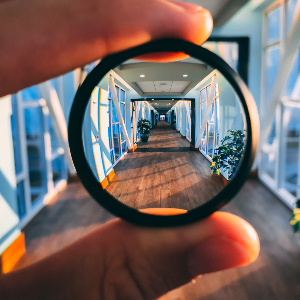
Back when I studied cello in college, I would seclude myself in a tiny practice room (we called them “cells,” a completely appropriate moniker) and spend many hours on “intonation”. In this context (remember that word!), the term means the degree to which the notes of a piece of music are played or sung exactly in tune.[1] Intonation was an obsession with me, as it was for most of my fellow cellists. A cello, like a violin or string bass, has no frets or other guides for the fingers. One has only the vaguest of visual clues as to where to place a finger to play a certain note. Only through careful listening and much practice does a string player achieve some level of success at playing in tune.
My teacher had me go through a piece of music note by note, hearing the next pitch in my head, and checking it against reference points like open strings or harmonics (where just touching a string at certain points results in a note). I’d walk into a lesson after a week of this work and feel that my intonation would be perfect. All would be well until my accompanist arrived to play the piano with me. Suddenly, I heard the unmistakable “beats” of out-of-tune notes, and frantically tried to adjust my finger placement. How I envied pianists who never had to worry about intonation at all – someone else tuned every note their instruments could play, and then all of us had to take those notes as gospel.
Next, I’d head to my string quartet rehearsal, to practice with three other tuning-fixated string players, and we all discovered the parts we had worked on so diligently separately didn’t mesh perfectly when played together. We’d all adjust, lowering a note here, raising another there, until we found the resulting combination of tones and harmony satisfying.
It was only recently that I came to understand how contextual intonation is. Oddly, what led this realization was Laura B. Madsen’s book, Disrupting Data Governance. Laura is the first person I’d read who defined data quality in terms of context:
“…intention is not what we should measure data quality against – it’s actually context. It’s a judgement of fitness of purpose that can and should be objective. But here’s the challenge, and it’s the same quandary we find ourselves in with data governance: how do we hit a moving target? Context and fit-for-purpose changes as the situations change. In a standard measurement situation, I would create a baseline and measure the current against it to get the delta. But if the baseline changes (our context), how can I objectively assess the delta?”[2]
Intuitively, this made sense to me, based on my work experience creating regulatory credit risk reports. In the context of one report, we would include some transactions as loans, but for other reports treat them as traded assets. The credit exposure metric itself could vary wildly between those reports which followed accounting rules versus credit risk metrics.
“Context” is a fascinating word. The Oxford English Dictionary (OED) cites the original, now obsolete, definition as: “The weaving together of words and sentences; construction of speech, literary composition.”[3] The word remained firmly planted in the realm of text and discourse for hundreds of years – “The whole structure of a connected passage regarded in its bearing upon any of the parts which constitute it; the parts which immediately precede or follow any particular passage or ‘text’ and determine its meaning”. Then in the 1800s, as the OED marvelously suggests, context became “transferred and figurative,” and usages such as “moral context”, “context of a building”, and “context of experience” emerged.
Recently, I’ve been listening to The Radical AI podcast, hosted by Jessie ‘Jess’ Smith and Dylan Doyle-Burke, featuring interviews with many of the thought leaders, scholars, and activists in the fields of AI ethics and responsible technology, from Ruha Benjamin and Kate Crawford to John C. Havens and Timnit Gebru. I can’t count how many times the words “context” and “contextual” came up in discussions on data bias, algorithmic justice, and understanding the impact of AI on all stakeholders.
Of course, the other area where this resonated with me was that old struggle to play in tune. I remembered that a big part of the challenge was that pianos are tuned using “equal temperament,” so that the musical “distance” between each white and black key is exactly the same. For better or worse, we string players have the freedom to adjust as the musical context calls for. For my solo playing, my teacher urged me to follow the example of the legendary cellist Pablo Casals, who played certain notes much closer together than they sound on the piano, to create more “gravitational attraction” to the next note.[4] David Blum, in his book Casals and the Art of Interpretation, summarizes Casals’ approach, “expressive intonation” in words which resonate with my thoughts on data and context:
“[Casals’] assertion that ‘each note is like a link in a chain – important in itself and also as a connection between what has been and what will be’, applied as equally to intonation as to other aspects of interpretation. The notes of a composition do not exist in isolation; the movement of harmonic progressions, melodic contours and expressive colorations provides each interval with a specific sense of belonging and/or direction. Consequently, Casals stressed that the equal-tempered scale with its fixed and equidistant semitones – as found on the piano – is a compromise with which string players need not comply.”[5]
Compelling! But I also remembered hearing something about “just temperament,” which aligned more to the natural “overtones” one hears generated when a cellist plays a low note sonorously.
I thought this concept might reveal novel insights into data quality, but I had never delved into the topic, until I found a book, How Equal Temperament Ruined Harmony (and Why You Should Care), by Ross W. Duffin.[6] Duffin does a terrific job of explaining the complexities of the evolution of tuning systems from the ancient Greeks through the Middle Ages right up to today, and he issues his own “call to action” which is all about context. Rather than clinging to equal temperament as the be-all end-all, he suggests we consider using a more “just” approach for music of Bach and Beethoven, allowing us to hear the music as they heard it. Even with music written since then, Duffin urges his readers to explore “harmonic intonation” when the context calls for it.[7]
So, what insights does this musical perspective on intonation and context yield about data quality?
- Despite how often we hear the importance of finding that “One Version of The Truth,” there is no one version of the truth when it comes to data. The quality of data is contextual, depending on the use case, usage, and purpose, just as whether one musician plays in tune with another depends on the context of which instruments they play and the intonation framework or frameworks they follow. An example of this is subscribing to multiple market price vendors because certain price datasets work for some countries or products and not for others. The “golden source” here is contextual.
- Quality measurements need to take context into account, calling for a degree of fluidity when defining rules and metrics. A rigid data completeness rule that mandates a specified field must always have a value falters when users add a new data set where this rule is no longer always valid. Only by assuring the rule is “living,” easily updated for changes in context, can we sustain effective quality measurements.
- The difficulty in preparing a data set in isolation for use with a certain context is, well, devilishly difficult. It’s akin to me spending all those hours in a practice cell and then finding that music which I thought I could play perfectly in tune sounded jarring when combined with whomever I needed to play it with. Years ago, I remember spending months looking for data anomalies in preparation for a migration but didn’t apply all the rules the system would apply. We went live with few production issues, but one we did run into was that a particular contract type the SMEs said was obsolete was still in use within one country, making it impossible to update those contracts in the new tool. You can’t profile a data set in a vacuum – you must look at multiple scenarios to find flaws which will reveal themselves contextually.
In closing, accepting that you can’t categorize a data set
as perfectly accurate/complete/consistent, or not, and that data quality is
contextual, can be hard to swallow. But trust me, there’s nothing worse than
stubbornly sticking with one’s own interpretation of what’s in tune and
ignoring the fact that you are out of tune with everyone else. By taking
context into account, we can tune the data to truly be fit for purpose.
[1] Cambridge Dictionary, https://dictionary.cambridge.org/us/dictionary/english/intonation
[2] Madsen, Laura B., Disrupting Data Governance, 2019, Technics Publications, pp. 123-124
[3] OED Third Edition, December 2013; most recently modified version published online September 2021
[4] Blum, David, Casals and the Art of Interpretation, 1977, University of California Press, pg. 103
[5] Ibid, pg.102
[6] Duffin, Ross W., How Equal Temperament Ruined Harmony (and Why You Should Care), 2007, W. W. Norton & Company
[7] Ibid, pg. 156