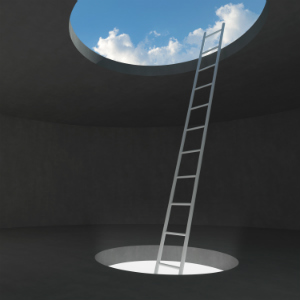
Data Acumen, Literacy, and Culture
Data literacy, or data acumen[1] as we like to call it, is increasingly cited as a critical enabler of being a data-driven organization. The trouble is it isn’t well defined. We set out to do something about that and developed a data acumen quick reference. Using the quick reference, folks across the organization can see themselves with regard to data use and evaluate their data acumen.
To orient ourselves we begin with data culture. It is a closely related, term to data acumen, and like data acumen, it’s also emphasized as critical to success in a data-driven enterprise. But it also isn’t well defined. Even within the data community, its meaning isn’t clear. So here are some definitions we like:
- “…the collective behaviors and beliefs of people who value, practice, and encourage the use of data to improve decision-making. As a result, data is woven into the operations, mindset, and identity of an organization.”[2]
- Forbes’ Thriving Data Culture[3]
- Executive leadership owns and drives the use of data
- Data champions break silos between teams and promote collaboration
- Data is trusted, easily accessible, and freely shared
- Data literacy is considered a critical skill for every role
- IDC Elements of Data Culture[4]
- People: expectations of people using data in their jobs; how employees are encouraged to develop data competencies
- Attitudes: encouragement of experimentation and elimination of the fear of failure; fostering data curiosity
- Values: Organizational stated mission around the use of data; Decisions using data as a leadership role-model
- Goals: transparency around business metrics; evaluation of how employees are using data
- Social: forums for ideation and innovation with data; fostering internal and external collaboration
Looking at these definitions, it’s quickly obvious that embracing the effective use of data across the organization by everyone is the key to data culture. How, then, does data culture translate into data literacy or acumen, and who needs it? As you might imagine, definitions of data literacy are closely related to data culture, and many people beyond data professionals are projected to need it. For example:
- “…the ability to make good judgements about the use of data to support problem solutions. It is not only the basis of statistical and quantitative analysis; it is a critical mechanism to improve society and a necessary first step to statistical understanding”.[5]
- “…the ability to read, write and communicate data in context, including an understanding of data sources and constructs, analytical methods and techniques applied, and the ability to describe the use case, application and resulting value.”[6]
- “We envision three types of readers with
intertwined goals:
- Individuals reading to learn how to better manage their own data
- Individuals hoping to learn what their organizations should do to improve organizational data literacy, and
- data professionals interested in what they can do to help move our profession, the citizenry, and society forward.”[7]
In the last definition above, Peter Aiken and Todd Harbour really expand on who should be data literate, a case they present in their book. While this is a commendable goal, for the data acumen quick reference we focus on data acumen for professionals.
Having established the close relationship between data culture and data literacy, one other point is worth noting before we dive into the quick reference. That is, data culture continues to be the number one roadblock to success cited by Chief Data Officers (CDOs). For example, the recent “Data and AI Leadership Executive Survey 2022” highlights that 90% of respondents cited data culture as a roadblock to success.[8]
The Data Acumen Quick Reference
To help mitigate this lack of data culture, we developed a data acumen quick reference. Our aim was for people involved with data in an organization to see themselves in the quick reference and make a reasonable assessment about what data skills they have, should emphasize, and would be helpful to acquire.
The quick reference highlights key data skills and data knowledge needed for common functions (e.g., data science, data stewardship, data architecture, records management, data engineering) at all levels of an organization (i.e., for everyone, for staff, for managers, and for executives) across the data lifecycle from creation to retirement. It isn’t meant to be prescriptive, but it is meant to convey the importance of data acumen (or literacy) across the organization and to help practitioners better understand data management skills and needed agency data knowledge. We also aimed to align it with— and extend on— the Open Data Institute’s (ODI) Data Skills Framework.[9] Following is a summary of the kinds of data skills needed in the various job functions.
- What Everyone Should Know: basic security, records management, organizational policies, relevant business domain, critical thinking, statistic interpretation, and ethics. Understanding how to answer questions and escalate data issues (staff & managers); understanding use of data for decisions (managers & staff)
- Data Science: from wrangling big data (staff), to understanding tools and environments (managers) to advocating for and effectively integrating an analytics program (executives)
- Data Analysis: Translating business problems into underlying data (staff), data calls (managers), championing use of data to solve business problems (executives)
- Data Stewardship: Translating business problems into underlying data (staff), cultivating deep domain knowledge (steward); championing data management and secure funding (executives)
- Data Governance Operations: Facilitating data initiatives (staff); creating data strategy roadmap (managers); championing data management and securing funding (executives)
- Data Architecture: Defining data lifecycle and understanding data platforms (staff and managers); understanding major technology / data gaps (executives)
- Knowledge Management: Skills in applying taxonomies and unstructured data management (staff); understanding knowledge management platforms and discovery (managers); understanding major data architecture technologies / data gaps (executives)
- Records Management: Experience with data lifecycle of structured and unstructured data (staff); application of policies and strategies (managers); knowledge management resource allocation (executives)
- Data Engineering: Developing and maintaining data pipeline (staff and managers); deep understanding of IT infrastructure and associated strategy (executives)
Our aim was to fit the quick reference on one 11×17 sheet. In the end, the full quick reference spans about four 11×17 pages. We had many suggestions from data management subject matter experts some of which, while very good, would have turned the quick reference into something much larger. To provide an idea of how the quick reference looks, below is a sample snapshot.
So far, we’ve found significant interest in the quick reference. We are evolving it in a number of ways. For example, we plan to publish learning paths— many of which are free or low cost— to obtaining the skills needed for the functions and organizational levels reflected. The full quick reference is publicly available— just contact this author through the email listed in the bio. We’ve also obtained an open collaboration license, so if you are interested in co-evolving the quick reference, just let us know.
———-
This article was written by Mike Fleckenstein. Mike has over 30 years of experience leading data management initiatives. His range of practice includes founding a data solutions company, acting as a trusted advisor to CDOs and other senior executives, and guiding enterprise-level data programs & projects. As Chief Data Strategist at MITRE, He supports numerous data-specific efforts across the federal government, including shaping the Federal Data Strategy. Mike has been recognized by the American Council of Technology’s (ATC) and Industry Advisory Council’s (IAC) for Excellence and by Computerworld as an Honors Program Laureate for Data Governance and Enterprise Architecture. Mike teaches and holds workshops in data management and CDO Fundamentals both within and external to MITRE. He is a known speaker and author on data management topics. In 2018 he co-published the book “Modern Data Strategy”.
[1] We’ve noticed that folks are sensitive to the term “literacy”, interpreting it as being illiterate. We’ve found greater acceptance of the term “acumen”. Industry uses both terms interchangeably.
[2] “What is Data Culture?”, https://www.tableau.com/why-tableau/data-culture, Tableau, accessed July 2022
[3] “4 Key Signals That Indicate A Data Culture Is Thriving In Your Organization,” Forbes, https://www.forbes.com/sites/ganeskesari/2021/08/25/4-key-signals-that-indicate-a-data-culture-is-thriving-in-your-organization/?sh=7efc23be30bb, accessed July 2022
[4] “Become an Intelligent Enterprise by Creating a Data Culture”, IDC, https://info.idc.com/rs/081-ATC-910/images/FoIt-eBook_Become-an-Intelligent-Enterprise-by-Creating-a-Data-Culture.pdf?utm_medium=email&utm_source=operational&utm_campaign=us-idc-foit-data-culture-ebook&utm_content=mkto-1427-em-00&utm_idc=emo-mkto-1427-idcus&mkt_tok=MDgxLUFUQy05MTAAAAF9kTeVP1fJVGpDCN4UbMKJ-Zrc9-uWhGsvNV8Ep9rUB4-gdD6hi_fQj2C3_AOl8B-hvuHLJkn4v2vr0MAPCx7o8Gyr7k41FpYFMoQLbs-v#:~:text=IDC%20has%20found%20that%20a,with%20an%20established%20data%20culture, accessed July 2022
[5] Keller, Sally Ann, et. al. “Doing Data Science: A Framework and Case Study”, Harvard Data Science Review, Issue 2.1, Winter 2020, https://hdsr.mitpress.mit.edu/pub/hnptx6lq/release/9#:~:text=We%20define%20data%20acumen%20as,first%20step%20to%20statistical%20understanding accessed July 2022
[6] “A Data and Analytics Leader’s Guide to Data Literacy”, Gartner, August 26, 2021, https://www.gartner.com/smarterwithgartner/a-data-and-analytics-leaders-guide-to-data-literacy accessed July 2022
[7] Aiken, P., Harbour, T. “Data Literacy Achieving Higher Productivity for Citizens, Knowledge Workers, and Organizations”, Technics Publications, 2021
[8] “Data and AI Leadership Executive Survey 2022”, https://www.newvantage.com/_files/ugd/e5361a_ad5a8b3da8254a71807d2dccdb0844be.pdf, accessed July 2022
[9] “Data Skills Framework”, ODI, https://theodi.org/article/data-skills-framework/, accessed July 2022