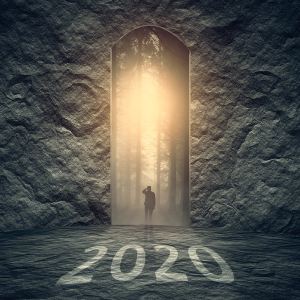
Data is commonly referred to as the new oil, a resource so immensely powerful that its true potential is yet to be discovered. We haven’t achieved enough with data research and other statistical modeling techniques to be able to see data for what it truly is and even our methods of accruing data are rudimentary and rough. People involved in data, or interested in taking advantage of its uses (read as most companies across the world), are always looking ahead to future developments in the discovery, the collection, the storage, and the usages of data. One area very much worth looking ahead in is data architecture, the field in which the structure of a data model is decided and related back to the type of data available. Things are always evolving in this field and, as we head into the new year, there’s lots for us to look at in this coming year. So, let’s get started.
Explainable AI
The use of AI in relation to all elements of the data process is a given at this stage but it isn’t without its problems. Specifically, at the present point in time, there is a trend towards overly complex and opaque implementations of AI for data. This is liable to being ignored if it wasn’t for the fact that governing bodies are now becoming stricter and stricter on data use and privacy issues that always need to be addressed. In this instance, explainable AI is a way to use AI where it transparent with exactly what it is doing with data at all points and easily graspable for those who want to know.
Augmented Data Practice
As we get more and more efficient at collecting data and uncovering where valuable data may be found, we’re also building pressure on ourselves to be able to deal with all of the data. “As we flood ourselves with data, there has been a real struggle in regard to handling the data and uncovering the best way to analyze and manage at great speed. This is where augmented practices, for analysis and management, will flourish, greatly increasing automated speeds and increasing the real time impact of data”, explains Sarah Grose, data analyst at BritStudent and WriteMyx. This development can really increase how useful data is and how quickly we are able to use it.
Introduction Of Data Fabric
Data fabric is a trend that can work alongside the augmentation ideas discussed above. Data fabric relates to data housing, an area which has recently begun to evolve once people began to question the benefit of housing all data together in a single ‘warehouse’. Data fabric is an architectural solution that changes the structure of data housing so that data can be more efficiently used and understood at its different levels. It will boost efficiency and it makes a strong case for the idea that data is definitely an area where real reform to seemingly ‘founding principles’ is not just allowed but ought to be actively encouraged all the time.
Graph Processing
This is a data exploration technique that is set to make a big splash in the coming years and will greatly increase the comprehension capacities of data handlers. “Graph processing and databases are a bridge between human understandings of logical concepts, relationships, transactions, organizations and more with the raw data on the screen. It’s a way of dealing with data in a human-friendly manner, not only an AI-friendly manner”, explains Howard Ash, tech blogger at 1Day2Write and NextCoursework. This will be hugely beneficial moving forward as the use of data becomes more popularized.
Continuous AI
This is a model for looking at receiving and storing data as an ongoing project for a piece of AI, not a one stop job. The benefits of this are obvious and, though it is difficult, a lot can be achieved with not all that much effort if it can be cracked.
Conclusion
Data is a rapidly shifting world in which elements to all sorts of stages in the data process morph and evolve constantly. It’s exciting, but it also takes a lot to stay ahead of, so don’t fall behind!