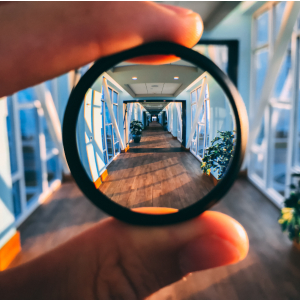
Synthetic Data is, according to Gartner and other industry oracles, “hot, hot, hot.” In fact, according to Gartner, “60 percent of the data used for the development of AI and analytics projects will be synthetically generated.”[1]
I had never heard about synthetic data until I listened to the AI Today podcast, hosted by Kathleen Welch and Ronald Schmelzer and their episode Overview of Synthetic Data.[2] Kathleen and Ron supply a useful overview of what this exotic sounding invention is all about – “computer-generated data that matches the range and quality of data needed to train systems.”
Their employer, Cognilytica, proclaims that, “The vendor landscape for Synthetic Data continues to expand with 76 vendors tracked in this snapshot, with a market size over $110M in 2021 growing to $1.15B by the end of 2027.”[3]
Truth be told, the first thing that sprang to mind when I heard about this ultra-hot, ultra-cool new trend was WandaVision, the Marvel series on Disney+ – so wonderful, right? Vision is a “synthezoid” and when I did my typical “word-nerdery” for this column, I was astonished to find that after a thorough online search (well, 10 minutes worth), synthezoid is a Marvel invention and does not have its roots in Greek or Latin.[4] How disappointing! However, “synthetic” does have an etymology worthy of note, traceable back to those ancient languages:
synthetic (adj.)
1690s, as a term in logic, “deductive,” from French synthétique (17c.) and directly from Modern Latin syntheticus, from Greek synthetikos “skilled in putting together, constructive,” from synthetos “put together, constructed, compounded,” past participle of syntithenai “to put together” (see synthesis). From 1874 in reference to products or materials made artificially by chemical synthesis; hence “artificial” (1930).[5]
Plenty of Greek, Latin and even French! It’s clear the purveyors of Synthetic Data are proclaiming their products may be “artificial” yet evidence their skill “in putting together” information, which is just as good, if not better, than the real thing.
After I got through my digression to the comics of my youth, my next reaction to synthetic data was skepticism, especially with regards to data bias. If synthetic data is designed to augment “ground-truth” data, then either it will preserve and even amplify the biases in the original data set, or it will reflect the biases of the synthetic data creators themselves. And indeed, bias is one of the limitations cited by IA Today and others.[6]
Neil Radan, in his article Synthetic data for AI modeling? I’m still not convinced,[7] cites The Advantages and Limitations of Synthetic Data by Marcello Benedetti[8]. After debunking most of the advantages Marcello identified, Radan reviews the limitations, which include:
- Outliers may be missing
- Quality of the model depends on the data source
- User acceptance is more challenging
- Synthetic data generation requires time and effort
- Output control is necessary
It was Radan’s comment regarding the first point which inspired this article: “outliers in the data can be more critical than regular data points, as Nassim Nicholas Taleb explains in-depth in his book, The Black Swan.” [9]
Ah, yes, Nassim Nicholas Taleb (who I will henceforth refer to as NTT, as he himself often does) and The Black Swan. Here was a book I had heard cited with overwhelming frequency during 2008 and 2009 when I worked first in one giant bank and then for another which swallowed the first, managing a corporate credit risk reporting team – talk about having a front row seat to the financial crisis! A Black Swan was the term everyone from bank executives to analysts used all the time– no doubt purposing to explain why they didn’t see the sub-prime mortgage/CDO debacle coming. (CDO = collateralized debt obligation, NOT chief data officer– an unfortunate coincidence of acronyms?). NNT himself who had written The Black Swan in 2007 and then added a novella-length essay to it in 2010, On Robustness and Fragility, didn’t think much of this attempt at spin doctoring:
“I will only very briefly discuss the crisis of 2008 (which took place after the publication of the book, and which was a lot of things, but not a Black Swan, only the result of fragility in systems built upon ignorance – and denial – of the notion of Black Swan events. You know with near certainty that a plane flown by an incompetent pilot will eventually crash).”[10]
Readers of my previous columns will be unsurprised that this chance reference to a book I knew about but had never read drove me to immediately get a copy from the local library (the first time I had checked out a book since before the pandemic). I was completely caught up by NNT’s brilliance and his sometimes whimsical, sometimes caustic style (especially his comments about economists and financial prognosticators). Particularly delightful is his full-throated admiration for one of my literary heroes, Michel De Montaigne, and Montaigne’s wonderful dictum – “I suspend judgement.”[11]
Speaking of the pandemic, NNT lives up to the blurb from GQ on the book cover “The most prophetic voice of all,” when he writes, in 2010, “I see the risk of a very strange acute virus spreading throughout the planet.”[12]
It’s how NNT brings his healthy skepticism – his suspension of judgement – to the way he views data which I think is expressly relevant to our consideration of the promises and pitfalls of synthetic data:
“So, we can learn a lot from data – but not as much as we expect. Sometimes a lot of data can be meaningless; at other times one single piece of information can be very meaningful. It is true that a thousand days cannot prove you right, but one day can prove you to be wrong.”[13]
As far as synthetic data’s ability to “create” outliers, artificial Black Swans, NNT warns us that “after a Black Swan, such as September 11, 2001, people expect it recur when in fact the odds of that happening have arguably been lowered. We like to think about specific and known Black Swans when in fact the very nature of randomness lies in its abstraction.”[14]
I do think synthetic data can have its place, to augment the ground-truth data from what NNT calls Mediocristan, the world of predictable ranges, like people’s height. Synthetic data generation can fill in the gaps for data sets like these. But where volatility is the rule (financial markets, for example), we are instead wandering through NNT’s Extremistan, where we need to approach any attempt to synthesize data describing that world with an excess of Montaigne-inspired suspension of judgement.
One of the most important lessons I took away from The
Black Swan is how critical it is to accept uncertainty and focus on putting
robust structures in place to manage the risks of those “unknown unknowns.”
Often, people assume as “ground-truth” that the more data you can feed into
your machine learning, your AI, your analytics, be it synthetic or real-life,
the more positive you can be of your results. But suspending judgement, a la Montaigne
and NNT, may mean that capturing ever greater quantities of data yields greater
uncertainty. If we acknowledge this and build robustness into our systems
and frameworks accordingly, then maybe, just maybe, this paradox is a good
thing.
[1] “Fake It to Make It: Companies Beef Up AI Models With Synthetic Data”, WSJ Pro, Artificial Intelligence, https://www.wsj.com/articles/fake-it-to-make-it-companies-beef-up-ai-models-with-synthetic-data-11627032601
[2] AI Today Podcast; Overview of Synthetic Data, https://www.cognilytica.com/2022/02/02/ai-today-podcast-overview-of-synthetic-data/
[3] Synthetic Data Generation Market: Research Snapshot Feb. 2022, Synthetic Data Generation Market: Research Snapshot Feb. 2022 – Cognilytica
[4]https://marvel.fandom.com/wiki/Glossary:Synthezoid#:~:text=Synthozoid%2C%20or%20Synthezoid%2C%20is%20a,a%20%22synthetic%20humanoid%22%20robot.
[5] Online Etymology Dictionary, https://www.etymonline.com/word/synthetic
[6] “ground-truth”: machine learning lingo for real-life, empirical data
[7] Radan, Neil, https://diginomica.com/synthetic-data-ai-modeling-im-still-not-convinced
[8] https://www.sama.com/blog/2018/01/24/the-advantages-and-limitations-of-synthetic-data
[9] Radan, Ibid.
[10] Taleb, Nassim Nicholas, The Black Swan, 2nd edition, 2010 Random House Trade Paperback Edition, pg. 321
[11] Taleb, Nassim Nicholas, Ibid, pg. 191.
[12] Taleb, Nassim Nicholas, Ibid, pg. 317.
[13] Taleb, Nassim Nicholas, Ibid, pp 56-57
[14] Taleb, Nassim Nicholas, Ibid, pg. 78