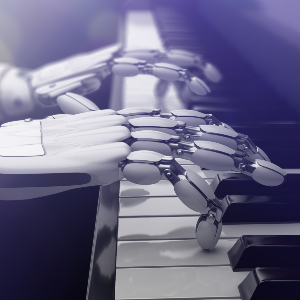
As AI continues to penetrate many areas of our lives, the music industry is no exception.
Although there are many music production-related applications that involve AI, too many of them look like fun science projects without any applicability to the craft. However, in the past few years, the music industry has experienced a somewhat significant impact from AI-powered platforms and plugins for music mastering, arguably one of the least creative stages in music production.
In this article, we discuss the impact and limitations of ML-powered mastering services and provide a future outlook for the technology based on our company’s experience.
What is Mastering?
In general, we can divide the music production process into three stages:
- Composing, recording, arranging
- Mixing of separate tracks to ensure that they sound good together
- Mastering
Mastering engineers enhance and correct certain sonic elements to ensure that the song will sound equally good across different playback systems. When it comes to working on a music album, mastering engineers also ensure that separate songs have a cohesive sound. To achieve this, they change a stereo recording by adjusting its dynamic range, correcting relative loudness of different frequencies, shaping the stereo image, etc. Usually, professional mastering engineers are specialists operating in high-end, carefully acoustically tuned studios, and use expensive audio processing hardware and software.
In the past 20 years, mastering (as well as music production as a whole) has moved from an entirely software-less process to a computerized one, significantly lowering the entry barrier to music production. Spotify recently confirmed that more than 60,000 tracks are now being uploaded to the platform every day. ML-powered mastering platforms are poised to lower the barrier to entry even further.
Although there is a high degree of aesthetic judgement, mastering remains a very technical and complex process, which requires in-depth knowledge of audio processing and respective tools. Previously, independent artists had to either learn to master themselves or turn to expensive mastering engineer services. Today, services like LANDR and CloudBounce introduce one more option: intelligent software that can master songs in a matter of minutes and 10 times cheaper than a human professional.
However, when an ML-powered algorithm has to deal with a largely aesthetic process like mastering, some issues will inevitably be revealed. For example, one of the most recurring problems of bedroom producers is that they don’t have the means to adequately hear and manage low frequencies, which is a problem when it comes to playback system variability. In essence, producers working in poorly acoustically treated home studios often can’t find the sweet spot between taming the bass too much and letting it overtake the whole mix. While there are no statistics on this matter, it would be safe to say that these automated mastering platforms often get mixes with problems in the low-frequency range. Relying on data rather than on hearing, the ML algorithm can quickly recognize if there is too much or not enough bass and fix the problem.
In one study, however, researchers expose the problems that this approach can have. Mastering engineers have purposely tested LANDR’s ability to master a song with a bass solo. In this case, a mastering engineer would typically let the bass shine and make it as loud and as bright as possible. LANDR’s algorithm, however, clamped down the bass, making it dull and flat. While we can only guess what exactly happens under the hood of the ML algorithm, it’s rather evident that LANDR’s engine doesn’t treat the song as a song, but as sonic data. It simply recognized that there was too much information in the low-mid frequency range and dealt with it the way it was taught to.
One can argue that these algorithms can easily learn to differentiate between the instruments, recognize different compositional parts of the song, and ultimately, make more informed decisions. ML, however, can’t learn to understand the aesthetic ideas behind a song.
We can draw a parallel with the AI case for music playlisting. While Spotify’s algorithmically personalized playlist ‘Discover Weekly’ was a real breakthrough for the industry, the company and its competitors still employ hundreds of elite music experts to curate playlists for different occasions. For better or worse, ML can’t exactly understand what songs are perfect for shower singing, distant traveling, workout, etc. It can make educated guesses, but in the majority of cases, the reason for a certain song fitting a particular occasion can’t be measured or explained by data.
Will ML-powered Mastering Eliminate Engineers’ jobs?
The answer certainly depends on the context. What do you consider mastering? For emerging independent artists and music hobbyists, who are often responsible for every stage of music production, mastering often ends with pushing the software limiter to achieve a streaming platform-specific LUFS level. For an experienced self-taught engineer, mastering is about honing the frequency spectrum and dynamic range in a certain direction to achieve a genre-specific sound and ‘vibe’. And then we have the biggest artists in the music industry, who can travel continents to work with a particular mastering engineer who has defined the sound of the whole genre and still has that 1965 version of an LA-2A compressor. In the not-so-distant future, when these services earn enough reputation and fine-tune their underlying algorithms, they can potentially take jobs from low-level engineers. Currently, though, these services are used by people who can’t afford or don’t need a mastering engineer in the first place.