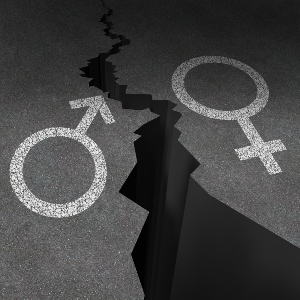
When working with students and educators to address a particular issue, I often have them draw a massive tree. They usually look confused, but always comply. I tell them to jot down the issue in question in the center of the tree, its consequences along the branches, and its causes beneath the soil. We call this the Root Cause Tree and discuss how the foliage at large (the issue at hand) could not exist without first putting down roots. So far, this column has delved into multiple issues related to data’s gender gap.
This month, I’d like to take a step back and look at the origins of the disparity, or its roots. Only by examining where this phenomenon comes from can we begin to understand how to eradicate it. In the introduction to her 2019 book Invisible Women, Caroline Criado Perez writes, “One of the most important things to say about the data gender gap is that it is not necessarily malicious, or even deliberate” (XII). The first step in addressing an issue like this one is to recognize its existence. The second step is to look at its seeds.
In short, data’s gender gap is rooted in the tendency of our algorithms and studies to reflect systemic bias. The issue will continue to appear in the fields surrounding data collection, so long as those who conduct said collection harbor prejudices, conscious or not. It exists in addition to (and is tied to) the tendency of many industries to be male-dominated. A gap in representation leads to a gap in information.
Every person has a personal, intersectional lens through which they approach the world, and our data and information are often most applicable to those who research it. Therefore, having white, heterosexual, cisgender, financially well-off men as a majority in a given group gives us data, and therefore a world, based around seeing that type of person as the norm. Power structures and data go hand in hand: which came first, the chicken or the egg? Our hierarchies reflect our biases, and in turn, our biases reflect the hierarchies and systems we are raised to be familiar with. The fact that we as a society tend to place men in positions of power rather than women must be considered when we are analyzing the road to data’s gender gap.
Take, for example, events within the New York City Department of Education in the past few weeks. Schools were originally set to open for rotating in-person learning on September 10th, but the prospect of a teachers’ union strike forced the opening back to September 21st. Many union members are arguing that this delay is not enough and are sharing the fact that 82% of Department of Education employees are women, but it is largely men (NYC Mayor Bill de Blasio and Schools Chancellor Richard Carranza) who are making decisions regarding reopening.
Why is this a significant issue? Female educators are more likely than their male counterparts to be concerned about finding childcare for their own families if forced to go back to teaching in person while their children are learning remotely. If we poll the Department of Education leadership team, which is 40% male, over whether or not they believe it is possible to have teachers back in the classroom full time, the data gathered will not be representative of a teaching force that is in fact only 18% male. To ensure proper representation, we must take samples of a population that effectively portray the group we are trying to represent. Otherwise, our data gender gap will persist, and many folks will be left out of the equation.
A lack of gender diversity is a cyclical issue in fields such as technology and medicine. With few non-men visible in these industries, those of other genders do not necessarily have relatable role models in the field who they wish to emulate. The lack of representation may make the environment appear hostile, and that hostility in turn perpetuates the lack of representation. Which came first, the chicken or the egg?
Groups of people are not the only factor encouraging this division, however. Carnegie Mellon University (CMU) conducted a study analyzing to whom targeted ads for high-paying ($200,000+ annual salary) and tech-related jobs were directed. They found that for every ad a woman was shown, men were shown six. CMU’s official report on this stated that “To study the impact of gender, researchers used AdFisher to create 1,000 simulated users—half designated male, half female—and had them visit 100 top employment sites.”
When AdFisher was used to reviewed the ads that were shown to the simulated users, the site most strongly associated with the male profiles was a career coaching service for executive positions […]” showing that even when users of different genders had the same online presence and activity, particular positions were still gender-catered. This differentiation between opportunity visibility encourages the gender divide in many industries, including computer science and technology. In order for certain groups to take up certain opportunities, they must know that said opportunities exist.
According to a 2015 VICE article titled When Algorithms are Sexist, “Dr. Louise Selby is a pediatrician. She’s also a woman. According to the computer system at the gym she uses, that’s not possible. Selby told the Cambridge News that she found herself locked out of the women’s changing rooms at PureGym in Cambridge, even though she had an access code. It turned out that she couldn’t enter because she had given her title as “Dr.”—and so was automatically registered as male.”
Misogynistic microaggressions are encoded into the fabric of society, up to and including our experiences at the gym. If our engineers and tech wizards cannot consistently make algorithms or technology that places all genders on equal footing, how can we expect our researchers and doctors to do so? To work toward a more equitable world, we must ensure that we deal with the roadblocks preventing one from the ground up. We must dig the data gender gap tree out of the ground, roots, and all. Tune in next quarter to read about the steps we can take to ensure that our data is fair and just.