prioritize clean data to build stronger customer relationships, make better decisions, and enhance overall efficiency. Invest in clean data now and set the stage for a more agile and data-driven future.