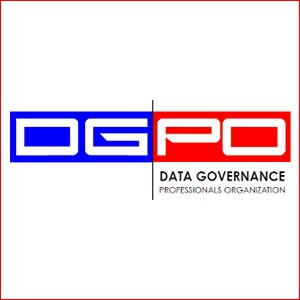
In October 2020, the Office of the Comptroller of the Currency (OCC) announced a $400 million civil monetary penalty against Citibank for deficiencies in enterprise-wide risk management, compliance risk management, data governance, and internal controls. [1,2] The OCC took this action based on observed concerns regarding the safety and soundness of the Bank’s risk management and data governance and internal controls.
In a recent DGPO webinar, Michael Nicosia shared “Data Governance Value Proposition – Understanding Your Data Risk.” [3] This webinar outlined the steps to establish a data risk framework. The recording of this valuable webinar is available to DGPO members on DGPO.org.
Data Governance has become a critical aspect of organizational Risk Management, Governance and Compliance (GRC) platforms. Many companies leverage Data Governance for operational efficiency and overall data quality initiatives. Within the financial verticals, Data Governance has been elevated to a board-level responsibility for managing risk. As the Citibank issue problem points out, there can be severe consequences when an integrated risk management platform, including Data Governance, is not properly functioning. Nicosia made similar references in the DGPO webinar that warrant further investigation.
Not knowing what dangers lurk within the data puts a company’s operations at risk, not to mention their reputation. Auditors, regulatory agencies and examiners are reporting adverse findings in the financial reports of companies. The goal is to ensure the transparency and accuracy with financial disclosures. Non-compliance has direct and indirect costs. Increased audit and consulting fees alone can run into millions and last for years. It is known fact that once people start to doubt data quality in reporting, all aspects of the company become suspect. The net effect is a downward spiral consuming resources, time and money.
There are definitive steps that a company can take to mitigate operational and financial risk. The DGPO defines Data Governance as “a discipline that provides clear-cut policies, procedures, standards, roles, responsibilities, and accountabilities to ensure that data is well-managed as an enterprise resource.”
In this column we are going to explore the three key aspects of Data Governance: Awareness, Controls and Communication.
Governance Awareness
Implementing Data Governance typically starts with asking lots of questions about the source and the use of data. Companies indicate that the answers are always surprising, and not in a good way. During the governance awareness process, managers and analysts are able to articulate what keeps them awake at nights. Awareness questions key in on:
- What is the true source of data within the company (insert the subject area (e.g. loans)?
- Where are copies of the data stored?
- Who is ultimately responsible for the data quality (Data Owner?)
- Who consumers the data?
- What controls are in place to ensure that the copies equal the true source (or system of record)?
The answers to these questions provide a newfound awareness of data across the organization. This information can be shared in some visual form among managers and users within governance organizations, technology, audit, and operations.
Feedback from DPGO members consistently tell us there is no clear understanding of the correct data sources within their companies. Departments are pulling data from different systems. Data used for reporting is inconsistent because spreadsheets are being manipulated to create alternate systems of record. There is also variability with organizational controls. Incomplete validation leads to inaccurate reports and disclosures. Reporting managers suspect that there are data issues in the reporting cycle but lack the evidence that is available in inquiry leading to the governance awareness within an organization.
The above scenario is not an exception, but more of the norm. Data Governance implementations should be designed to eliminate this type of risk by creating awareness of the true source and use of any data within an organization. This problem of inaccuracy becomes even more onerous when dealing with confidential or PII data, or unstructured data as found in Information Governance.
How can this problem still be so pervasive given the focus on Data Governance for so many years? Companies simply cannot associate the data to the governance policies in a consistent manner. Any organizational structure includes a series of procedures, policies, roles and responsibilities to manage data. However, problems can lie within the data that is replicated across many servers and databases. Companies do not typically have the governance metadata structures in place that can tie the actual data back to the organizational structure.
There is an emerging discipline of Governance Architecture that targets this problem by creating a metadata awareness across the countless siloed metadata solutions that came about by vendor and custom software packages. This will be a subject in future columns.
Data Governance Controls
Data Governance controls must be able to monitor the consistency of the data, including reconciliation to known and trusted values at the content level. Technology groups will often implement row count or hash total controls when moving data from point A to point B, as often in the case when loading a Data Warehouse. For example, IT provides evidence that the group successfully moved 1,000 rows of data (row counts), and the sum of the Accrued Interest matched when compared between the 2 systems (hash totals), giving a value of $123,456,00. So far so good, but it’s not enough. Do we know if the correct 1,000 rows were moved? Is the Accrued Interest value of $123,456,00 accurate? What is the true state of the data? Only specific staff knew that there was a loan missing from the source system. The correct row count should have been 1,001 and the total Accrued Interest was $234,567.00. In this example, Accounting updated their spreadsheet. At least their reports were correct. We can’t say as much for the rest of the company’s data.
The key here, is that Data Governance controls need to not only look at data movement, but also reconcile data back to independent data sources. Any discrepancies must be flagged and fixed. In the above example, had the company put in place the proper controls between total loans at the customer level, or total Accrued Interest within customer statements against the Data Warehouse, this problem would likely have been caught.
Here we are only talking about one loan. In many cases, the magnitude of errors is much more significant, as could be found digging into the details of the recent Citibank issue.
Data Governance Communication
Effective Governance Awareness includes targeted communication. The business rules (Metadata) within a Data Governance awareness matrix can provide the specific people or departments that care about any given domain or subject area of data at a point in time. These are the Registered Governance Stakeholders, the people who need to be proactively alerted about any real or potential data variances. Governance stakeholders are the ones who are held accountable when governance, control or compliance frameworks fail. With such high stakes it’s no surprise they that they can be the biggest proponents of these system improvements for their organizations.
Wikipedia defines risk as, “the possibility of something bad happening. Risk involves uncertainty about the effects/implications of an activity with respect to something that humans value (such as health, well-being, wealth, property or the environment), often focusing on negative, undesirable consequences…The understanding of risk, the methods of assessment and management, the descriptions of risk and even the definitions of risk differ in different practice areas.” [4]
Data Governance is a structured discipline for addressing all aspects of data-related risk. It becomes a competitive game-changer when the focus is top-down, business-driven, technology-enabled, and integrated tightly into the value chain of the organization.
The Data Governance Best Practices Framework on DGPO.org outlines proven experience for the advancement of Data Governance. This guidance based on community input from real-life lessons can be used to solve the issues outlined in the column along as many different facets of Data Governance.
Citations
[1] https://occ.treas.gov/news-issuances/news-releases/2020/nr-occ-2020-132.html
[2] https://www.occ.gov/static/enforcement-actions/ea2020-056.pdf
[3] https://dgpo.org/members-only/dgpo-events-recordings-and-presentations/webinars/
[4] https://en.wikipedia.org/wiki/Risk
Upcoming DGPO Webinars
January 5, 2021 – 2:00 PM ET
Best Practices in Data Governance Communications
Malcolm Chisholm, Data Millennium
Webinar Link: https://us02web.zoom.us/webinar/register/3116079165399/WN_koLxaIBeQeajBOn7MF1kqQ
February 3, 2021 – 2:00 PM ET
Evolving Data Governance for Vanguard’s Next-Gen Infrastructure
Jason Caplan, Vanguard
March 3, 2021 – 2:00 PM ET
Information Risk Management for Ensuring Data Confidence
David Loshin, Knowledge Integrity
Interested in Networking with over 4900 Data Governance Professionals?
Join the conversation in the Data Governance Professionals Organization LinkedIn Group.
To learn more about the DGPO and data governance best practices, visit DGPO.org and look for e-mail communications featuring regular updates and registration links for our webinars.
The DGPO is a non-profit, vendor neutral, association of business, IT and data professionals dedicated to advancing the discipline of data governance. Members have access to a community of practitioners, industry expertise, timely best practice articles and materials, as well as discounts to relevant industry events. If you would like more information about the DGPO and benefits of membership, please check out the DGPO Website.