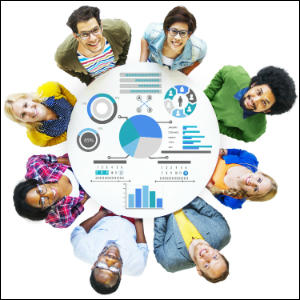
Several years ago, as part of some strategic marketing, we decided to target certain companies and industries. The thinking was and is, that certain sectors are inherently more amenable to the economic arguments behind the data-centric approach.
We didn’t do any market research, didn’t do any surveys, didn’t purchase any reports or data, we just declared some markets more likely to yield fruit than others. After pursuing this for about four years, we got some right and missed the mark on others. This article is about what we learned and some hypotheses as to why this is so.
What Did Go into Our “Analysis”?
Even though we didn’t do any formal research, it’s not like we were completely clueless. We’ve been promoting a data-centric approach to a broad market for ten years, and therefore, we get some feedback as to who is picking up the signal and who isn’t. Our marketers and senior consultants have been in the enterprise systems space for many more decades, and from that we’ve seen how decisions are made, how initiatives are funded and how firms choose to transform themselves.
We also co-produced the Semantic Technology Conference for 10 years and continue with the Data-Centric Architecture Forum and the Enterprise Data Transformation Symposium. So, we have a front row seat, just not a lot of hard data.
We postulated that at least two things needed to be present for someone to embrace data-centric:
- Significant economic pain from decades of perpetuating the application-centric approach to silo building.
- The economic capacity to take on a long-term transformation project.
There are a couple of corollaries to the above:
- The target firms need not be aware of their pain, nor of the source of it. In some cases, we understand that it may be our role to educate them. Obviously, sales cycles are shorter if they are aware, but we didn’t make that a precondition.
- There doesn’t need to be a major capital commitment to data-centric, indeed right now such a commitment would be hard to get, because this isn’t yet mainstream. ERP and CRM implementation projects, Legacy Modernization projects, and even Digital Transformation projects are regularly funded to the tune of hundreds of millions of dollars at large firms, partly because executives believe that “everyone else is doing this” (mostly because everyone else is doing it). While we don’t expect our targets to fund multi-year capital projects to become data-centric, we do have to look to companies that have the capacity to fund these initiatives as they go.
Our Original Targets
We ended up splitting potential opportunities into three categories:
- Target – These are industries and individual firms that are known knowledge graph implementors and should be expected to be good near-term prospects.
- Opportunistic – These are areas where we have seen very little uptake, but logic suggests they should, eventually, be candidates. These are areas where there are no known leaders yet, so the first sales will be trailbreakers.
- Avoid – These are areas that may sound promising but have some structural flaws that we thought would impede adoption.
Target
No surprises here. These are the sectors that already have referenceable success stories, and momentum is building:
- Financial Services
- Life Science
- Publishing, especially data publishing
- Manufacturing
Opportunistic
These are industries that should be ripe, but we haven’t seen much action yet. There are a few exceptions, for example NASA has done considerable work in this area. But most are puzzlingly slow. The insurance industry pretty much single handedly led the business rules movement, but have been largely absent so far in semantics, and data centric.
- Extractive industries (Oil & Gas, Mining, Agriculture)
- Construction
- Professional Services
- Transportation & Distribution
- Insurance
- Government
Avoid
Our reasoning here was as varied as it was wrong. Certainly, digital native companies (the incumbents like Google and Facebook as well as the hordes of VC funded startups) have the capacity and the knowledge to recognize the benefits of data-centricity and move forward on their own. We believed that many of them would have built their own in-house versions of what we were promoting.
We believed that systems integrators and application software vendors were too wed to their business models to really embrace data-centric.
We believe that healthcare delivery is overly dominated by a very few application software vendors (Epic and Cerner) and have difficulty escaping that center of gravity.
We believed hospitality (hotels and restaurants) retail and personal services were too decentralized and often at too small a scale to have the capacity to adopt something like this.
- Digital natives
- Systems integrators
- Application Software Vendors
- Healthcare Delivery
- Hospitality
- Retail
- Personal Services
Limitations of Our Observations
We’re a small firm. We don’t conduct large marketing campaigns. So, our conclusions may not be statistically valid.
But we do have enough data to discuss what we will call our false positives and false negatives (and their true variations as well).
For this purpose, if we nominate a firm or sector as a target (positive prediction) and land a successful project in that area (positive result), we would call that a true positive. A false positive occurs when we declare a sector to be a target, but fail to land any projects in thespace. A false negative occurs when we declare a sector to not be a target and they surprise us by becoming one. A true negative is when we don’t target an area and don’t land any projects in the area.
Because by definition we are not targeting those in our avoid category, we have almost no data or experience with true negatives. It’s all confirmation bias at this point.
It’s no surprise that most of our book of business is in the true positive category. We expected these to be good areas to fish and they were. We’re picking up a bit of interest in the opportunistic category, but its slow going.
Where we are learning something is in the other three areas: false positives, false negatives, and true negatives. Getting data on the latter was unexpected, given our attempt to avoid them.
What We Learned
So far, our major false positives have been in non-life science manufacturing. While there are a few referenceable use cases out there (Schneider-Electric, Ikea, and Procter & Gamble come to mind), the general uptake has been slower than we expected. Part of this may be that budgets are being consumed on large ERP system upgrade projects which are taking the oxygen out of the room. We’re still convinced this is a viable sector, but we may move it to the opportunistic category for now.
The more interesting false negatives, and some accidental true negatives, came from the avoid category.
We have had some successful, and fun, projects with smaller digital native firms. We still have not gotten any requests from what the investment community used to call the five horsemen: Facebook, Apple, Amazon, Netflix, and Google who they called FAANG, but now must be MAANA (Meta, Apple, Amazon, Netflix, and Alphabet). We haven’t gotten any inquiries from these mega firms and don’t really expect to, but the fact that there are start-ups looking for help with graphs is encouraging and wasn’t really expected. There seems to be a whisper campaign that the uptake of LLMs in the enterprise will depend on the enterprise organizing its data, seems to be nudging some startups toward Knowledge Graphs.
More surprising is the interest we’ve gotten from systems integrators and application software companies. The former is still very much on the fringe of their practices, and we’re convinced will not become mainstream offerings for large systems integrators (the economics just don’t work for them, one more SAP upgrade is worth far more than dozens of data-centric projects). But a couple of small systems integrators and SaaS vendors are coming to the conclusion that knowledge graphs may be part of their secret sauce.
Maybe the most interesting, and the motivation for the title of this paper, are the true negatives. We accidently got introduced to two senior executives. One who is the CIO of a company that manages hundreds of hotels; the other, the CIO of a restaurant chain with thousands of stores.
I never really thought much about hotel management, but there are four major players involved in the running of a hotel: the “brand” (Marriott, Four Seasons etc.), the third-party booking agencies (Expedia, Booking.com etc.), the owners (each property has separate owners, and often these are private equity firms), and the management firm (becoming more and more centralized).
Restaurants have similar diverse interests. Many are owned by franchisees. Some are managed by the franchisee; some are managed by professional management firms.
Here is where an interesting similarity and dissimilarity came up. I may have mentioned elsewhere in these white papers an observation I’ve come across. The number of applications a firm has implemented rises in proportion to its size. That by itself isn’t that surprising. What is surprising is the relative consistency of the ratio across a large range of firm sizes. Many firms, where we have access to the number of applications they have and the number of employees they have, have on the order of one app for every ten employees. That is, we expect a firm with 10,000 employees to be managing 1,000 apps.
A thousand apps mean 1,000 data silos and is a strong indicator of the need and the economic benefit of migrating toward data-centric.
Our “n of 2” (small sample size) in the hospitality industry had nearly the same ratio, but that’s where the similarity ended. A chain of restaurants may have 1,000 application systems, but they are all the same. A financial firm, or life science company or manufacturing plant with 1,000 applications has 1,000 different applications. Every schema in each of those systems is different. The restauranter has 1,000 point of sale systems, they might be all be identical, at most they have 2 or 3 variations.
The integration problem for a hospitality firm is just an aggregation problem. Bring in the data from the field and put it in one data warehouse. The schema for the data warehouse is no more complex than the schema for a single point of sale system. The lodging system turned out to be very similar. Hospitality companies have integration challenges. And they have a lot of third-party integration challenges. But they do most of their integration at the property and by the time they send the summaries to HQ the integration issues have been solved.
The hospitality industry became a true negative, but not for the reasons we originally thought. They really don’t need data-centric, because in a way they already are. (One single, simple model that can access all the data of the firm.)
We made an additional observation about major digital natives. Most have knowledge graphs at their core; this gives us the more complete answer to the question in the title: Companies that accidentally evolved to be data-centric don’t need to transform themselves.