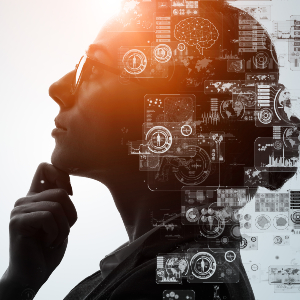
Data is critical for any business as it helps them make decisions based on trends, statistical numbers and facts. Due to this importance of data, data science as a multi-disciplinary field developed. It utilizes scientific approaches, frameworks, algorithms, and procedures to extract insight from a massive amount of data. These days, data science is the backbone of any industry and current trends indicate that it’ll accumulate even more importance in the coming days. If you want your business to succeed, it is critical that you bank on data science. This article offers you a comprehensive insight into data science.
History of Data Science
In 1962, John Tukey in wrote about the convergence of computers and statistics to devise measurable outputs within hours. In 1974, data science was mentioned multiple times by Peter Naur in his review, Concise Survey of Computer Methods.
Data Science: What Is It?
Data science can be understood as a combination of algorithms, tools and the principles of machine learning designed to uncover secret patterns hidden in raw data. The IASC (International Association for Statistical Computing) was formed to connect domain expertise, traditional statistical methodology and modern computer technology to convert data into knowledge.
By 1994, various organizations started collecting tremendous individual data for new showcasing efforts. William S. Cleveland in 2001 put forward an activity plan on how to create a focused understanding and scope of data scientists and highlighted six regions of studies for colleges and offices. In 2003, the Data Science Journal was published by Columbia University to set up a platform for data teams. In 2005, a collection of digital data was published by the National Science Board and in 2013, it was revealed by IBM that 90% of global data was created in the previous two years. By this time, the importance of data science was realized. As of now, data science is in constant demand and is crucial for the success of any business.

Source: edureka.co
As illustrated in the image above, a data analyst generally explains what’s happening by processing the data’s history. As for a data scientist, he performs exploratory analysis and uses numerous advanced algorithms with the intention of predicting future occurrences. A data scientist scrutinizes a given data from various angles including angles that might not have been known earlier. In a nutshell, data science uses machine learning, prescriptive analysis and predictive casual analytics to make decisions and predictions.
Pattern Discovery Through Machine Learning: If you don’t possess the parameters to make predictions, you need to discover hidden patterns with the dataset to make meaningful predictions. This is the unsupervised model as you don’t own any predefined labels for grouping. Clustering is the most common algorithm for discovering patterns.
Making Predictions With Machine Learning: If you own a finance company’s transactional data and you need to construct a model to determine future trends, you must bet on machine learning algorithms. This is an example of supervised learning as you already possess the data through which you can train your machine.
Prescriptive Analytics: Prescriptive analytics is necessary if you want a model capable of making its own decisions and alter it through dynamic parameters. This field mostly concerns with offering advice. It predicts and offers a range of prescribed actions and related outcomes.
Predictive Casual Analytics: Application of predictive casual analytics is necessary if you want a model that can predict the probability of a particular future event.
As illustrated in the image below, data analysis is mainly occupied with descriptive analytics and prediction. On the other hand, data science primarily deals with machine learning and predictive casual analytics.
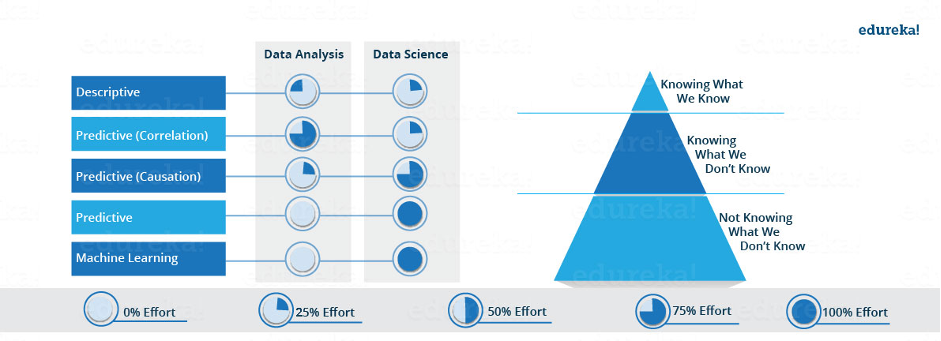
Source: edureka.co
Now that you know what data science is, let us look at what a data scientist does.
Role of A Data Scientist
Generally, a data scientist has to handle a massive amount of data and then analyze it through data-driven methodologies. After they make sense of the available data, they decipher the trends and patterns through visualization and then relay it to the information technology leadership teams. Data scientists also utilize AI, machine learning and programming knowledge such as data mining, BIG data Hadoop, SQL, Python, and Java. Data scientists also require efficient communication skills to effectively translate their data discovery insights to businesses. Let us look at the tasks of a data scientist more closely:
Data Cleaning
Most of the data available aren’t in an easy-to-use format. A data scientist ensures that the data is nicely formatted and conforms to some rules. For instance, a CSV that describes a fast-food franchise’s finances. There will be various columns for state, city and number of burgers sold the previous year. However, the data will be spread across various files that need to be joined together. Making sense of the resulting combination is the difficult part. In most cases, there will be formatting inconsistencies. Data cleaning is all about finding these mistakes and fixing them.
Data Analysis
Data analysis deals with visualization. Through this process, a data scientist tries to simplify the data for communication and understanding. This can be something simple like what event or property signals when new users convert into long term ones or it can be something more complicated like figuring out when someone is trying to scam you out of your money. For instance, data scientists discovered that having at least 10 friends will ensure that a user remains active and as result, there’s a lot of machinery devoted to helping users find new friends.
Engineering/Prototyping
A good model and a clean data is only the tip of the iceberg. For instance, even if a data scientist comes up with a good model for predictions, it doesn’t mean much if they can offer those predictions to customers and do it consistently. For this, data scientists will have to build a data product that can be used by people other than data scientists. This takes various forms; an application, a visualization or a metric on a dashboard.

Source: MedicoReach
Why Is Data Science So Important?
Putting it simply, data science eliminates uncertainty for organizations. Setting up a tech company, coming up with a great product and building traction has become easier due to enhanced connectivity, declining computing costs, cloud storage and the easy accessibility of distribution platforms for reaching target audiences. This has dramatically reduced the time taken by a product to reach the 100 million monthly active users mark. For instance, it took just iTunes 100 months to reach the 100 million mark and for Pokemon Go, it was just a matter of days. The below graph has some amazing examples.

Source: medium.com
Due to the increase in production of products, internet connected devices, and increased time spent online; there has been a massive increase in the volume of user interaction data. As a result, there has been unprecedented data in mining this data and deriving critical insights to help produce excellent products. These days, a company’s competitive ability is measured on accounts of how successfully it is able to apply analytics to vast unstructured data sets covering disparate sources to push product innovation. Due to this phenomenon, data scientists are increasingly in demand and a team of data scientists can make or break a company.
Application of Data Science
Data science pervades almost every industry.
Fraud Detection
Banking and financial institutions make use of data science and related algorithms to prevent and detect fraudulent transactions.
Logistics
Logistic companies utilize data science to optimize routes to ensure quicker delivery of products and enhance operational efficiency.
Recommendation Systems
Major companies including Amazon and Netflix offer product and movie recommendations based on what you like to browse, purchase, and watch on their platforms.
Image Recognition
Detecting objects and identifying image patterns is one of the most popular applications of data science.
Gaming
Data science is now assisting the creation of video and computer games and that’s taking the gaming experience to a whole new level.
Healthcare
Data science is helping healthcare companies build more sophisticated medical instruments to detect and cure sicknesses.
Data Science as a Career
In recent years, there has been an exponential increase in vacancies for data science jobs and related roles. In 2019 alone, Glassdoor named it the number one job in the United States. By 2026, the U.S. Bureau of Labor Statistics that there will be at least 11.5 million job vacancies related to data science. If you’re interested in the data science domain, there are several jobs you could look into. Some important job roles are:
- Data analyst
- Data consultant
- Machine learning engineer
- Data scientist
According to Glassdoor, a data scientist average salary in the United States is about $114,000 USD every year and in India, it’s about 900,000 rupees every year. As of now, data science has become a lucrative career option.
Conclusion
This brings us to the end of our article on what is data science and its importance in 2021. As illustrated above, there has been an enormous increase in the importance and usage of data science. If you want your business to prosper, it’s essential that you bank on data science. Similarly, if you’re looking for the most lucrative job, you might consider data science.