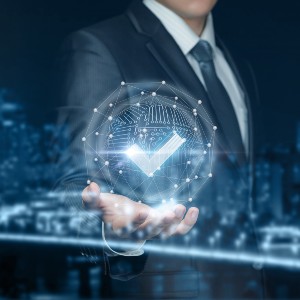
The concept of “walking the data factory” drew a great deal of interest during our recent DGPO webinar on data classification as part of a holistic governance program. We discussed ways to connect the stove-piped worlds of data governance and information governance under a common governance classification. There is great power in lean governance when you can identify and resolve the biggest risks and sources of waste within your organization.
MetaGovernance builds off the success of lean manufacturing in our methodologies. (See our previous TDAN columns and DATAVERSITY blog posts for more on this topic.) We equate the manufacturing and processing of data with what takes place on the floor of any production facility, be it for cars, clothing, or food. The process starts with raw materials and ends with finished goods to demanding customers.
To better explain the idea of walking the data factory, some context is helpful. When converting a manufacturing plant to Lean Manufacturing, the first step is to conduct a current state assessment. Investigators are invited to triage the problems. The checklist is extensive, including:
- Just how misaligned were the processes in place?
- How much waste, rework, risk, and customer disdain existed?
- To what degree were corporate policies and metrics causing the plant to fail?
This assessment is often performed discretely as a special project by a very small group of individuals, often reporting to the board or chairman. You can read more about this trailblazing period in books including “Lean Thinking and The Machine That Changed the World” by James P. Womack, et al.
On the factory floor, waste typically accumulates due to inefficient inventory management or manufacturing yields well below targets. A classic example would be a pile of work-in-process inventory stacked behind one machine, while the machines down the production line sit idle. This inventory pile was the proverbial “Herbie” from Eli Goldratt’s classic book, “The Goal.” He said when you resolve the “Herbie,” you fix the flow.
Enterprise data management is akin to manufacturing. Instead of cars or widgets, we are producing data and information that is processed for analytics, disclosure, and reporting. Minimum quality standards must align with the corporate risk appetite and profitability goals. Potential liability for a firm includes financial risk and reputational risk. Symptoms of problem areas include bad data, customer complaints, and declining orders.
Webinar participants asked what walking the data factory looks like in an IT setting. We share below what we’ve learned from our remediation work in restoring technology data systems to full health and efficiency.
Spreadsheets Continue to Rule the Day
Walk through most accounting or financial reporting departments on the days (and nights) leading up to the deadline of a key disclosure, e.g., Form 10-K, and you will see that Microsoft Excel is the most widely used software. On the computer screen, we see beautifully formatted corporate reports ready for signatures and forwarding to the appropriate agencies. Unfortunately, these reports can include errors that can’t be corrected before the filing deadline.
We all prattle about fixing data at the source. Tell that to the accountant who discovers that the source data is bad, and time is running out to update the source transactional system, reload the data warehouse, and regenerate the report. The only choice is to update data in the spreadsheet and generate a new report with the corrected numbers. In most cases, these spreadsheets are considered end-user applications from a SOX perspective. As such, manual controls and approvals are needed to remain in regulatory compliance. In addition to significant wasted effort, this ongoing scenario introduces risk for it is rare that the actual data changes make it back to the source system. When this happens, the entire company is exposed to bad data.
The Added Cost of Manual Data Reconciliations
Many people take pride in their work. They want their work to be accurate. In our world, this requires quality, trusted data. Talking to data consumers is a key tool for detecting waste, and what we find is the excessive use of manual reconciliations. Like the factory machine operator who must redo work caused by bad parts, business units must often re-run their reporting, underwriting analysis, or modeling caused by bad data. To protect themselves, they reconcile the data often back to an official set of numbers like the general ledger. They also might reconcile to another system, like customer invoicing, that should also have the same set of data. Again, the spreadsheet is the tool of choice.
This approach flies in the face of Lean Governance. Why do highly skilled people have to dump data from multiple systems into spreadsheets to see if it agrees? The data resides in a source system or data warehouse that should be reconciled on the enterprise level. Why aren’t the reconciliation results posted and shared? During a recent month-end reporting process, we identified at least ten departments reconciling their balance sheets back to the General Ledger, each one having found and resolved errors. This is a massive waste and unnecessary risk.
The above two examples are but a small sampling of what you’re likely to see when walking the halls among data consumers at most companies. Lean Thinking can change the future way of doing things. It requires being open to new approaches to enterprise data management and governance. The goal is to create a more robust and efficient data factory, with a higher throughput at a lower cost. As mentioned in my last column, it requires an integrated governance technology platform that validates the source, use, and state of data quality to meet the expectations of the data consumers, the true customers.