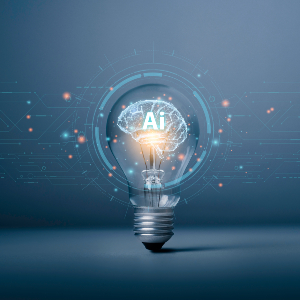
Being an AI-ready organization involves identifying and then overcoming data issues that hinder the effective use of AI and generative AI. These organizations ensure their data is prepared for AI applications including data cleansing, normalization, and data integrity. Collaboration across various teams, including IT, data science, and business units, is essential to produce AI-ready data. Additionally, gaining proper attention and alignment from the CIO office and business leaders is crucial for prioritizing data initiatives and achieving the necessary support for AI readiness efforts. To learn more, I spoke with data professionals at #CIOChat.
Key Data Issues
In organizations that leverage AI or generative AI, two major hurdles often impede progress say CIOs: data quality and data literacy. With data coming from multiple sources of varying reliability and timeliness, maintaining high-quality data is a continuous challenge that starts with education and training. At the same time, the workforce can struggle to keep pace with evolving data standards and definitions, which hampers effective data utilization.
According to Isaac Sacolick, former BusinessWeek CIO, common problems that hinder AI-ready data include:
- Accessibility: Is the data on a platform that allows for no-code integrations with large language models (LLMs) and copilot systems? If not, significant development work is needed.
- Data Cleanliness: Ensuring data quality and maintaining confidentiality is crucial. Dirty or incomplete data requires extensive data cleaning efforts.
- AI Governance: Leadership must clearly define AI governance policies to guide the ethical and effective use of AI technologies.
For enterprises, the most significant data issues (in rough order) include the following, says Dion Hinchcliffe, Research Vice President at Constellation Research:
- Data Ownership and Control
- PII Safety and Privacy
- Data Wrangling and Readiness
- Cybersecurity
- Intellectual Property Retention
- Accuracy and Grounding
- Training Effectiveness
- Governance
One of the biggest challenges, finds in-transition CIO Martin Davis, “is ensuring data quality and trustworthiness. Knowing the provenance of the data — where it came from, its validity, and its source reliability — is critical. Using unverified or poor-quality data can lead to flawed AI outcomes, encapsulated in the adage: “AI + bad data = Bad AI.”
Additionally, staffing the right skills to manage a diverse portfolio of data and AI tasks can be challenging. Consolidation in data management can help IT leaders streamline their hiring, training, and staffing processes, focusing on a narrower and more specialized skill set. This approach not only enhances operational efficiency, but also supports the effective deployment of AI solutions.
Actions to Create AI-Ready Data
Creating AI-ready data involves establishing an AI data-readiness capability, which should be an extension of a Data Management Center of Excellence. This initiative, says Hinchcliffe, requires “new, dedicated team members, a solid technical foundation for data models, and a continuous feedback loop from end-users. Continuous improvement and robust testing strategies for random answer generators (RAGs) and large language models (LLMs) are also essential.”
Fundamental data governance practices are crucial, including clarity of ownership, purpose, and derivation, to ensure data trustworthiness. A broad approach to data governance is necessary, promoting organizational data literacy and fluency. A mature data governance practice will support both the technical and human aspects of data assets. Jim Russell, Manhattanville College CIO, says, “Robust data governance is essential. There needs to be a big-tent approach to managing data standards and improving organizational data literacy and fluency. A mature practice will help both the technical and human side of your data assets.”
Additionally, Sacolick suggests, specific objectives will dictate further actions:
- For customer service, integrating CRM, product information, and other customer 360 data sources is key.
- Knowledge management requires a strong taxonomy to be effective.
- Treating code as data necessitates proper context to maximize its utility.
Teams to Produce AI–Ready Data
Ensuring enterprise data is AI-ready requires the continuous improvement of data management practices, led by data owners and supported by a proactive data governance strategy. This involves not just functional teams responsible for data ingestion, processing, and modeling, but also technical teams that architect, support, and develop with the data. Additionally, security and governance teams play crucial roles, leaving few teams uninvolved in the data vision. Key groups that must be involved as partners in creating AI-ready enterprise data include the following, according to Hinchcliffe:
- Data Owners: Responsible for the stewardship and governance of data.
- Data Consumers: Both internal and external users who utilize the data.
- Data Scientists and AI Practitioners: Experts who analyze and derive insights from the data.
- AI Architects and Model Owners: Professionals who design and maintain AI models.
- AI Operations/Delivery Teams: Ensure the deployment and operational efficiency of AI solutions.
- Data Management/Compliance Teams: Oversee data standards and regulatory compliance.
- Chief Data and Analytics Officer (CDAO) and Chief Information Security Officer (CISO): Provide executive oversight and ensure the security and integrity of data.
Producing AI-ready data is a collaborative effort that requires the involvement of diverse teams across the organization, each contributing their expertise to ensure data quality, security, and usability. Russell adds, “I am not sure any teams that are not involved … The functional teams (data ingestion, processing, etc.) need to be all in on the data vision. Plus, the technical teams that model, architect, support and develop with the data. Add in security and governance and few are left out. Sacolick adds, “AI-ready is about continuous improvement led by data owners and with a proactive data governance strategy.”
Proper Attention
The level of attention from the CIO office and business leaders on making data AI-ready is inconsistent. Russell says, “CIOs often have competing priorities and, despite being generally well-focused, their efforts can be undermined if other business leaders or C-suite executives are not actively participating in data governance. The transformative potential of generative AI (GenAI) might necessitate a radical rethink of how organizations relate to data, akin to the broad awareness cultivated around customer and employee experiences. While the value placed on data experience might not yet be as high, adopting this mindset is essential for leveraging AI effectively.”
Many organizations currently pay attention to AI readiness for the wrong reasons. Often, Davis says, “companies are not adept at data management and governance but still expect AI to produce good results from their existing flawed data, leading to frustration and misplaced blame when AI systems fail.” For enterprises, Hinchcliffe says, “AI readiness is a top CIO priority for 2024 and 2025. Business leaders, too, show significant interest, particularly when useful problems are identified that AI can solve. Achieving the necessary alignment across the organization requires a shared commitment to robust data governance and a collective understanding of the strategic value of AI-ready data.”
Parting Words
Ensuring data is AI-ready requires a robust data capability involving fresh, dedicated team members, solid technical foundations, continuous improvement, and robust testing strategies. Fundamental data governance practices, including clear ownership, purpose, and derivation, are essential to data trustworthiness.
Key stakeholders include data owners, consumers, scientists, AI practitioners, architects, model owners, and compliance teams, all supported by executive oversight from the CDAO and CISO. Effective data governance must promote organizational literacy and be adaptable to specific objectives, such as customer service integration, knowledge management, and context-aware data usage. At the same time, while CIOs prioritize AI readiness, active participation from the entire C-suite is essential. Mismanagement often leads to poor AI outcomes, highlighting the need for a collective focus on data governance and the strategic importance of AI-ready data.