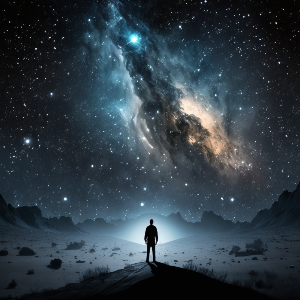
I’ve been deeply involved in implementing data lineage using several technologies throughout my career, but things are different now.
One practical difference is that now I do advisory work for data leaders. They expect input on their priorities and strategy, so I need reasoned opinions based on value, opportunity cost, and organizational maturity. This means I concentrate more on the strategic picture while not losing touch with what can be accomplished tactically.
The other difference is —wait for it — AI. There, I said it. Sorry. I know we are all overrun and getting a bit sick of hearing that AI is the thing that changes everything. I feel a bit guilty giving you one more thing to consider regarding AI, but I will not apologize because I am convinced it is a unique perspective and one that you need to consider thoughtfully.
Standard Lineage Value Propositions
The standard value propositions for investing in data lineage have not changed much.
- Regulatory Compliance: The value is proving via audits that data management controls are in place to mitigate operational risks.
- Operational Efficiency: The value is related to the speed with which the root cause of system issues can be identified and resolved.
- Development Efficiency: The value is related to understanding the scope and level of effort involved in changing or implementing new applications/integrations. Often, this is referred to as impact analysis.
Although the emphasis on each has shifted due to legal changes and the evolution of technology, the conventional wisdom is that these remain the key value drivers.
Are these value drivers important enough and impactful enough for a data leader to allocate more of their budget to data lineage than they do already? The key to the question is my assumption that data leaders are already spending per standards, norms, and benchmarks for their industry, organization size, and the complexity of their data landscape. Naturally, if a data leader is underinvesting, they have exposure and should raise the investment.
The real question is, is data lineage rising in strategic importance and providing a new value proposition that deserves additional funding and emphasis?
Is the Answer Using Lineage to Govern AI?
The conventional wisdom is that AI needs to be governed, and a significant element of that is understanding the data it is trained on and produces. I agree 100%. Data lineage must play an essential role in the governing of AI.
Of course, there are many nuances specific to AI, such as bias, intellectual property infringement, accuracy, etc., but is using lineage to help govern AI any different from using it to govern any other application or technology that consumes and produces data?
The simple answer is no. Well-implemented governance policies, procedures, and controls should apply to all data uses, and AI is the newest and very exciting. The value drivers are still compliance, operational, and development efficiency.
Let’s Pivot Our Orientation
The promise of AI for the enterprise is to usher in unprecedented levels of efficiency and automation that result from an intelligent set of actors (currently called agents).
As an aside, all reputable measures of labor productivity, including the U.S. Bureau of Labor Statistics, show productivity increasing dramatically up and to the right since they started measuring it in the 1950’s. AI is another enabler for that trend to continue but at an exponential rate.
How exactly will AI be able to provide this efficiency and productivity? Doesn’t AI need to be trained? Doesn’t that training need to provide enterprise context and meaning? Where is that going to come from? You can see where I am going with this.
Data lineage is, at its essence, a representation of an enterprise’s data assets, relationships, and contextual knowledge that further describes terminology, processes, metrics, policies, roles, etc. Done well, lineage is the enterprise “data twin” and can be the ‘yin’ to AI’s ‘yang,’ providing all the necessary training context.
Lineage-Driven AI Intelligence
The possibilities are staggering if we allow ourselves to imagine the power of AI driven by accurate data lineage. Here are just a few:
- It could compare industry benchmarks to business process efficiency and recommend specific cost optimization changes.
- It could suggest approaches for optimizing sales based on existing data and identifying gaps.
- It could anticipate data and reporting issues, notify, and possibly proactively implement solutions.
- It could monitor and enforce data usage and security policies, identify the need for new policies, and draft them for human review.
- It could serve as the chief assistant to decision-makers, suggesting issues that require their attention, providing them with analysis, and summarizing options.
The point is that training AI on the enterprise-specific data landscape and all its associated business context is one of the foundational elements needed to transform AI from a personal productivity aid into a strategic tool for business optimization.
What is the New Value Proposition for Lineage?
If you accept that data lineage should be used for training AI, then as leaders, we must ask the question of value to understand whether it is worth investing in.
So many are overhyping AI. The truth is that the value an enterprise receives from implementing AI is currently unclear. While there is massive potential, the current generation of AI is better at improving workers’ productivity than optimizing business processes, making decisions, or carrying out intelligent tasks.
I sense growing frustration. It feels like we are fast approaching the end of what Gartner calls the “hype cycle” and entering the “trough of despair.” Yet, I see some green shoots of experimentation, with some lineage vendors working on marrying their technology with LLMs.
I also see some forward-leaning enterprises ahead of the vendors, using standard APIs to extract lineage and other metadata assets for LLM training, fine-tuning, and retrieval-augmented generation (RAG) patterned deployments.
Can we point to concrete value yet? I haven’t seen it, but I firmly believe it will happen. Enterprises will power AI by training it using an accurate understanding of the data landscape based on its lineage.
Practical Next Steps
Data lineage is growing in importance and will become more strategic. However, I also understand that very few leaders have the luxury of having teams work on experimental projects without a near-term value that impacts the business.
Here is what I suggest you do now to prepare your team and be positioned for the future:
- Place data lineage high on your trend tracking list and review the latest from industry analysts like Gartner at least once every six months.
- At least once a year, engage with what you think is a leading lineage provider to see their demo and roadmap.
- Ensure your current investment in data lineage meets a reasonable standard that matches or exceeds your peers.
- Establish and lead an internal working group of enterprise architects, data architects, and AI experts. Task them with conducting research and reporting to the group.
- To gain internal experience, ensure that any AI pilot projects include some aspect of metadata and lineage-based data relationships LLM training.
- Perform limited experiments with extracting lineage metadata from your existing tools and feeding it to an LLM to start forming a view of current capabilities, limitations, and priorities.