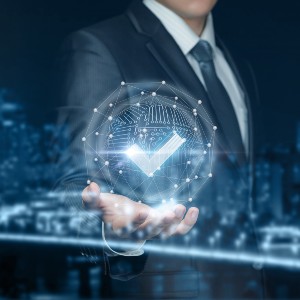
A systems thinking approach to process control and optimization demands continual data quality feedback loops. Moving the quality checks upstream to the source system provides the most extensive control coverage. Data quality approaches not utilizing these loops will fail to achieve the desired results, often worsening the problem.
Data Governance is about gaining trust and control over an organization’s data assets. There has been a steady progression in the maturity of data management, which today focuses on data governance, information governance, and metadata governance. Significant breakthroughs have also occurred in the relationships between these disciplines, which are integral to Enterprise Data Risk Management. Unfortunately, any further progress will be hindered by linear thinking.
Linear or event-oriented thinking considers only the interactions between data, process, system, and time. In contrast, systems thinking looks at feedback loops, interactions, and the impact of time. Systems thinking addresses the root cause of a problem, not just the symptoms.
To solve data governance or risk management issues effectively, you must view your organization as an entire system — the lean thinking discipline that revolutionized the factory floor demonstrated systems thinking. Our lean approach applies those same concepts to what we call a company’s “data factory.”
So, what is a feedback loop? Think about a thermostat in your home. This device asks, “Is the temperature correct based on the desired setting?” If the answer is no, it sends instructions to the HVAC system to adjust the temperature. If yes, it sleeps for a few seconds and then asks the question again.
Let’s apply this to the fundamentals of data quality. Tech-enabled governance continually monitors the quality, state, or data. This includes the data movement across the data factory (e.g., data warehouse load) or following some event (e.g., income forecast model results). You want to answer the question, “Is the data correct?” regarding business risk appetites, business needs, budgets, and other critical priorities. Lean thinking, on the other hand, poses the question, “Is the data in line with the minimum quality standards?” which are often set by end consumers. This topic is a separate column for another day.
Before delving into a tech-enabled approach, look at the typical data quality cycle. It starts with a business unit detecting an error in a report, or worse, an auditor or regulator uncovers it. More controls are then added, many of which are manually performed. Treating only the symptoms of insufficient data in this manner will worsen the problem. You end up with a negative reinforcing loop of more controls and people. Plus, the data quality problems remain.
We offer a better approach using applied technology and governance metadata. Consider the flow of data in an average data warehouse load. Transactions and their associated details are maintained within source systems. This data becomes a warehouse as part of a daily (or hourly) closing cycle. Governance specialists understand this flow as a series of metadata components, creating a complex puzzle represented by source systems, data objects, target systems, data owners, and data consumers.
Companies with effective control over their metadata and an integrated data quality tool can define automated controls that monitor the data quality quickly, with anomalies instantly reported to registered stakeholders. This continual feedback loop maintains total awareness of the actual state of the data across the enterprise. Like the thermostat in your house, this configuration keeps everything working as it should.
Russell Ackoff, a pioneering systems thinker, often said, “Successful problem solving requires finding the right solution to the right problem. We fail more often because we solve the wrong problem than because we get the wrong solution to the right problem.” Fast-forward and he could have been referring to tech-enabled governance.
As evidence, spreadsheets remain one of the most used formats to fix data issues. Here’s the scenario. It is late at night, and the report to the regulator is due by 10:00 p.m. There is no time to update the source and reload the data warehouse. Data will be updated in the spreadsheet. The report gets sent out with the correct data on time. From the business unit’s perspective, this task was completed. But was it? The continual data quality feedback loop and the communication to all stakeholders ended on that spreadsheet. However, was the source system ever updated? Is the rest of the company working with the same new data? Most likely, the answer is NO.
Companies that run continual data quality controls will detect this data imbalance when they run subsequent controls. Updating the data in the spreadsheet will keep the system balanced in one or more areas. We need to assume the total set of data consumers were not involved in this spreadsheet correction and, therefore, are working with a different data set. This situation is a primary cause behind negative audit and regulatory findings.
A systems thinking approach to process control and optimization demands continual data quality feedback loops. Incorporating the governance metadata can provide a simple solution given the correct governance technology stack. Moving the quality checks upstream to the source system provides the most extensive control coverage. Further, to catch issues with spreadsheet updates, the controls must incorporate final data results, not just system-to-system data movement. Examples include balancing the general ledger or reconciling customer statements. If your organization is serious about data quality, a systems thinking approach will chart a path to the solution.
Systems thinking takes us back to the fundamentals. Basic but proven feedback control loops that monitor the health of any process. Think about your car dashboard, the controls of an airplane, a refinery process control, etc. These are all system-thinking feedback loops at work. Data Quality is no different. Adequate process controls are dependent upon the quality of the technology deployed. Unfortunately, not all quality tools are created equal. We get lost in the marketing hype, but the real litmus test of success is business testimonials of quality improvement and process optimization. In the next column, we will expand on automated process monitoring and predictive analysis as part of an ongoing quality control framework.