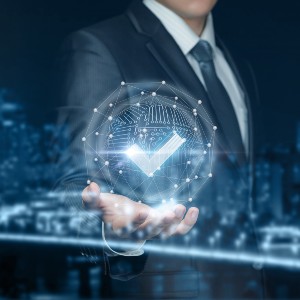
Data inventory optimization is about efficiently solving the right problem. In this column, we will return to the idea of lean manufacturing and explore the critical area of inventory management on the factory floor. More and more firms are implementing lean inventory management techniques to reduce costs, improve flexibility and focus more on their customers.
FlashGlobal is a supply chain logistics company that promotes lean inventory management, stating that “Lean refers to a systematic approach to enhancing value in a company’s inventory by identifying and eliminating waste of materials, effort and time through continuous improvement in pursuit of perfection.”1
Many disciplines have emerged from lean manufacturing, including Just-In-Time inventory, Six Sigma, and Total Quality Circles. The work pioneered by Toyota and management gurus such as Eliyahu Goldratt and W. Edwards Deming transformed inventory management into a science through repeatable practices with proven results measured by bottom-line profits and customer value.
They eliminated the waste in inventory by reducing the number of spare parts, making certain that the right component was at the right machine when needed. They knew the quality of an automobile was directly related to the quality of the parts. Despite the benefits, the move to lean manufacturing did not happen overnight. More importantly, it could never have been successful without significant change in a company’s metrics of success.
Back to the world of Data Governance and Enterprise Information Management. We maintain that the parts inventory on the factory floor equates to the data and information across a company’s technology landscape. Companies are really data factories. This allows us to apply the same principles that returned factories to profitability and increased customer value through a focus on total quality.
The greatest impact is realized by optimizing inventory, or in the case of Data Governance, data. This includes raw structured data that exists across so many databases, spreadsheets, and data warehouses within the same company. We will address unstructured data and records in subsequent columns.
In the 1980’s, manufacturing companies had a choice to go “lean” or risk declining profits. The first step was to reconfigure the factory floor. Specifically, the way inventory was managed. Upon arrival, lean specialists found warehouses full of excess inventory, often outdated. Quality issues were rampant. Throughput was hampered by many bottlenecks. Regardless of how many cars came off the production line, the old corporate metrics of units sold took no account of the capital invested and labor costs to produce each unit. This explains why many manufacturers were losing more money than they realized until it was too late. Does any of this sound familiar to those of us in the governance world?
MetaGovernance did a walkthrough for an insurance company that was struggling with data quality problems and the associated regulatory oversight issues. We found redundant data copies, poor data quality, and unknown system of record. The company had no awareness of the flow of data across their systems. Over a span of years, databases had sprawled out of control due to lack of architectural oversight. In the end, the system failed to accomplish their business objectives. As a band-aid solution, spreadsheets were used to fill the gaps. The true picture of their inventory of data simply was incomplete. The problems this company faces are not unique.
Our solution was to follow the path of lean manufacturing to optimize their data. We wanted to show proof of concept, starting with the division having the greatest potential for risk. Next came the tasks of evaluating the management of their data inventory.
Using the power of governance metadata, we developed the awareness matrix showing the relationship between the businesses and their data. With this matrix the company was able to associate the familiar Data Governance roles of owner, steward, consumer, and delegate with the subject areas of data back to the businesses. They could now associate the information security risk profile with the data through classification and risk tags. The awareness matrix became the point of focus for all subsequent conversations, identifying specifically who needed to be included in discussions on data management.
We then worked on the problem of source and copies of data. Working with the SMEs, it was very easy to put this puzzle together. Governance metadata was again used to capture this relationship at the subject area level. In the case of risk or critical data, we extended the knowledge down to the key business attribute level along with their associated physical copies, or data objects.
Following the footsteps of the lean pioneers who walked the factory floor, we were able to determine the “lay of the land” — the data landscape for this division. The first step in optimizing their data inventory was to identify, classify, label or tag it. This was accomplished via the partnership with the SMEs and the use of governance metadata.
The next priority for this client was to assess the overall data quality of their key critical data. Like the factory foreman, they understood that the quality of their financial statements and customer services were directly related to the quality of the underlying data. One of the interesting aspects of this approach to data inventory optimization was that we had at our disposal the key relationship between the information and data architecture from initial interviews or documents the client had provided. Determining the quality of this data was a simple step of comparing the data objects against each other for consistency and reconciling the data against known valid sources. This puzzle is not so daunting to piece together once you understand the power of governance metadata and look at the problem through the lens of lean thinking.
Data inventory optimization is all about solving the right problem in an efficient manner. In subsequent columns, we will expand this idea into ways for identifying waste across the data factory to optimize total customer value through applying the concept of total quality management to the underlying data.