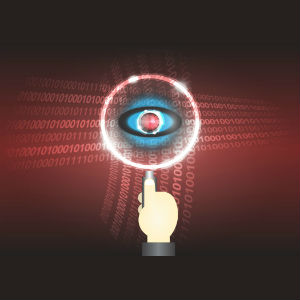
The world is a-buzz with articles about ChatGPT and other Large Learning Models such as Google’s Bard. The tone and tenor of these articles range from blind hype to abject horror. Some of the hype sounds like a bad paraphrase of Dire Straits:
“Look at them losers, that’s the way you do it. Running your business on ChatGPT.”
A few weeks ago, I even succumbed to the temptation and wrote a blog post about it for my company. That post quite literally broke our internet as our hosting provider had to redirect traffic away from it to a Google cache because there was such a sudden spike in traffic to the site.
My perspective: These tools are, quite simply, just tools. As such, they are fraught with the same potential weaknesses as other data management technologies. They have the potential to make aspects of our daily work lives simpler, taking away the drudge tasks that we all have performed in organisations or on projects since the early days of our careers. But, as they are at heart a data management process that ingests source data (training data, prompts) and produces an output.
The pragmatic reality is that these tools will become a part of our daily working lives in years to come, just as email, texting, and relational databases have become part of our day to day grind.
And at this point, I have to invoke O Brien’s Third Law of Process Automation (the ‘suitable for work’ version).
“Automating a badly understood process badly can result in bad things happening faster than you can possibly keep up with them.”
Remember: “Garbage In, Garbage Out” remains one of the fundamental (data quality) laws of the universe.
Stochastic Parrots
At their simplest, LLMs ingest and are trained on large bodies of text (ChatGPT was trained on the entire internet up to 2021) and they learn to guess what the most likely next word should be in a sentence. The key words in a prompt are (and this is a gross oversimplification) used to infer what an expected outcome or output would be, based on the model that has been built from the training data that the model has indexed.
So, there are three potential areas where garbage can enter the system.
- The underlying training data can, and will, encode social and societal biases based on how often a theme, topic, or cluster of keywords appeared together in a string in the source texts. And because it can be expensive to retrain or update models with new source inputs, the underlying model can be slow to properly represent or reflect social or societal changes. The best it can do is to guess what.
- The prompt used to trigger the LLM’s processing can encode biases of the user or, based on how they are phrased, introduce a weighting into the generation of the responses produced by the LLM.
- How we react to and interpret the output of the LLM to our prompt may in turn be influenced by our own cognitive biases. After all, confirmation bias is a key issue in data analytics and data-driven decision making where we are more likely to accept data or statements that match our own views or opinions on a topic.
Finally, another potential source of Garbage In can arise where the LLM has been trained using RLHF (reinforcement learning from human feedback) to rank and fine tune the generated text outputs from the model. There is a potential for the feedback model to inherit biases from reviewers. This bias can arise from their background (social/cultural), education, motivation and incentivisation, or their understanding of the subject matter that is being presented back in the LLM’s output.
Shifting Costs
The promise of tools such as ChatGPT is that they will help reduce cost and save time by helping us to research and write. And this is undeniably true. However, we need to learn the lessons of the past and step back for a moment from the technology and consider what the overall ‘total cost of ownership’ when we introduce an LLM into our organisation’s processes might be.
Environmental Costs
Environmental costs cannot be ignored. Whether it is the energy consumption of training the LLM or the energy consumed when processing a prompt, this all adds up. Training ChatGPT is estimated to have used over 1200 MWh of electricity. This amounts to the total annual energy consumption of almost 300 Irish households. At a time when climate change risks are beginning to manifest, this is not an issue we can ignore. One estimate puts the daily carbon footprint of running ChatGPT at over 24 tonnes of CO2 emitted per day, or almost 9000 tonnes of CO2 per year.
Knowledge Costs
My blog post looked at the potential for loss of learning and knowledge in organisations and society, particularly if low quality and inaccurate LLM outputs are published online and become part of the training data corpus for LLMs. I highlighted two issues:
- Garbage in, Garbage Out applies to training data. And if low quality/inaccurate outputs become the inputs to the next iteration of LLMs, then newer models will compound the errors because they will repeat the error and use it as the basis for predicting the text they should output. This means we need to ensure that we have rigorous quality control checks and accuracy checks applied to the outputs before they are published or used in a ‘production’ context. This will require expertise on the part of organisations and people in organisations to be able to identify errors in outputs and validate them before putting them to use. It will also require expertise to understand how to ask better questions and provide better prompts to the magic box so we can get answers we can rely on.
- Development of knowledge and expertise has, historically, involved people learning by doing and making mistakes as they produce outputs or deliver projects. The introduction of a tool that can find answers to questions quickly (as opposed to helping you find links to sources of potential answers, which is what a search engine does) means that we risk undermining that element of the human learning process in the work place. This is particularly the case in organisations that are working in a remote work/connected work setup where the traditional ‘learn by watching’ or mentorship approaches of the office-based work environment. At the moment, we have a generation or two of people in organisations who can look at a ChatGPT output and identify if the response provided is articulate bullshit or not, and who have the expertise and experience to pose well structured and unbiased prompts to the magic box so they can get better quality responses. What happens in the next two generations of recruits into our organisations when they have not had the opportunity necessarily to ‘learn by doing’ that we have historically taken for granted?
Social and Ethical Costs
There are also social and ethical costs to systems like ChatGPT that we need to consider. At a minimum, we should be concerned about the use of low-cost labour to perform the RLHF tasks of tagging and scoring the outputs of the model during training. OpenAI reportedly paid Kenyan workers less than $2 per hour to curb ChatGPT’s less acceptable and offensive outputs.
This issue of outsourcing the unsexy human effort required to make data processing systems work magically is not new. But as we develop more powerful capabilities to process data, it is important to remember that, just as Cloud is “Someone Else’s Computers”, AI is often “Some other Human’s intelligence”. And often, those other humans are working in low-paid and high stress conditions.
Equally, the challenge for LLMs that are trained on historic texts and produce new outputs based on predicting what will come next will lead to issues of actual or suspected plagiarism. Academia is already concerned about this issue, but in the broader context, the risk of replicating someone else’s content in a commercial context cannot be ignored either. Students of modern music history need look no further than the various cases that have arisen over the years when a songwriter has created a melody that, consciously or not, mimics or copies a melody from another song. One of the most famous of these incidents relates to George Harrison’s My Sweet Lord.
Paying the Piper
The genie is out of the bottle with LLMs like ChatGPT. What we need to do now as data management professionals is realise that the piper who is playing for us now will need to be paid. In previous iterations and evolutions of data processing technologies, we have incurred technical and data debt that we have rolled over again and again over the years.
Hopefully with the potential benefits of tools like ChatGPT and similar LLMs, we will learn the lessons of the fundamentals of good quality data and good quality data management so we can deliver good quality outcomes to businesses and society without incurring costs now that future generations will need to pick up the tab for in our organizations, our societies, and our environment.