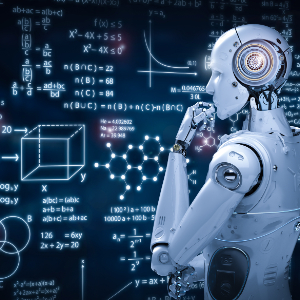
The domain of AI and data science so far has created significant value in the technological landscape. However, the path to reaching success isn’t easy and quick. With multiple technologies involved, even deep learning algorithms can’t do the trick. 2019 witnessed record-breaking AI funding, and it’s mostly possible because, over the years, decision making has transformed dramatically. The website and application development sector focuses entirely on the upcoming predictions and trends to survive. Some 2020 predictions are listed below.
End-To-End Model Management
When it’s time to move onto the production stage of AI models, end-to-end management becomes the most excellent practice to adopt as far as production is concerned. Over the years, the popularity and attention AI garnered has been nothing short of incredible. As the scope, size, and digital footprint of AI projects grew massively over the years, it’no surprise that the growth and progress of the industry has opened new doors of opportunities.
Because of the significant increment in the number of AI and data science projects launched every year, the number of problems faced became quite substantial. Thanks to end-to-end management, the problems came together to form a foundation for model management. It includes model deployment, identifying the need for retraining or rebuilding models, model monitoring or Model Ops, and, most importantly, various layers of support.
AI Chip Market Thriving with Potential
Artificial Intelligence chips are silicon chips, specialized in optimizing machine learning algorithms. Used in many industries, the chip aims to solve computational and mathematical problems to boost efficiency in systems. The development of these chips increased global competition in the artificial intelligence chip market. From autonomous robots to smart home applications, AI chips carry huge potential in transforming the tech industry globally.
The AI chip market is expected to value around $91,500 million by the year 2025. It’s not about the chip anymore, but about experiences. Huawei, Alibaba, Intel, Facebook are some popular companies investing in this particular market. Due to high competition and the significant impact of a powerhouse, companies such as Google and Amazon in the market only push startups to strive for more.
AI Automated Decision Making
The power of technology lies in its ability to produce precise results. Whether it’s through an app or software, technology has completely transformed the way things work, and with data science or AI incorporated, it’s only fair to expect a revolutionary experience.
Combining decision making with AI automation has definitely caused some concerns in the past. Examples would be when Amazon used a recruiting tool that displayed gender biases and the famous Cambridge Analytica scandal that used more than millions of Facebook profiles to influence political agendas.
However, this provided a bird’s eye perspective on the ethics involved in data science and AI. To create a more safe and personalized experience, AI and data science ethics are now fixated on a few focus areas such as privacy and consent, the inclusion of a human in the process, algorithmic partiality, and automated decision making.
It’s necessary to align AI and data science ethics with global privacy standards. In some countries, profiling and data processing is limited, with maximum transparency needed to be sustained. This helps in getting rid of unnecessary constraints while working with newly established AI and data science ethics.
Trend Towards Specialization
When it comes to data science and AI, we’re expecting continued growth of specialization in these roles. Divided into two categories, ‘engineering-heavy’ and ‘science-heavy’ data science roles focus on different aspects of the data science space. ‘Engineering-heavy’ roles include machine learning, AI, and data engineers, focusing on the infrastructure, platforms, and production systems. ‘Science-heavy’ data science roles include analytics consultants, data scientists, and business analytics specialists, focusing on decision and inquiry work.
Demands for these roles are increasing rapidly as AI models move to production on a daily. The trend in the specialization will continue to grow over the next few years for sure.
Being multi-talented definitely garners a lot of attention, however recruiting those who excel and specialize in one field will help you greatly in the long term (when you’re investing in your startup).
More Tools, More Confusion
In this digital age, you can easily find a plethora of tools available to complete any technical task. Although there are several ways of approaching a particular problem, this causes great confusion amongst people new to the industry.
While you have a myriad of options available to choose from, narrowing down the options according to what your work is will clear most of the confusion. Some examples include:
- If you’re a software engineer, choosing machine learning APIs to create an AI app or software works well.
- Using Microsoft SQL Server to model in a database helps when you’re a database administrator.
- Going for Azure ML Studio if you’re an expert with the cloud helps you build powerful platforms.
The possibilities are endless, and you can never be too sure of the perfect tool to build your AI platform.
AI Literacy
The Artificial Intelligence industry not only contributed to the technological advancements in the world, but it created immense value by forming human-centric experiences. To understand AI advancements, one must know how to use them. Several industry experts believe a lack of understanding is one of the major reasons why many don’t realize the value of data science or AI.
Most organizations offer complete training and education to their employees about any in-house programs available. Though an investment, it allows the companies to re-assess problems and data differently with complete understanding.
Architecture Patterns
The shift in the digital landscape demands for sophisticated and creative architecture patterns. Bigger models demand better architecture patterns. To compute and transfer data seamlessly for edge computing, real-time video analytics focus on providing real-time video content through private clusters and public clouds. Computer vision algorithms have the power and potential to face challenges in this area.
Creating Value from AI and Data Science Is Easier
Many industry players think creating AI or data science apps and software isn’t the tough part. Discussing and consensus between the technical AI and data science roles is necessary as well. AI systems are optimized to provide the best personalized possible. It all depends on doing things properly with architecture, data, and modeling. These decisions are generally taken by non-technical seniors.
2019 was an incredible year for the artificial intelligence industry in terms of published papers and AI funding. There is no doubt 2020 looks quite promising as well.