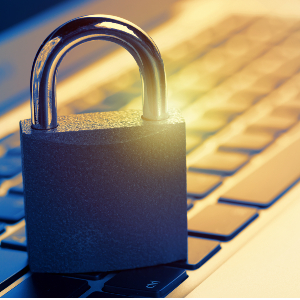
The road to creating business value through a well-oiled data management strategy can be long and challenging. A successful data management strategy is one that generates value rapidly and unlocks new data-driven insights. This is done by the mining of raw data into analytics– self-service analytics– that can be shared and used by various teams and consumers across the enterprise.
Self-service analytics, introduced over 30 years ago and iterated upon numerously, enabled data administrators, users, and consumers to move at the speed of the business to build and evolve new data products rapidly.
By removing the barriers to data access (e.g., alleviating the need to repeatedly ask data administrators for access to data related to projects), data users like data scientists and analysts can generate actionable insights from data to inform faster and better business decisions.
Self-service analytics come in many forms; most recently, COVID-19 forced companies to adapt to ever-changing customer preferences quickly. With the rapid access to critical data self-service analytics provides, data scientists are able to transform data to find new relationships worth exploring and justify the tools that empower them to connect different datasets with easier visualization.
The Rise of Data Governance
Coupled with rapid access to data, self-service analytics also encourage data sharing across businesses and data owners; however, this must be done with the proper security guardrails in place. A critical prerequisite to enabling secure self-service analytics is effective data governance. Data governance’s role is humanizing and connecting the data management journey to the business, as it aligns business goals and objectives by facilitating the finding, understanding, and accessing of trusted data.
Data Warehouses, the original solution to securing self-service analytics, acted as the “bundled,” centralized solution with built-in access control mechanisms. However, Big Data challenged the traditional norms of data management and gave rise to the adoption of cloud data technologies, or the modern data stack. Cloud gave businesses the advantages of elasticity, flexibility, and reduced operational/infrastructure costs, which allowed more “à la carte” access to flexible data solutions and enabled more capabilities. In turn, this “unbundling” enabled organizations to adapt to their unique requirements and use cases, become more agile, and build off of their ability to unlock new data insights.
The Rise of Unified Data Access Governance
As modern enterprises continue to break down data silos, add more cloud services to their data stacks, and add new data users, their environments become increasingly difficult to secure, posing many security and privacy challenges. To remove the complexity of managing multi-cloud enterprises with an ever-increasing volume of new data sources, applications, and users, Unified Data Access Governance (UDAG) must be the foundation of the data governance stack. UDAG brings more advanced security controls that address common problems like broken access control and implements proactive processes to enable rapid data access, while protecting its privacy, preserving self-service service analytics. UDAG also ensures that no matter where an enterprise is at on their data management journey, or how they evolve their data stacks, they can adapt and evolve.
UDAG is not a brand-new concept; it’s tested and proven. Apache Ranger was one of the first tools to tackle this in the golden age of Big Data. In on-premises data lakes, entire networks were contained in a single cluster. This evolved with the arrival of cloud; as the network cluster scaled, Apache Ranger evolved to meet the new requirements.