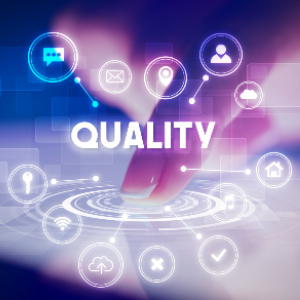
Fortune 1000 organizations spend approximately $5 billion in total each year to improve the trustworthiness of data. Yet, only 42% of the executives trust their data. According to multiple surveys, executives across industries do not completely trust the data in their organization for accurate, timely business-critical decision-making. In addition, organizations routinely incur operational losses, regulatory fines, and reputational damages because of data quality errors. Per Gartner, companies lose on average $13 million per year because of poor data quality. [1]
These are startling facts – given that most Fortune 500 organizations have been investing heavily in people, processes, best practices, and software to improve the quality of the data.
Despite heroic efforts by data quality teams, data quality programs simply failed to deliver a meaningful return on investments.
This failure can be attributed to the following factors:
1) Iceberg Syndrome: In our experience, data quality programs in most organizations focus on what they can easily see as data risk based on past experiences which is only the tip of the iceberg. Completeness, integrity, duplicate, and range checks are the most common type of checks that are implemented. While these checks help in detecting data errors— they represent only 30-40% of the data risk universe. WAIT, WHAT?! The other 70-60% goes undetected and surfaces as data errors to consumers. [2]
2) Data Deluge: The number of data sources, data processes, and applications have increased exponentially in recent times due to the rapid adoption of cloud technology, big data application, and analytics. Each of these data assets and process requires adequate data quality control to prevent data errors in the downstream processes.
While the data engineering teams can onboard hundreds of data assets in weeks, data quality teams usually take between one to two weeks to establish a data quality check for a data asset. As a result, data quality teams prioritize data assets for data quality rule implementation leaving many data assets without any type of data quality controls.
3) Legacy Processes: Data quality teams often go through a lengthy process of change requests, impact analysis, testing, and signoffs before implementing a data quality rule. This process can take weeks or even months, during which time the data may have significantly changed.
There is a need for a more effective and scalable way to deal with data quality. Current methods are not working well and they are not sustainable. One possible solution is to adopt Data Trustability.
What is Data Trustability?
How can it address the scalability challenges of data quality programs? Data Trustability is the process of finding errors using unsupervised machine learning, instead of relying on human-defined business rules.[3] This allows data teams to work more quickly and efficiently. Data Trustability leverages machine learning algorithms to construct data fingerprints for each dataset. Deviation from the data fingerprints is identified as data errors.
More specifically, the Data Trustability finds the following types of data quality issues without any user input:
- Dirty Data: Data with invalid values, such as incorrect zip codes, missing phone numbers, etc.
- Completeness: incomplete Data, such as customers without addresses or order lines without product IDs.
- Consistency: inconsistent Data, such as records with different formats for dates or numerical values.
- Uniqueness: Records that are duplicates
- Anomaly: Records with anomalous values of critical columns
There are two benefits of using data trustability. The first is that it doesn’t require human intervention to write rules. This means that you can have a lot of data risk coverage without significant human effort. The second benefit is that it can be deployed at multiple points throughout the data journey. This gives data stewards and data engineers the ability to scale and react early on to problems with the data.[4]
Data quality programs will continue to exist and they will be designed to meet specific compliance requirements. Data Trustability can help you achieve high data quality and observability in your data architecture.
Data is the most valuable asset for modern organizations. Current approaches for validating data, in particular Data Lake and Data Warehouses, are full of operational challenges leading to trust deficiency, and time-consuming, and costly methods for fixing data errors. There is an urgent need to adopt a standardized ML-based approach for validating the data to prevent data warehouses from becoming a data swamp.
References
[1] https://www.gartner.com/smarterwithgartner/how-to-improve-your-data-quality
[2] https://firsteigen.com/blog/why-do-data-quality-programs-fail/
[3] https://tdan.com/data-managements-next-frontier-is-machine-learning-based-data-quality/29282