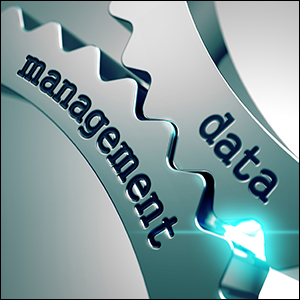
Data governance is considered an oversight activity for work pertaining to the creation and management of data. That is, what are the policies, procedures, roles, and accountability around data assets.
Data management supports the development and maintenance of architectures, best practices, and procedures that manage the data life cycle of an organization. Data governance does not, however, identify how to do the activity within the work of data management.
One of the reasons data governance efforts fail is that they do not integrate with the other organizational data management functions. They do not move from stating what they will do to actually doing the data governance activities that provide value, organizational clarity, and standardization. Integrating data governance activities into project work reinforces the purpose of the data governance organization.
It is within data management activities that data architecture artifacts such as data models and data integration models are developed and maintained. In most organizations, 85% of their metadata is captured and developed through the Data Modeling process. Yet, none of that metadata is being governed. Organizations tend to treat data modeling as a project deliverable to be tucked away after the project is finished.
Capturing metadata information at creation avoids having to rediscover it later. By applying some formality to the execution of the modeling work that you’re already doing, you can increase quality and productivity. What is needed is the integration of data governance policies, processes, and standards within data management functions for data modeling activity.
The Data Modeling Center of Excellence
Well integrated data governance stresses that the governance processes must be integrated into project activities and ongoing data management tasks as part of a regular routine. Setting up a Data Modeling Center of Excellence (DMCoE) provides the clarity and purpose needed to establish the process and quality control checks needed in integrate data governance into project work. The DMCoE is a body within an organization that has data modeling knowledge and competency. It is comprised of individuals who disseminate modeling knowledge and share best practices. The DMCoE goal is to ensure that each of the relevant data governance functions is integrated into the data modeling design and development activities.
The principles that provide the clarity and purpose must also align with the data governance objectives, business policies, strategies, goals, and other things that motivate the business. Below is a sample listing of a few data principles that support the mission of the DMCoE
- Data is a valued asset
- Data is managed
- Data is fit for purpose
- Data is standardized
- Data is reused
Global vs Local Data Governance
Big Picture Governance
The big picture view of data governance is enterprise wide. An organization may already have a large data governance initiative underway. Part of any big data governance program plan is the identification of what needs to be governed and how the governance is applied to the management of the data assets. The DMCoE is the integration point where what is governed meets its ‘how to’ processes. The DMCoE could be assimilated into a much larger governance effort.
Small Picture Governance
In a small picture view of the DMCoE, key data governance activities such as business definition validation, naming standards enforcement, and metadata management become a part of a localized data governance program, rather than being a standalone effort. This approach is not a high-profile initiative, so it is not as involved as the enterprise wide data governance program. It becomes the starting point for formalizing data modeling activities that already exist. It could be thought of as a starter governance program. Once the success criteria have been established and measured, it can be identified as an example of what a larger effort might be able to attain.
An Example
This is a basic example of the workflow for how the integration might be achieved. The data modeler creates the project’s entities, attributes, and relationship business and technical definitions. Upon completion, the data model would then be reviewed to ensure adherence to naming and design standards.
For new data objects that have not undergone a validation process, the reviewer would reconcile those data artifacts with the guidelines for a standard reusable data object while the project continues its data modeling activity. This moves the data governance function from a reactive project inhibitor to a proactive agile engagement.
In this approach, the business works directly with the DMCoE to assist and ensure that the entities, attributes, and relationships are defined and that they are consistent with agreed-upon corporate definitions and naming standards. A validated data object is now a reusable component for other data modeling projects which accelerates project completion because the data objects have already undergone a governance process.
Measuring Success
At some point the question will arise: ‘Is this effort really worth the time invested?’ The DMCoe needs to measure its effectiveness in order to determine if the goals are being met. Success is measured by the effectiveness of the people, the processes and the technology. Process is the definition of how work gets done. That work may be executed by people or technology, so metrics can be broken down into people process effectiveness and technology process effectiveness.
Process Metrics
Quantitative and qualitative processes ensure that data meets precise standards and business definitions. Below are a few of the metrics that could be applied to people or roles governing data assets.
- Number of approved and implemented data standards
- Number of processes aligned with data governance objectives
- Number of data objects approved for reuse
- Data model quality review checklist scorecard
Technology Metrics
It stands to reason that because metadata is created via data modeling tools, the DMCoE should be able to leverage those tools to help manage the integrity and consistency of metadata. Below are a few measures that can be identified to show how technology improves the progress towards the DMCoE goals.
- Number of business terms associated with data models and data objects
- Percentage of lineage across data objects
- Percentage of validated data objects reused in new projects
Summary
Data modeling creates the metadata that provides context to raw data; it is the business and technical rules that provide data element meaning. Data modeling projects include everything from transaction processing and master data management (MDM) consolidation, to BI aggregations. Each modeling effort creates metadata that should be captured and managed within an enterprise point of view. The DMCoE is the convergence of data governance with data modeling.