There are plenty of reasons you might want to use proven “universal” data model patterns to improve the outcome of your enterprise initiative or your agile project – the patterns are proven, robust, efficient now, and flexible for the future.
There are also many reasons for considering the Data Vault (DV) architecture for your data warehouse or operational data integration initiative – auditability, scalability, resilience, and adaptability.
But if your enterprise wants the benefits of both, does their combination (as a “Universal Data Vault”) cancel out the advantages of each? A case study looks at why a business might want to try this approach, followed by a brief technical description of how this might be achieved.
Country Endeavours Pty Ltd
“Creative solutions for Difficult Problems”
Why Would Anyone Want a “Universal Data Vault”?
Patterns at Work
The idea of using proven data model patterns to deliver business benefits isn’t new. In his 1995 book, Data Model Patterns: Conventions of Thought, David Hay stated, “Using simpler and more generic models, we will find they stand the test of time better, are cheaper to implement and maintain, and often cater to changes in the business not known about initially.”
That’s the theory, and I’m pleased to say it works. I smile when I remember one interview for a consulting job. The interviewer reminded me that another telecommunications company we both knew had spent years developing an enterprise logical data model (ELDM). He then threw me a challenge, saying, “John, I want you to create the same sort of deliverable, on your own, in two weeks – we’ve got three projects about to kick off, and we need an ELDM to lay the foundations for data integration across all three.”
I cut a deal. I said that if I could use the telecommunications industry data model pattern from one of Len Silverston’s data model patterns books, modified minimally based on time-boxed interviews with the company’s best and brightest, I could deliver a fit-for-purpose model that could be extended and refined over a few additional weeks. And I did.
I’ve done this sort of thing many times now. I commonly use “patterns”, but it’s the way they’re applied that varies. Sometimes an ELDM is used to shape an Enterprise Data Warehouse (EDW). Other times it is used to define the XML schema for an enterprise service bus (ESB). Or maybe mold a master data management (MDM) strategy, or define the vocabulary for a business rules engine, or provide a benchmark for evaluation of the data architecture of a commercial-off-the-shelf package…
There’s a key message here. If you use a pattern-based ELDM for any of these initiatives, the job of information integration across them is much easier, as they are founded on a common information model. This is exactly what an IT manager at another of my clients wanted. But his challenges were daunting.
Some of you will recognize the data administration headaches when one company acquires another, and the two IT departments have to merge. It can be painful. Now try to imagine the following. As of June 30th, 2010, there were 83 autonomous, but related, organizations across Australia, each with something like 5 fundamental IT systems, and each of these systems had maybe 20 central entities. If they were all in relational databases, that might add up to something like 8,000 core tables. Then on July 1st, under orders of our national government, they become one organization. How do you merge 8,000 tables?
They had some warning that the organizational merger was going to happen, and they did amazingly well, given the complexity, but after the dust settled, they wanted to clean up one or two (or more!) loose ends.
Firstly, I created an ELDM (pattern-based, of course) as the foundation for data integration. It’s a bit of a story in its own right, but I ran a one-day “patterns” course for a combined team of business representatives and IT specialists, and the very next day, I facilitated their creation of a framework for an ELDM. It did take a few weeks for me to flesh out the technical details, but the important outcome was business ownership of the model. It reflected their concepts, albeit expressed as specializations of generic patterns. This agreed expression of core business concepts was vital, as we will shortly see.
A Data Vault Solution Looks Promising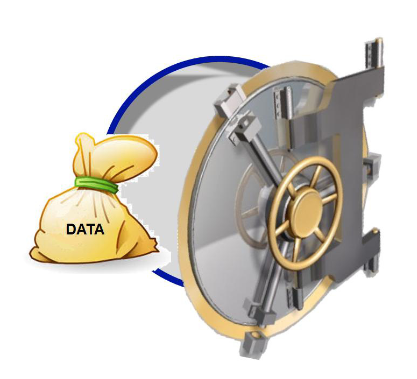
The second major activity was the creation of an IT strategy that considered Master Data Management, Reference Data Management, an Enterprise Service Bus and, of course, Enterprise Data Warehouse components. The major issue identified was the need to consolidate the thousands of entities of historical data, sourced from the 83 disparate organizations. Further, the resulting data store needed to not only support Business Intelligence (BI) reporting, but also operational queries. If a “client” of this new, nationalized organization had a history recorded in several of the 83 legacy organizations, one consistent “single-view” was required to support day-to-day operations. An EDW with a services layer on top was nominated as part of the solution.
The organzation had started out with a few Kimball-like dimensional databases. They worked well for a while, but then some cross-domain issues started to appear, and work commenced on an Inmon-like EDW. For the strategic extension to the EDW, I suggested a Data Vault (DV) approach. This article isn’t intended to provide an authoritative description of DV – there’s a number of great books on the topic (with a new reference book by Dan Linstedt hopefully on its way soon), and the TDAN.com website has lots of great material. It is sufficient to say that the some of the arguments for DV were very attractive. This organization needed:
-
Auditability: If the DV was to contain data from hundreds of IT systems across the 83 legacy organizations, it was vital that the source of the EDW’s content was known.
-
Flexibility/Adaptability to change: The EDW had to be sufficiently flexible to accommodate the multiple data structures of its predecessor organizations. Further, even during the first few years of operation, this new organization kept having its scope of responsibility extended, resulting in even more demand for change.
I presented a DV design, and I was caught a bit off guard by a comment from one of the senior BI developers who said, “John, what’s the catch?” He then reminded me of the saying that if something looks too good to be true, it probably is! He was skeptical of the DV claims. Later, he was delighted to find DV really did live up to its promises.
But there was one more major challenge.
A Marriage of Convenience? The Generalization/Specialization Dilemma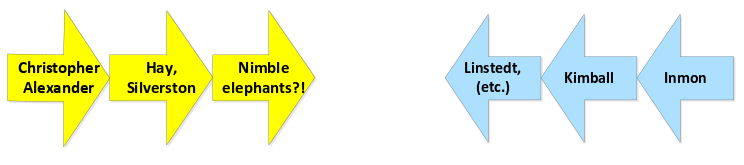
Data model patterns are, by their very nature, generic. Len Silverston produced a series of 3 books on data model patterns (with Paul Agnew joining him for the third). Each volume has the theme of “universal” patterns in their titles. Len argues that you can walk into any organization in the world, and there is a reasonable chance they will have at least some of the common building blocks such as product, employee, customer, and the like. Because the patterns are deliberately generalized, their core constructs can often be applied, with minor tuning, to many situations. In Volume 3, Len & Paul note that data model patterns can vary from more specialized through to more generalized forms of any one pattern, but that while the more specialized forms can be helpful for communication with non-technical people, the more generalized forms are typically what is implemented in IT solutions. The bottom line? We can assume pattern implementation is quite generalized.
Conversely, DV practitioners often recommend that the DV Hubs, by default, avoid higher levels of generalization. There are many good reasons for this position. When you talk to people in an enterprise, you will likely discover that their choice of words to describe the business appears on a continuum, from highly generalized to very specialized. As an example, I did some work many years ago with an organization responsible for emergency response to wildfires, floods, and other natural disasters. Some spoke sweepingly of “deployable resources” – a very generalized concept. Others were a bit more specific, talking of fire trucks and aircraft, for example. Yet others spoke of “water tankers” (heavy fire trucks) and “slip-ons” (portable pump units temporarily attached to a lighter 4WD vehicle) rather than fire trucks. Likewise, some spoke of “fixed-wing planes” and “helicopters” rather than just referring to aircraft.
In practice, if much of the time an enterprise uses language that reflects just one point on the continuum, life’s easier; the DV implementation can pick this sweet spot, and implement these relatively specialized business concepts as Hubs. This may be your reality, too – if so, you can be thankful. But within my recent client’s enterprise involving 83 legacy organizations, there was no single “sweet spot”; like with the wildfire example, different groups wanted data represented at their sweet spot!
Here was the challenge. By default, data model patterns, as implemented in IT solutions are generalized, while DV Hub designs are, by default, likely to represent a more specialized view of the business world. Yet my client mandated that:
-
The DV implementation, while initially scoped for a small cross-section of the enterprise, had to have the flexibility to progressively accommodate back loading the history from all 83 legacy organizations. As a principle, any DV implementation should seek to create Hubs that are not simplistic, mindless transformations from the source system’s tables. Rather, each Hub should represent a business concept, and any one Hub may be sourced from many tables across many systems. So even though the combined source systems contained an estimated 8,000 tables, we did not expect we would need 8,000 Hubs. Nonetheless, if we went for Hubs representing somewhat specialized business concepts, we feared we might still end up with hundreds of Hubs. There was a need for a concise, elegant, but flexible data structure capable of accepting back loading of history from disparate systems. I met this need by designing a DV solution where the Hubs were based on “universal” data model patterns.
-
The DV implementation of the new EDW had to align with other enterprise initiatives (the enterprise service bus and its XML schema, MDM and RDM strategies, and an intended business rules engine). All of these, including the DV EDW, were mandated to be consistent with the one generalized, pattern-based ELDM. Interestingly, the business had shaped the original ELDM framework, and it was quite generalized, but with a continuum of specialization as well. I needed to create a DV solution that could reflect not just more generalized entities, but this continuum.
I called the resultant solution a “Universal Data Vault”. This name reflects my gratitude to the data model pattern authors such as Len Silverston (with his series on “universal” data model patterns) and David Hay (who started the data model patterns movement, and whose first book had a cheeky “universal data model” based on thing and thing type!), and Dan Linstedt’s Data Vault architecture.
We have now considered why I combined generalized data model patterns with Data Vault to gain significant business benefits. Next, we look at how we design and build a “Universal Data Vault”, from a more technical data model perspective.
Introducing the “Universal Data Vault” (UDV)
Foundations: A Brief Review of Data Vault – Hubs, Links and Satellites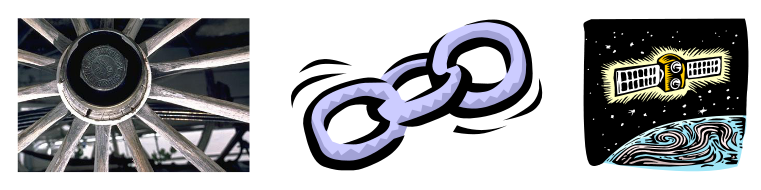
If we go back to the emergency response example cited earlier, and prepare a data model for one tiny part of their operational systems, we might end up with a data model something like the following:Here we see aircraft (helicopters or a fixed wing planes) being assigned to planned schedules-of-action for emergency responses (fires, floods, earthquakes, etc.). This model reflects an operational point-in-time view: at any one point in time, an aircraft can be assigned to only one emergency. Of course, over time, it can be assigned to many emergencies. If we now design a database to hold the history, and if we choose to use Data Vault (DV) architecture, we could end up with something like this:
Without going into details of the transformation, we can note:
-
Each business concept (an aircraft, a schedule) becomes a Hub, with a business key (e.g. the aircraft’s registration number), plus a surrogate key that is totally internal to the DV. Each Hub also records the date & time when the DV first discovered the business instance (e.g. an aircraft registered as ABC-123), and the operational system that sourced the data.
-
Each relationship between business concepts (e.g. the assignment of an aircraft to an emergency) becomes a Link, with foreign keys pointing to the participating Hubs. Like a Hub, the Link also has its own internal surrogate key, a date & time noting when the relationship was first visible in the DV, and the source of this data. Note that while an operational system may restrict a relationship to being one-to-many, the Links allow many-to-many. In this case, this is essential to be able to record the history of aircraft assignment to many emergencies over time.
-
Hubs (and Links – though not shown here) can have Satellites to record a snapshot of data values at a point in time. For example, the emergency schedule Hub is likely to have changes over time to its severity classification, and likewise, its responsible incident controller.
Fundamentals of the UDV “How-to” Solution
As noted in the previous part of this paper, people may challenge the level of generalization/specialization of the business concepts that become Hubs. While some may be comfortable with the business concept of an “aircraft”, others may want separate, specialized Hubs for Helicopters and Fixed Wing Planes, and yet others may want to go the other way – to combine aircraft with fire trucks and have a generalized Hub for Deployable Resources.
One solution is to have a Hub for the generalized concept of a Deployable Resource, as well has Hubs for Aircraft (and Fixed Wing Plane and Helicopter), Fire Truck (and Water Tanker and Slip-On), Plant (Generator, Water Pump) and so on. The inheritance hierarchies between the generalized and specialized Hubs can be recorded using “Same-As” Links. This approach is most definitely something you should consider, and is explained in a number of publications on Data Vault. However, for this particular client, there was a concern that this approach may have resulted in hundreds of Hubs.
I created the “Universal Data Vault” (UDV) approach as an alternative. A sample UDV design follows. At first glance, it may appear to be a bit more complicated than the previous diagram. However, the generalized Asset Hub not only represents the incorporation of Aircraft, but additionally it can accommodate a large number of specialized resource Hubs for Fire Trucks, Plant & Equipment and so on. Likewise, the generalized Activity Hub not only handles the Emergency Response Schedule Hub, but also other specialized Hubs such as activities for preventative planned burns.
In the next part of this paper, we will look in more detail at the individual UDV components.