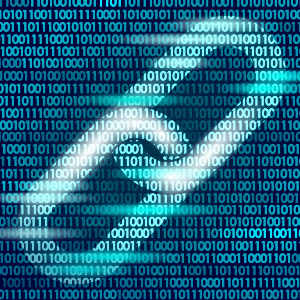
In 2021, there were many new use cases that came to life and became viable “data products” due to focus on data as a commodity. The advent of data democratization and formal enterprise data management maturity driven KPI’s within the companies have resulted in a seat at the board level for chief data officers.
Please join The Data Administration Newsletter (TDAN.com) in welcoming Karan Dhawal and his new quarterly column, Data Value Chain, to our pages.
The fact that companies are becoming data driven is evident with recent data driven products from many companies. Taco Bell recently started a data enabled product for monthly taco subscriptions and Walmart uses big data analytics to improve a customer emotional intelligence quotient. Strategic data leaders are using the opportunity provided by innovations in data eco-systems to use historically siloed data and create new data products by building trust and delivering on transparency promise.
Data driven culture allows for building trust and provided transparency across all decisions. Insights based decisions are becoming a fabric as part of overall culture for many companies and is driven by data health. In this article, we will review the concept of data & information value chains and how this foundational concept allows for faster development of data products.
In Business Management, Value Chains have been used as a decision support tool to model the chain of activities that an organization performs in order to deliver a valuable product or service to the market -Porter 1985. The value chain categorizes the generic value-adding activities of an organization allowing them to be understood and optimized. A value chain is made up of a series of subsystems each with inputs, transformation processes, and outputs.
In words of Rado Kotorov, CEO at Trendalyze, “Taking the leap from data collection and analysis to using data to make a real difference to the business’s bottom line is a challenge for many organizations.” The key is having a ‘Data Value Chain’, which can let companies tap into data as a new revenue stream or to reduce costs. In Specific “Big Data”, Value Chain was defined in 2014 by Edward Curry to model the high-level activities that comprise an information system.
McKinsey further takes this concept and provides example use cases for top line growth or operational excellence for bottom line with a formula for value captured.
Data / Information value chains can be defined in 5 key steps towards value generation: data capture, data refinement, data enrichment, analytics and data monetization also called the “Land of Opportunities”. Data value chain is NOT complete until the data insights have not been used towards monetization either for new data products or risk/cost reduction or operational excellence.
Data subject matter experts, product managers and business strategy leaders to brainstorm and create new go-to market data products and need to spend most of the time in the “Land of Opportunities” data value chain step.
Information and business technology teams tend to spend more time in data raw collection, data integration, data quality fixes and in creation of complex data models for analytics applications and sometime lose sight that value of data is perishable. Big data presents actionable opportunities at the time data is collected and hence needs agile approach towards data strategy enabled data products.
Data Value Chain example here provides some use cases on data monetization with a data value chain reference from Data2x organization.
In the eight foundational end to end process value stream steps of marketing, sales, configure/price/quote, contract management, fulfilment, customer support, billing, revenue recognition and receivable, most of the data needed for business product or service offerings in created and stored. These eight value stream processes usually have many IT and or business owned applications with complex integrations and data models. Primal data objects like customer, product, pricing can have siloed data models due to business needs, applications that create need for complex data transformations during the collection set of data value chains. Transactional data like customer bookings, billing, customer service records may be product or business unit specific and may also require special data marts during the publication step of data value chains.
Uptake and Impact steps of data value chains is where the most value of data exists and can be liberated.
Using technology to connect to users and “multi” processing data for new insights. Promoting data usage culture and encouraging data use for every decision are critical aspects of uptake step of a data value chain.
Impact phase of data value chain relies on identification of data driven policies, tracking tangible behavior changes and reusing the information to enhance marketing and sales efforts allow for enhanced process value stream.
Uses cases, like below, can bring exponential value if implemented with data health and agility.
- If your customer buys a product from store or commerce portal, is your company offering relevant products to improve revenue or enhance customer experience?
- Is your data value chain providing you insights into operational efficiencies (returns, safety, compliance, margin, etc.)?
- Is your data value chain providing you with differentiation in your products / go to market strategy? (agility, net revenue growth etc.)
One new innovative way for agility on data value chain optimization and usage is to label “Useful Data” as “Monetized Data Element” based on foundational data reduction techniques. The MDE label becomes metadata as part of data enablement. For further readings, consider “Monetized Data Element”? These MDE labels can be classified into 4 different types: revenue, cost, quality and business. These monetized data elements can become part of meta data management like critical and sensitive data management practices. If stochastic optimization is your passion, then feel free to check out the article, “Using Monetized Data Elements Towards Stochastic Optimization”.
In summary, influence and connection with customer towards the landing and retention of customers is key to data monetization from the four data value chain steps of collection, publication, uptake and impact. Uses case like customer 360view across all Business Units and Products for bookings and billings are enabled by visualizing data value chains.
Article References
- https://data2x.org/resource-center/data-value-chain-moving-from-production-to-impact/
- Reference Book: The Big Data Value Chain: Definitions, Concepts, and Theoretical Approaches by Edward Curry
- Achieving business impact with data: A comprehensive perspective on the insights value chain by Dr. Niko Mohr and Holger Hürtgen – McKinsey & Company