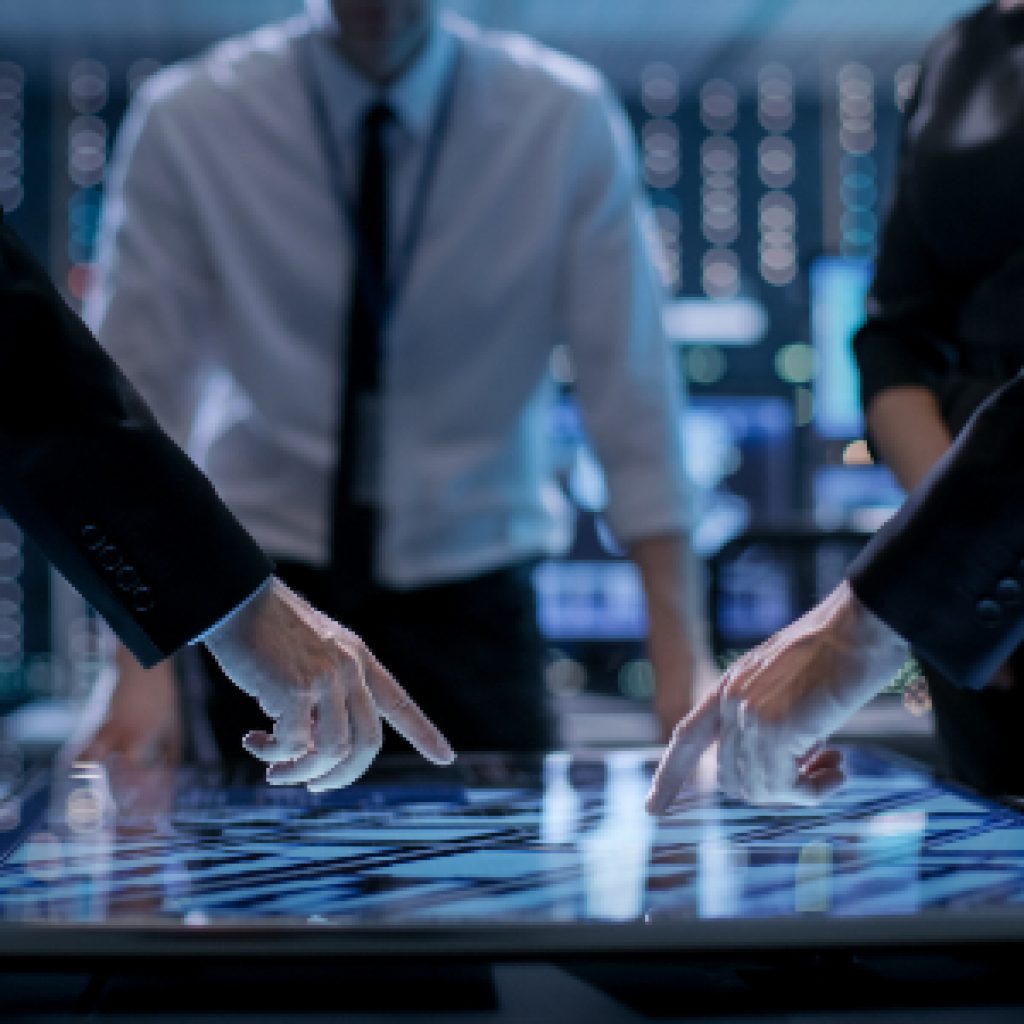
Big data has been one of the most discussed topics of the last few years. However, this term has been around for a while. Since the term “big data” was introduced in the 1940s, technologies have developed and now we live in a world that depends on data. We produce data all the time, and all areas of our lives depend on apps and devices that process data. The global data market has already reached $26 billion, and companies spend more money on big data every year.
Thanks to machine learning and artificial intelligence, companies can use data of any volumes. All the data collected from mobile devices, sensors, and GPS is no longer just information. It’s a source of priceless insights into people’s behavior and preferences. This way, data helps businesses target the right audience, offering the right products to the right people. However, many people get surprised when they realize how many things big data cannot do. Even though there are many possible applications of big data, it isn’t a universal solution for all the problems organizations face.
We decided to help you understand what big data is and isn’t capable of. Let’s take a look at the five things big data can do, and the things it cannot do so that you can manage your expectations.
Things Big Data Can Do
- Predictive analysis
This is one of the most common tasks. Machine learning created numerous opportunities for predictive analysis. Machines can analyze historical data, detect patterns, and predict the probability of certain events occurring in the future. For example, if you own a chain of restaurants all over the world, you can predict which restaurants are likely to get fewer customers than expected. Therefore, you can focus on these restaurants and prevent this problem before it happens. - Diagnostic analysis
Whenever some problems happen, companies need to know what their causes are. This is what machines are really good at. The analysis of patterns in data helps determine correlations between different events so that you can avoid similar situations in the future. - Finding relations between unknown elements
Big data also enables organizations to find relations between virtually any events or elements, even if it seems like they have nothing to do with each other. How can it be useful? For example, you can find out that your sales are not related to the changes in the number of salespeople. In this case, you may want to reduce the number of employees if it won’t affect other areas of business. - Monitoring events
Monitoring events in real-time is also a task that requires computers to process a lot of data. They also need to do it quickly. Thanks to big data, we can monitor any events. For example, marketers can see how different segments of their audience react to a campaign. - Prescriptive analysis
This is a very promising area of analytics that quickly develops. The prescriptive analysis is based on predictive analysis. However, this approach enables organizations to not only predict certain events but also to find the most effective solutions for each possible scenario. Predictive analysis is used in different areas, For instance, this approach can be used to fight terrorism.
Things Big Data Cannot Do
- Imputation of new data sources
The evaluation of data sources and replacing missing data with substituted values takes a lot of time, no matter what kind of analysis you need to perform. Even though machines can now process data and provide insights from it, people still have to deal with one of the most boring tasks associated with the analysis of data because it requires business understanding and creativity. - Predicting a definitive future
The most sophisticated machine learning algorithms can formulate predictions with high accuracy. However, they are still far from reaching 100% accuracy. No matter how accurate your tools are, they won’t be able to provide you with solutions that could help you achieve a 100% response rate by targeting a certain segment of the audience. - Dealing with creative tasks
Although machines become smarter every day, they still cannot work on creative tasks. For example, chatbots can answer questions in a conversational manner but they don’t understand the context of the conversation, and neither can they understand sarcasm or jokes. Therefore, if you need good content for your website, you cannot simply use AI to write it. Businesses still have to look for talented writers to create their copy and other types of content. - Data management
The more data we have, the more difficult it becomes to manage it. We have different types of data and different types of data structures. If you need to simplify data for a new data source, a computer won’t be able to deal with this task. For instance, if you have graph data, it might be useless for campaign data and yet very useful for network analysis. Machines won’t be able to analyze this type of data so you will have to manage and process it manually, changing it so that machines can work with it. - Solving problems that are not well-defined
One of the main problems of analytics is turning business problems into analytics problems. Machines can only work with well-defined tasks because they lack an understanding of business processes. Computers can analyze data and detect patterns in it but they don’t really understand what this data represents. Therefore, businesses still have to rely on human employees who can formulate tasks for computers. For example, if you want to manage attrition, you have to define time windows, responders, etc., because otherwise, predictive algorithms won’t be able to help you.
Conclusion
We live in an era of big data. All areas of our lives depend on data and we produce tons of data all the time. Thanks to technologies like artificial intelligence and machine learning, computers can analyze any volumes of data, providing us with actionable insights. However, machines still have certain limitations so everyone should understand what machines can and cannot do. For example, machines still lack creativity and they don’t understand the meaning of data they’re working with. Therefore, even the most sophisticated algorithms still need help from humans.