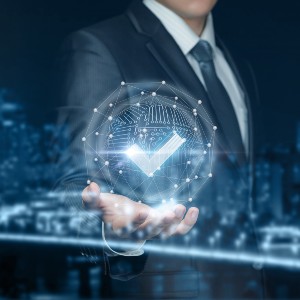
In this column, we will discuss a common problem for data warehouses that are designed to maintain data quality and provide evidence of accuracy. Without verification, the data can’t be trusted. Enter the mundane, but necessary, task of data reconciliation.
This is often a time-consuming and wasteful process. Fortunately, it doesn’t have to be. Lean Governance will transform your outdated methods for verifying data quality. Wasted resources can then be redeployed for higher use.
Lean Governance applies the techniques of Lean Thinking to data management. The basic principles of Lean Thinking are quite simple:
- Only focus on activities that add value to the customer
- Execute those activities with the least amount of time and resources
Lean Manufacturing revolutionized the factory floor, beginning with the production of automobiles. The focus shifted from maximizing production (units) to satisfying the customers (quality). Here’s how the customer defined value:
- To buy the exact model they wanted
- With the desired options
- Free of defects
- Delivered on time
- At a reasonable price
Lean Thinking is all about delivering value to the customer. While we aren’t building cars, we can apply this approach to Data Governance. The goal is to supply your business units with the data, information, and reports they need to perform their jobs. To best analyze all the activities that support the production and management of data we use the analogy of a factory— a data factory.
So, who are the customers in our world and how to they define value? The majority of industry Data Governance Stewardship models name Data Owners and Data Stewards as customers. In our view, this doesn’t go far enough. We would add Data Consumers as a class of registered stakeholders, both internal and external to the organization. In the words of a client, “Understanding our data consumers has been a game-changer.” Our governance metadata model makes this possible.
Let’s review the process of Data Warehouse reconciliation. In this case, we focus on Data Consumers who define value in the form of data that is:
- Well defined
- Accurate
- Timely
- Complete
- Verified
Data Consumers also want a system in place in which variances are self-documented and reconciliation takes minutes, not hours or days. They want to know – and trust – the state of the data at all times. Below we offer two scenarios that demonstrate the power of Lean Governance by contrasting a typical data warehouse with a lean data factory.
Scenario One – Typical Data Warehouse
The process of data reconciliation starts when there is notification that the data warehouse has been loaded. The next step is to confirm that data quality meets individual departmental standards. This task often involves the use of multiple spreadsheets to extract and reconcile the data. Verifying balances against the general ledger is common as it provides a match between the operational and financial data. This reconciliation is performed by multiple departments simultaneously. Without coordination, chances are that many people will be unaware of the results and who is responsible for what needs to be done. Due to time constraints, any data problems found are corrected in standalone spreadsheets. After countless hours or even days, departments determine the data is “good enough” to proceed with their operational and reporting activities. The scenario is all too common. Even though the system appears to be working, it does so at a tremendous cost (waste) while putting the enterprise data at great risk.
Scenario Two – Lean Data Factory
After data is loaded to the data warehouse, the technical event scheduler runs the next set of jobs that reconcile the data automatically against predefined requirements and quality standards. Data Owners and Data Consumers receive targeted messages that the data is accurate or has issues. Any variances are recorded and available at the transaction or balance level. This information is shared across the enterprise for everyone who needs to know. Evidence of the reconciliation is retained for audit purposes. The process runs in minutes. Manual and redundant efforts (waste) have been eliminated. Enterprise risk is managed in a continuous process.
The difference between the two data reconciliation scenarios can be measured in hours saved, often exceeding 1,000 hours per month. We have found that data workers can spend up to 20 percent of their time collecting and verifying data. We call it a “waste tax.” Lean Governance can refund this tax back to the organization. Along with the significant gains in operational efficiency, the reduction of enterprise risk can also be calculated based on assessment against known risk standards such as COBIT, NIST and the expectations of regulators.
The switch to lean does not happen overnight by corporate edict. It happens in stages depending on the ability of each company to mobilize its key players. One of the first agenda items is to reimagine the role of Data Governance. We’re talking about a cultural change in which an organization moves from a bureaucratic-driven model to a focus on data quality and eliminating waste.
In most cases, existing technology assets can be aligned to run lean. Manufacturers learned years ago to resist the urge to buy a bigger machine to eliminate bottlenecks on the factory floor. The main goal is awareness. Awareness of the system of record and reconciliation points. Awareness of Data Owners and Data Consumers, along with roles and responsibilities. Awareness of the flow of data, or lineage. Awareness of the minimum quality standards. And the ability to track this awareness as metadata can help you manage production quality across the entire data factory.
There was a mad dash in the 1980s to remain relevant, and profitable, in the manufacturing world. Companies engaged lean efficiency experts to walk through their factories to find waste and solve quality problems. The data management world faces a similar opportunity to root out waste and deliver quality.