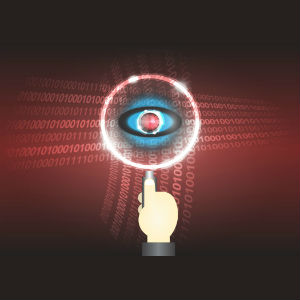
Regular readers of this column will have noticed a thread weaving its way through the articles over the past few months. The role of the individual in the execution of ethical data management and in addressing the risks that arise when we collect data, store data, share data, or put data to work is often overlooked in the face of the rising tidal wave of “ShinyTech3.0.” In many cases, it is the fundamentals of data and people’s interaction with it that are the cause of, and potentially the cure of, many of our data management challenges. As my friend and mentor John Ladley puts it: “Data is anthropological.”
“Recital 4” of the EU’s “General Data Protection Regulation” states that “the processing of personal data should be designed to serve mankind.” This is very much in the Deontological ethical mould, expressing a clear ethical principle that people should be seen as an end in and of themselves, not merely a means to an end. Philosophy majors, or readers of the book “Data Ethics” that I wrote with my colleague Katherine O’Keefe, will recognize this as Kant’s Second Formulation of the Categorical Imperative.
We can take this further of course: the processing of data should be designed to serve mankind. After all, why do we record data? Why is data the “24 by 7 recording of human existence,” as John Ladley has put it in recent conference presentations? Why does the oldest recorded text contain things like letters of complaint about the quality of copper?
This, of course, leads us to another question: How are we doing as a profession ensuring that the processing of data is designed to serve mankind? The troubling answer is that, at best, the jury is still out, for a variety of reasons. But there remain plenty of opportunities for us to tilt the scale in favor of humanity.
The Environmental Impact of Data Debt and Digital Waste
Several years ago, I did a “back of an envelope” study for a retail sector client to assess the environmental benefits of improving their compliance with GDPR and the EU’s laws around electronic direct marketing. The reason: The Data Protection team were in competition with the ESG team for budget and ESG sounded sexier. So, we looked at the volumes of bulk or automated emails they were sending and receiving in just one part of the organisation.
We used information published by Mike Berners-Lee (brother of famous web developer Sir Tim Berners-Lee) as the basis of this rough calculation. We looked at the inbound emails that were unopened (and therefore not actioned) and the outbound emails that bounced (and therefore not actioned). The study looked at just one process in the client’s organization. And the figures were thought-provoking. Just that one process was contributing the equivalent of several hundred kilograms of CO2 each year. That triggered a desire to change practices in process automation and email marketing to reduce the number of waste emails being generated. Not a huge win, but it highlighted the role of data management, data quality management, and data process design in the organization’s overall ESG agenda, while at the same time showing how compliance initiatives had second-order effects in the organization.
It was interesting to learn that the “back of an envelope” analysis that I carried out several years ago has a grown-up cousin, in the form of a research group at the University of Loughborough in the United Kingdom. These researchers have coined the phrase “Digital Decarbonisation” as part of their findings, which highlight starkly how we need to address fundamental issues in our approach to data management and recognize explicitly that the evolution of technologies in this area are not without environmental impacts.
Even the headline statistics they cite give pause for thought. Sixty-five percent of data is never used, and 15% is out of date. The data industry is forecast to account for more GHG emissions than the automotive and air travel sectors combined. The average worker generates 22 tons of CO2 yearly from their digital footprint.
Understanding Why Data Is Not Carbon-Neutral
Digging into the research by Tom Jackson and Ian Hodgkinson, we can find an obvious explanation for the growth in “dark data” and the environmental risks posed by what they term “single-use knowledge.”
In this context, they adopt Gartner’s definition of “dark data” as “the information assets organizations collect, process and store during regular business activities, but generally fail to use for other purposes (for example, analytics, business relationships and direct monetizing).” They define “single-use knowledge” as knowledge that is generated or acquired by a knowledge-worker, but which is not shared or socialised in the organisation due to time or other constraints. As such, the knowledge is “throw-away” and the associated data is “dark data” which the rest of the organisation may not know exists. Alternatively, even if the data is socialised it can become lost or forgotten due to staff turnover or systems changes. It might still exist as “dark data,” but it is not adding value.
This should all sound depressingly familiar to those of us working in data governance, document and records management, master data management, data quality management, or data warehousing.
Jackson and Hodgkinson are very blunt in their assessment of the environmental risks posed by ‘single-use knowledge’ and dark data:
“While single-use knowledge may be deemed a major step forward, as it enables decisionmakers to operate quickly and provide prompt answers, it can lead to surface learning and an over-reliance on technology, creating significant volumes of dark data. A critical consequence of this is that employees and decision-makers within organizations will repeatedly search and store the same digital data over time, with data being duplicated and generating huge drains on power and energy. As we move further into the single-use knowledge mindset, organizations may become even more reliant on information resources with a ‘single-use’ approach.”
In many respects, the outputs of an LLM could arguably represent the current pinnacle of single-use knowledge, with drains on power and energy in respect of the storage and processing of the underlying data as well as the power and energy costs of the model training and the execution of the model in response to prompts, for a response that may not be accurate in response to a prompt at a point in time in a process.
It behoves us as professionals and inhabitants of the planet to ensure that as we adopt and apply new technologies to manage our burgeoning data mountains that we don’t make the social, societal, and environmental problems worse.
Pioneering organizations that are adopting sustainable digital and data strategies will most likely be doing fundamental data management activities better to reduce dark data, reduce single-use knowledge, and generally reduce waste in how our organizations process data. From data modeling to data warehousing, every discipline in the DAMA DMBoK arguably has a role to play in reducing the energy and environmental interest rate that is being charged on the data debt in our organizations. While much has been written recently about the carbon footprint of large language models, we need to accept that even the humble database query or the unglamorous email comes with carbon price tags attached.
We need to recognize that unglamorous data management tasks like the development and understanding of data models (conceptual, logical, and physical) and business data glossaries have a key role to play in helping organizations improve their data carbon footprint. By having a better understanding of what data means (glossary) and how data concepts relate to each other in the organization’s data model and having a better understanding of how to navigate that data model efficiently, knowledge workers can write more efficient queries to support business reporting.
This, of course, means improving the training for knowledge workers so they have better skills in developing query and report designs that are efficient and reusable. LLMs may have a role to play here to support knowledge-workers in optimizing queries, but the human needs to have the knowledge and competence to be able to work with the tool as a tool and to be able to assess any suggested outputs for sanity and effectiveness. A fool with a tool is still a fool, they’re just a fool who can make mistakes faster.
The fundamentals of metadata management and document and content management are likely where there is the highest likelihood of high impact early wins for most organizations. Improving the searchability and findability of content, improving the storage of critical records and information (such as getting important records out of email inboxes and into proper filing systems), and culling obsolete or duplicate content and data will reduce storage and reduce the time taken to search.
Again, AI and machine learning have a potential role to play here as well as helping apply the necessary tagging and categorizations to content. Robotic process automation can be used to create rules to move data and records from email to a proper filing destination. And automated rules can be applied to the retention, disposition, or deletion of data.
This isn’t rocket science. It’s basic data governance and data management for structured and unstructured data. But it can have a bottom-line financial impact (lower energy costs) as well as contribute to ESG and environmental protection goals. But it starts with engaging the human, educating the human, and ensuring that organizations are considering how the good practices we have been promoting as a profession have a role to play in tackling the environmental impacts of data.
And, for eagle-eyed readers, you will see how this maps back to my theme in an earlier column of how we need to apply data management disciplines as the techne to bridge the episteme of ethical principles and the phronesis of actually putting those principles into practice. If our organisations are serious about reducing their carbon footprints, they cannot do that while continuing to ignore the elephant in the room that is the carbon impact of the most critical business asset: data!
What Can Data Professionals Do?
Data must be processed to serve mankind. Right now, that doesn’t seem to be happening as our industry and our stakeholders seem to be enthralled with yet another “ShinyTech3.0,” which brings with it a massive data debt price tag and a potential environmental impact interest rate on that debt. We need to be asking ourselves and our C-Suite critical questions such as “How precisely will introducing an LLM into our process reduce our net energy consumption?”
Jackson and Hodgkinson are clear. We need to reduce “dark data” and reduce the prevalence of “single-use knowledge.” This means addressing some of the fundamentals of how we design data processing activities to remove waste and improve efficiency. It does not mean layering a Large Language Model on top of poor quality and poorly curated data and hoping for a miracle.
According to their research:
“The key to digital decarbonization may, therefore, lie in how knowledge and data are used, and reused, by organizations and their employees in daily activities and operations.”
In addition to the environmental cost, the human productivity cost of our technical and data debt needs to be recognized. While each iteration of technology promises step-changes in efficiencies, to date, we seem to have merely succeeded in moving the inefficiencies to other stages in processes or other parts of our organizations.
We seem to have overlooked the quality systems mantras of muda (wastefulness) and muri (excessive burdens). We have created data debt and a growing mountain of dark data that needs to be addressed. New technologies can help, but we need to ensure that there is a recognition of the role of humans in the process with respect to how we got here and what we need to do to address and remedy the situation. We also need to recognize that, if we do not act, we will increasingly be feeding our new technologies a junk food diet of redundant, obsolete, and poor-quality data.
In Ireland for the past few years, we have had a mantra around packaging waste in food and consumer goods: Reduce, Reuse, Recycle. We are running out of time to apply this mantra to our data world.
My call to action: Pick ONE THING you and your teams can do today to reduce your dark data and apply good data management principles to reducing single-use knowledge. It might be as simple as cleaning house in your inbox.