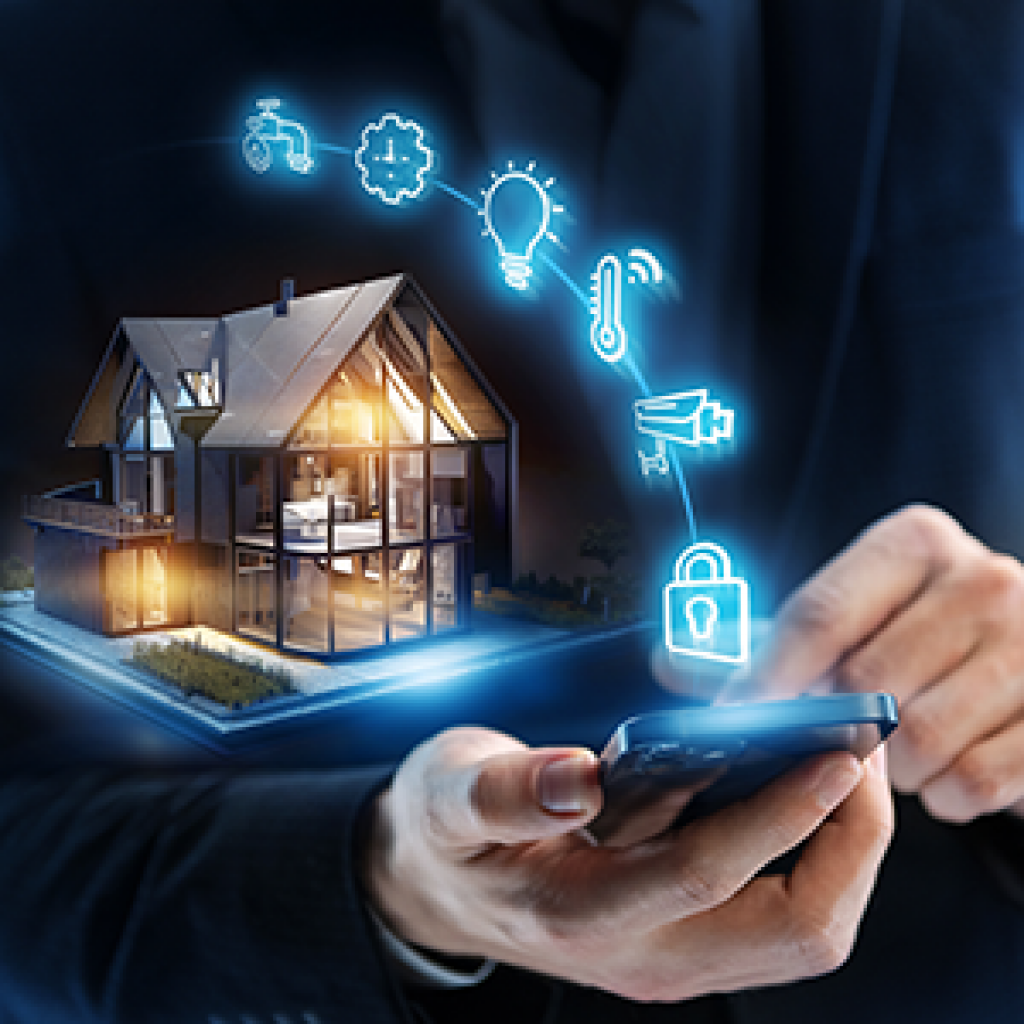
What Is IoT Data Management?
IoT data management refers to the process of collecting, storing, processing, and analyzing the massive amounts of data generated by Internet of Things (IoT) devices. These devices, which can range from sensors and cameras to smart appliances and industrial machines, generate continuous streams of data that need to be managed efficiently to derive valuable insights.
IoT data management encompasses several key aspects, including data ingestion from diverse sources, real-time processing, secure storage, and efficient retrieval. It also involves implementing strategies for ensuring data quality, security, privacy, and compliance with relevant regulations. As IoT ecosystems expand, managing this data becomes critical to enabling real-time decision-making and optimizing device operations across industries.
Importance of Managing IoT Data
Security and Privacy
Security in IoT data management involves protecting data from unauthorized access and breaches. This requires encryption, authentication protocols, and continuous monitoring. IoT devices are often targeted due to their connectivity and data sensitivity, making security essential.
Privacy is another concern, as IoT devices often collect personal data. Ensuring privacy involves data anonymization and compliance with legal standards. Users must feel confident that their data is protected, necessitating transparent data handling policies and practices.
Compliance with Regulations
IoT data management must comply with industry regulations like GDPR and HIPAA, which govern data protection and privacy standards. Non-compliance can result in heavy fines and loss of customer trust. Organizations need to implement policies that align with these regulations to secure and process data responsibly.
Ensuring compliance involves regular audits and updates to data management practices. Organizations must stay informed about changing laws and regulations to maintain compliance. This requires dedicated resources and expertise in legal and data management domains.
Data Quality and Accuracy
High data quality is crucial for accurate analysis and decision-making. Poor data quality can lead to incorrect insights, affecting business operations. Ensuring data accuracy involves validating data at collection points and adopting standardized data formats.
Data accuracy is enhanced by implementing real-time monitoring and cleansing mechanisms. These measures help detect and resolve inconsistencies promptly, maintaining data integrity. Consistent data quality ensures reliable outcomes in analytics and business processes.
10 Risks of IoT Data Management and How to Solve Them
1. Data Security Risks
IoT data management faces significant security risks due to the large attack surface created by interconnected devices. Each device presents a potential entry point for cyberattacks, including data breaches and malware injections. Attackers may exploit vulnerabilities in device firmware, weak authentication methods, or unsecured network protocols.
To mitigate these risks, implementing end-to-end encryption, device authentication, and secure communication channels is essential. Regular firmware updates, patch management, and network segmentation can further safeguard IoT ecosystems. Additionally, monitoring and anomaly detection tools help identify and respond to potential security threats in real time.
2. Privacy Concerns
IoT devices often collect sensitive personal information, which raises concerns about user privacy. The lack of transparency in how data is collected, processed, and shared can erode user trust, especially when data is shared with third parties without explicit consent.
Addressing privacy concerns requires the anonymization and pseudonymization of personal data. Adopting privacy-by-design principles, such as embedding privacy features in the early stages of device development, can ensure that privacy protection is an integral part of the system. Clear user consent policies and adherence to privacy regulations, like GDPR, are also necessary to safeguard personal information.
3. Data Overload and Storage Management
The massive influx of data generated by IoT devices can overwhelm traditional storage systems, leading to data overload. Managing this data efficiently is a challenge, as continuous data generation requires significant storage capacity and processing power.
To solve this, organizations can adopt edge computing, which processes data closer to the source, reducing the need for centralized storage. Implementing data compression techniques, data lifecycle management, and tiered storage solutions can also help balance storage costs while maintaining performance. Storing only relevant or summarized data can further optimize storage resources.
4. Inconsistent Data Quality
Inconsistent data quality is a common problem in IoT data management, as data is often collected from diverse devices with varying standards and formats. This inconsistency can lead to unreliable insights, affecting decision-making processes.
Ensuring consistent data quality requires standardizing data formats and validation protocols at the collection points. Automated data cleansing tools can help detect and resolve errors, such as duplicate or incomplete data, in real time. Establishing data governance frameworks that define quality standards and processes for maintaining data integrity is also essential for improving data reliability.
5. Latency and Data Transmission Delays
IoT applications often require real-time data processing, but network latency and transmission delays can hinder performance. High latency can lead to delayed responses in time-sensitive applications, such as autonomous vehicles or industrial control systems.
To minimize latency, edge computing can be used to process data locally, reducing the need for long-distance transmission. Additionally, utilizing faster network technologies like 5G, optimizing data packet sizes, and prioritizing critical data over less important traffic can improve overall system responsiveness.
6. Integration Challenges
IoT ecosystems often consist of a wide variety of devices, platforms, and communication protocols, which can lead to integration challenges. Devices from different manufacturers may use incompatible standards, making it difficult to aggregate and process data across the system.
Solving integration challenges requires adopting industry standards and open protocols, such as MQTT or CoAP, to ensure device interoperability. Middleware platforms that can bridge gaps between disparate systems are also essential. Organizations should consider designing flexible architectures that can easily integrate new devices and technologies as they evolve.
7. Data Governance and Compliance
Ensuring that IoT data management aligns with governance and compliance requirements is a complex task. IoT devices often operate across multiple jurisdictions, each with their own data protection laws, making it difficult to maintain compliance.
To address this, organizations must implement robust data governance frameworks that establish clear policies for data ownership, access control, and audit trails. Regular compliance audits and continuous monitoring of legal updates are necessary to stay aligned with evolving regulations. Appointing data protection officers (DPOs) or legal teams focused on IoT-specific compliance can further ensure regulatory adherence.
8. Data Ownership and Control
The question of data ownership in IoT environments is often unclear, as data may be generated by devices owned by users, but processed and stored by service providers. This ambiguity can lead to conflicts over who has control over the data and how it can be used.
Organizations need to establish clear agreements with users regarding data ownership and access rights. Transparent data policies, user consent mechanisms, and legal contracts that outline data usage can help resolve conflicts. Implementing solutions that give users greater control over their data, such as data portability features, can also enhance trust and compliance.
9. Scalability Issues
As IoT deployments grow, managing the increasing number of devices and data streams can present scalability challenges. Traditional data management infrastructures may struggle to scale efficiently, leading to performance bottlenecks.
To address scalability issues, cloud-based platforms that offer elastic scaling can provide the flexibility needed to handle growing data volumes. Leveraging distributed architectures, like microservices and serverless computing, allows systems to scale dynamically without significant infrastructure changes. Efficient resource management and load balancing across the system are also critical to maintaining performance as IoT networks expand.
10. Power and Connectivity Limitations
Many IoT devices, especially those in remote or harsh environments, face power and connectivity constraints. Devices with limited battery life or unstable network connections may struggle to transmit data reliably, affecting system performance.
Low-power communication protocols, such as LoRaWAN or NB-IoT, can help extend battery life and maintain connectivity over long distances. Devices should be designed with energy-efficient components, and power-saving strategies, such as sleep modes, to further reduce consumption. Additionally, deploying edge devices that can store and process data locally during connectivity outages can improve reliability in intermittent network environments.
Conclusion
IoT data management ensures security, compliance, and quality while mitigating associated risks. Addressing challenges systematically secures data integrity and enhances operational efficiency, supporting business objectives.
Organizations must continually adapt to regulatory changes, technological advancements, and increased data volumes. Strategic planning and implementation of best practices are crucial to achieving robust IoT data management and deriving maximum value from IoT systems.