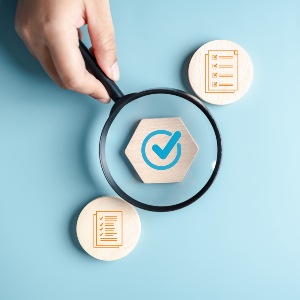
Data empowers businesses to gain valuable insights into industry trends and fosters profitable decision-making for long-term growth. It enables firms to reduce expenses and acquire and retain customers, thereby gaining a competitive edge in the digital ecosystem. No wonder businesses of all sizes are switching to data-driven culture from conventional practices. According to reports, worldwide data volume will reach 175 zettabytes by 2025, unlocking massive business growth opportunities. However, as the volume of data continues to surge, ensuring its accuracy and reliability becomes crucial and challenging. That’s where data quality and observability can prove to be a game-changer. Both share the common goal of ensuring data’s reliability, trustworthiness, and value to foster accurate decision-making in organizations.
This article covers the concepts of data observability vs. data quality, their key roles and benefits, differences and similarities, and how they can improve data usefulness.
What Is Data Quality?
Data quality measures the appropriateness of data to serve a specific purpose. It comprises several best practices, such as data profiling, cleansing, and more, to ensure it meets specific standards for accuracy, consistency, completeness, and validity.
Its main goal is to foster accurate decision-making for organizations by maintaining data health.
Poor quality data, including inaccurate, untimely, incomplete, and duplicative, can cost firms significant financial losses. In fact, a survey by Gartner stated that poor data quality costs businesses an average of $15 million annually.
On the other hand, high-quality data enables businesses to meet customer needs and expectations, deliver better services, and drive effective strategies to achieve positive ROI. Besides, it helps them maintain regulatory compliance and reduce legal risks.
No wonder firms are striving to collect high-quality data.
Key Dimensions to Measuring Data Quality
Businesses can measure data quality along the dimensions shared below:
- Accuracy: The data set should be free from inconsistencies and errors.
- Completeness: The data set should include all vital information.
- Consistency: Data across distinct sources and systems should exhibit uniformity.
- Timeliness: The data should be up-to-date according to recent industry trends.
- Uniqueness: The data should exhibit no duplicity or redundancy.
- Validity: The data should conform to standard formats.
What Is Data Observability?
Data observability is a relatively new concept that collects metadata from various data pipeline stages, including data ingestion, transformation, and consumption.
The purpose is to continuously monitor and understand data flows within organizations to detect and address any issues and anomalies in real-time. This reduces downtime and data-related challenges that negatively impact decision-making.
With data observability, businesses can identify their most vital data sets, users of that data, and challenges arising from it.
Vital Pillars to Measuring Data Observability
Data observability rests on the five pillars shared below:
- Freshness: It depicts the current data’s state and whether it’s up-to-date according to industry parameters and trends.
- Distribution: It reflects whether the data values fall within a suitable range, indicating its trustworthiness.
- Volume: It gauges the completeness of data. Inconsistent data volume depicts underlying issues with data sources.
- Schema: It monitors the changes in data within an organization. For instance, it tracks who updated or changed data, when (day and time), etc.
- Lineage: Documents the entire data flow from initial sources to end-user consumption.
Key Differences and Similarities between Data Observability and Data Quality
Both data quality and data observability ensure data reliability in an organization. They both help foster data-driven decision-making in a firm, strengthening its data management strategy. However, their primary goals and roles are different.
Here are a few crucial differences.
#1: Goals
- Data Quality: The primary goal of data quality is to ensure overall data accuracy and consistency. It’s mainly concerned only with the data.
- Data Observability: Its primary goal is to track the behavior of data in real-time while ensuring the reliability of data delivery systems. It’s concerned with both the data and its delivery system.
#2: Functionality
- Data Quality: It evaluates the accuracy and overall data health in static datasets or data at rest. It focuses on correcting errors.
- Data Observability: It monitors the reliability of data in motion through data pipelines. It focuses on addressing systemic challenges.
#3: Approach for Rule Generation
- Data Quality: It leverages static or fixed rules and metrics.
- Data Observability: It utilizes machine learning to generate adaptive and dynamic rules and metrics.
#4: Issue Resolution Methods
- Data Quality: It uses methods like data cleansing, profiling, and validation to deal with the outcomes of data issues.
- Data Observability: It uses techniques like anomaly detection and statistical analysis to deal with the root causes of data-related issues.
In short, data quality and observability are complementary but distinct aspects of data management.
How Data Observability and Data Quality Can Contribute to Improving Data Usefulness?
Since data quality and observability ensure keeping data’s health intact, organizations can use them to improve data’s usefulness.
With powerful data observability practices, firms can identify anomalies in the data and take measures to keep the data pipelines clean. This can minimize errors in data pipelines, thus improving data quality.
Here’s an example depicting how organizations can leverage both practices to improve data usefulness:
Say a Sitecore to WordPress migration agency comes across a SaaS client with a massive content-rich website that uses Sitecore for content or data management. With time, as the data grows in volume, the company decides to migrate to WordPress, which offers better features and functionalities.
The migration procedure mainly involves moving the entire content database, including blog posts, articles, images, and user data. Any data loss could negatively impact their client’s user experience and SEO rankings. So, the experts should ensure the data remains consistent throughout the process.
Here, deploying data quality checks alone cannot suffice. Combining data quality practices with data observability systems and tools is crucial to ensure a seamless transition.
Data observability tools can help experts monitor the flow of content and generate alerts on detecting anomalies during the data transfer. The timely identification of data-related issues allows experts to take the appropriate measures and minimize downtime. Besides, these tools can automate data quality checks by using advanced machine learning algorithms to create strategic quality monitoring rules based on data sources and patterns.
Data quality and data observability practices thus play a pivotal role in ensuring zero data corruption and seamless transition.
The outcome? The SaaS client can continue delivering quality content without hassles.
Conclusion
While the key goals and functionalities of data observability and data quality are different, they ultimately ensure data integrity and reliability in firms.
Both practices are vital pillars for building a data-driven culture in an organization, as depicted in this post.
Leveraging data quality and data observability can help organizations maintain data’s health, make data-driven decisions, and ensure long-term growth.
Image used under license from Shutterstock