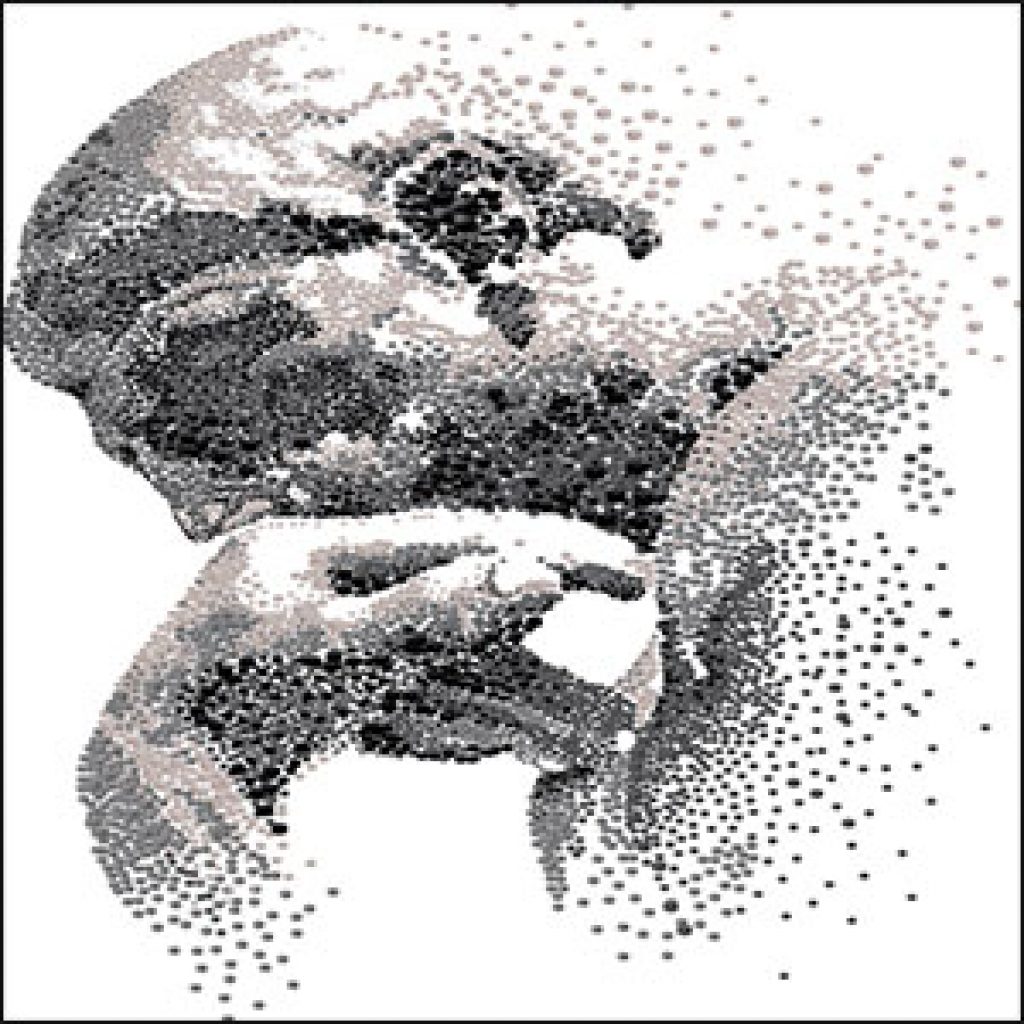
I’ve often encountered client staff, course students, and conference attendees who are grappling with the basic question:
“What is the difference
between Data Management
and Data Governance?”
It seems that the more our industry expands, the more frequently this question is asked.
———-
I attribute this to a few factors:
- The increasing volume of data management content produced and published, expressing varying points of view
- Differences in perspective that are influenced by vendors
- Varying data management frameworks applied
- Increasing specialization among industry experts
Another reason for the confusion is that both functions must operate together to implement solutions. That natural occurrence lends itself to viewing the nature of the relationship through a project-oriented lens. I think our industry needs to clarify the description of these functions, because that’s what they are, ongoing interdependent functions that an organization always needs to perform. In fact, as I’m sure we agree, all organizations would be in much better shape (data-wise) if they had recognized these complementary functions and strengthened them decades ago.
So let’s engage in some reflection about these terms. This column addresses some foundational concepts, and then offers definitions and corresponding diagrams that may help to clarify what Data Management is, what Data Governance is, and how they interact.[i]
We’ll start right at the top, with disambiguation of two umbrella terms that are often used interchangeably. The first is Enterprise Data Management per se, and this is the brief working description that I’ve used for many years:
- The goal of Enterprise Data Management (EDM) is to increase trust and confidence in corporate data assets and support progressive evolution towards the target state. It is a permanent function encompassing multiple integrated disciplines, constituting the capabilities of an organization to:
- Precisely define, easily integrate and effectively retrieve and share data for both internal use and external communication.
- Publish and promote policies, processes, standards and governance practices which are conducive to improving data quality, integration, optimization, and standardization of the enterprise data layer.
Unpacking that a bit, note that the first sentence describes overall strategic goals, and the second sentence, with bullets, is the working definition. Why worry about the data at all, and why do anything about it? To increase trust and confidence in the data assets, used for every decision. Why mention the ‘target state’ as a goal? Because the existing data architecture in virtually every organization is highly complex – unless that ever-increasing complexity is addressed, the organization will incur greater inefficiencies and expenditure year after year, continually cutting into the percentage of discretionary funding for innovation and new data technologies.[1]
The key phrase in the second sentence is ‘permanent function.’ This cannot be overemphasized. The failure to establish data management as a permanent enterprise function has been the most challenging issue in our industry for decades. Just as an organization has always had, and always will need, critical supporting functions such as Finance, Human Resources, and Facilities Management, it will always need EDM. Since data is a foundational component of an organization’s infrastructure, it should be understood and managed accordingly – and managed forever, because the data is forever. Many organizations I’ve worked with had not previously accepted this concept, e.g., they had been funding all data-related initiatives within the context of specific projects and specific applications. Projects, as we know, have a beginning, a middle, and an end (emphasis on ‘end’). When the project funding ends, any data progress that was accomplished has no forward path or planned designated resources to standardize or advocate for reuse across the organization.
‘Multiple integrated disciplines’ refers to the core capabilities we advise organizations to establish, strengthen, and follow, including managing business terms, metadata, data quality, data lineage, data requirements, etc. The disciplines are conceptually separable data management topics, or process areas, such as those described in the DMBoK and the Data Management Maturity (DMM) Model. However, they should be managed in an integrated manner, as they are in large part interdependent.[2] For example, without defined business terms, the starting point for business metadata, the metadata repository will lack meaningful organization. The same with quality rules for shared data, which need to be applied wherever the data may reside.
Note that all organizations have always been performing various data management activities, regardless of where they are sponsored, funded and performed (in IT, in the business lines, and within specific projects). However, many simply had not considered the EDM function as a unifying paradigm for those activities, meaning that they have been under-valuing their data assets, not giving them the attention or funding that Finance and Human Resources require, and leaving a cornucopia of benefits untouched. When you can engage executives in this discussion, there is near-universal agreement that data is vital to the organization’s success and that it should receive the requisite attention.[3]
The next term that needs some clarification is Enterprise Data Management Program. This is often a go-to term in our industry, mostly intended as a contrast with project-based data management. Here’s my working definition for this term:
- The infrastructure, programs, projects and staff resources that an organization commits to for the purpose of establishing and following sound data management practices for its prioritized data assets.[4] An EDM Program is advised to include four primary components:
- The Data Management Organization (DMO), a permanent centralized organizational unit, focused on ‘enterprise data’
- Data Governance, structured collaborative decision-making with representation across the organization, pertaining to ‘enterprise data’
- Initiatives /Projects – data management activities and resulting products, with a beginning, middle, and end, designated actors, and corresponding funding
- Sustaining Activities and Decisions – ongoing engagement in data management process and product updates and approvals
To summarize, EDM refers to the permanent organizational function of managing data, and the EDM Program refers to what the organization commits to, mandates, promotes and funds to implement and sustain a successful EDM function. The simple diagram below illustrates these concepts.
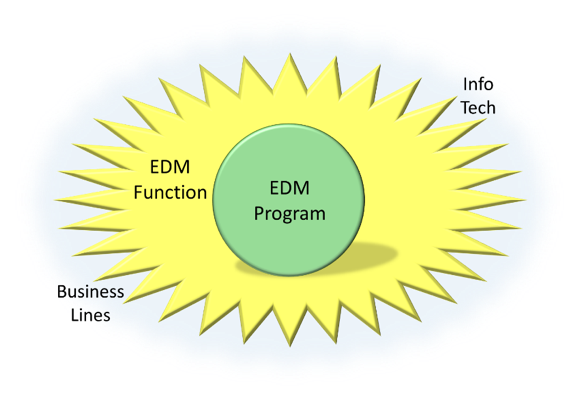
As shown, data management activities comprising the EDM function (the sunburst) are always being performed, both by the business lines and by IT. When an organization commits to structuring, organizing, and funding EDM capability building – organizational structures, policies, processes, standards and executes projects to implement them, it authorizes, funds and plans an EDM Program. A core chunk of funding should be non-discretionary and budgeted annually (e.g., think Human Resources) for the DMO and governance participants (i.e., charge codes), and discretionary funding should be allocated annually for capability-enablement business cases (e.g., creating the Data Management Strategy, developing a standard documented process for Metadata Management, etc.).
In an EDM Program, the Data Management Organization and Data Governance are key components, and projects are the activity mechanisms through which they work together to build data management capabilities. Sustaining activities pertain to the permanent EDM function, for example, modifications to the Data Management Strategy, modifications to metadata, etc.; these are executed collaboratively between the DMO and Data Governance.
Starting with the DMO – although data management activities are continually taking place, for example, data requirements are being developed, data sets are being cleansed, etc., to build out the EDM function, the EDM Program requires a permanent organization dedicated to:
- Data management capability development
- Creation and management of persistent products, such as policies, processes, standards, data definition repositories (e.g., the metadata repository, the business glossary, the enterprise data model, etc.) and the data management process library
- Promoting adoption
- Managing compliance
Quoting Ringo Starr, “Got to pay your dues if you want to sing the blues, and you know it don’t come easy.” The DMO is the backbone of EDM progress. It serves as the knowledge-based advocate of sound practices, and the accountable owner of the persistent products developed for the EDM Program. The DMO should be staffed with full time individuals who are knowledgeable about the organization’s business and skilled in data management disciplines[5].
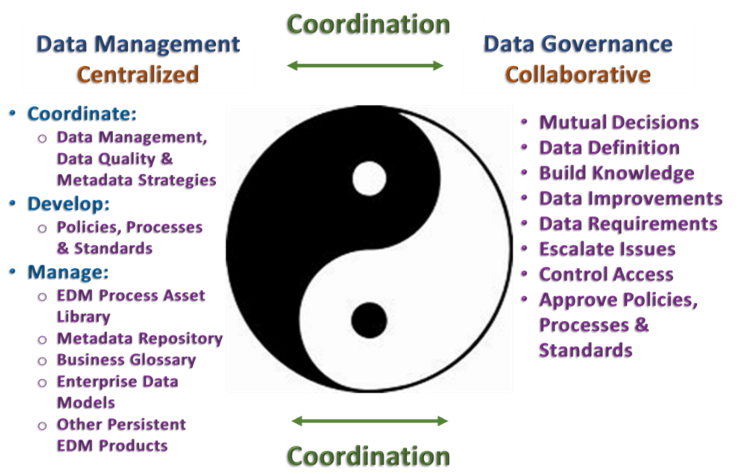
In the illustration, the DMO is shown on the left side as a Centralized function / organization. One of its key responsibilities is to coordinate creation of EDM foundational products, such as three important enterprise strategies: First, the EDM Strategy, which establishes the EDM Program.[6] Next, the Data Quality Strategy, which describes what data the organization will focus on, what disciplines it will standardize (e.g., Data Profiling, Data Quality Assessment, Data Cleansing), what technologies it will employ, and a high-level sequence plan the data scope to which the disciplines will be applied.[7] Finally, the Metadata Strategy, which describes the knowledge that the organization has determined to develop about its data assets (properties), how it will be captured and stored, how it will be maintained, and how it will be accessed.
The second key responsibility is to develop consistent policies, processes, and standards, persistent products intended to be applied across the scope of enterprise data. Organizations have implemented similar suites of policies, processes and standards for Finance and Human Resources, and these are usually mandated and widely communicated. As an organization establishes and strengthens its EDM function, the DMO takes a lead role in drafting, coordinating, and gaining approval and mandates for formalization of data management disciplines. Once these are established and rolled out, the DMO should develop a compliance assurance process (aka, Process Quality Assurance in the DMM).
The third key responsibility is to manage EDM products that will be maintained and enhanced over time. This is the control aspect of the EDM function, which should be centralized in the DMO. Some examples are:
- The EDM process asset library – where policies, processes, standards, templates, guidelines, and corresponding educational assets are stored, maintained, and made accessible
- The Business Glossary – as the approved terms represent agreement on the meaning of shared business concepts, this must be maintained following an established process
- The Metadata Repository – whether implemented in one platform or multiple platforms, knowledge about enterprise data assets is critical, and must be enhanced according to an established process
- If the organization has an enterprise data model, or domain data models, these need to be highly controlled, as new systems and repositories add to the scope of shared data
- Data management technologies implemented to support governance, data quality, metadata, etc., should be managed by the DMO (in contrast to ETL, data lakes, data warehouses, data marts, data provisioning services, etc., managed by IT)
It’s a significant bundle of responsibilities, isn’t it? And it requires corresponding staff resources to carry out. Here’s where I get on my soapbox and preach to the organizations who are reluctant to fund and champion a DMO – “This is the fire through which you must walk to reach the promised land.”
Moving to Data Governance – a DMO cannot accomplish these responsibilities alone. The EDM function is Collaborative. It depends on an actively engaged constituency, made up of executives, expert representatives from the business lines and IT. In a nutshell, governance is mutual decision-making about shared data.
Shared data refers to data that is: (a) within the scope of enterprise data defined by the EDM Program; (b) produced by one or more business lines; and (c) consumed by more than one business line. Reference data and master data – AKA, ‘highly shared data’ – are important subsets, as those data sets need to be timely, accurate, and highly available to multiple applications and user groups. Key responsibilities of governance groups include:
- Data definition – within the defined scope of shared data, definitions of key concepts (business terms) is the foremost responsibility of governance groups and representatives. The more important a shared concept is to the organization, the more carefully each relevant point of view should be heard and considered. For example, creating a shared definition for Product in a manufacturing organization.
- Knowledge description – governance needs to consider and agree on the scope of metadata important to the organization and determine what properties will be captured and stored.
- Data improvement – governance engagement in data quality is vital; governance business representatives are needed to determine what level of quality is desired (targets), what level of quality is acceptable (thresholds) and what quality rules should be applied. When there are multiple suppliers or consumers of data, these decisions are even more important, as rules ideally should be applied to shared data wherever, and whenever, it is captured and stored.
- Data requirements – business representatives have always been involved in determining data requirements for specific applications; for the EDM Program, this function is applied to requirements for shared data – repositories, reference data, master data, etc. – necessitating collaborative consideration and agreement. If enterprise data models or domain models are developed, governance representatives need to review and provide input to ensure that shared data is represented correctly for all relevant stakeholders.
- Issues escalation – when different business lines cannot agree on a decision due to conflicting business requirements, governance groups need to determine when issues should be are escalated for resolution. As an example, LOB 1 wants the values for Customer Status in the Customer Master Data Hub to be Active, Inactive, Returning, and Pending, according to the way it uses and refers to the data; LOB 2 wants the values for Customer Status to be Active, Inactive, and Unknown, and their definition of ‘Inactive’ differs from LOB 2. If this issue cannot be resolved through decomposition and parsing (e.g. applying logical modelling techniques), it should be sent to a more senior body to determine which LOB should change its terminology.
- Access control – the parties who control a data source (‘data owners’) have a responsibility to determine how access will be granted and to whom; the parties who maintain a data source (‘data custodians’ or ‘technical data stewards’) need to determine how access will be executed.
- Approvals – of strategies, policies, processes, standards, and templates developed by the DMO for the EDM program. Governance needs to review, evaluate and provide input on these products to the DMO, ideally in several review cycles, from the document outline to the final version. This is a critical function, as the EDM Program aims to increase organization-wide understanding of shared data assets and build sound and consistent management practices. Adoption will not occur without governance concurrence.
To establish, implement and evolve a successful EDM Program, it is recommended that organizations adopt the paradigm depicted in the diagram below – to act on the importance of, and need for, both a centralized Data Management Organization and an active, structured Data Governance function. This approach will assure strategic success in ‘realizing the organization’s data dreams’ through building and sustaining progress for the long term.
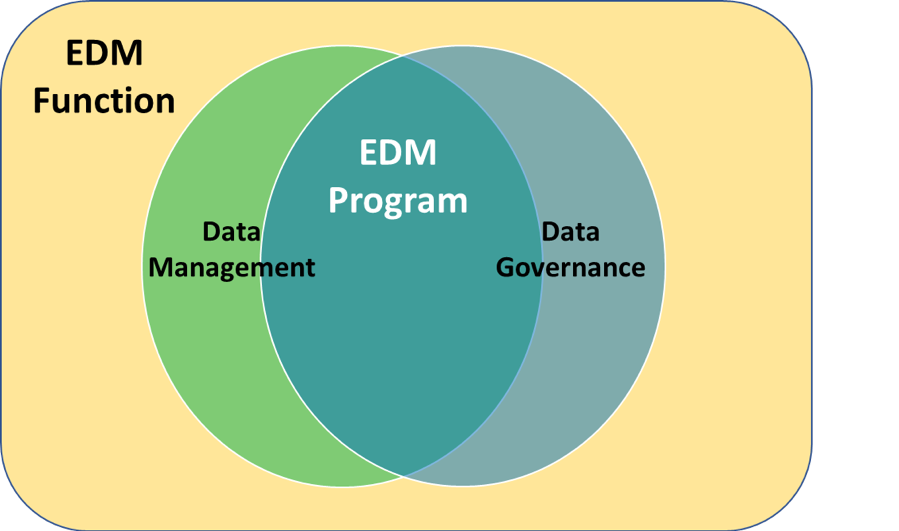
[1] While we’re here, let me put in a plug for developing a future enterprise data architecture. If your organization doesn’t have one, the path forward is obscured by fog. Eventually, the expense, inefficiency and complexity is likely to become a crisis.
[2] I often state that if all the dependencies and inter-relationships among data management disciplines were made explicit in a diagram, it would be a 5-foot-tall plotter diagram stretching around the walls of a 12’ x 12’ room.
[3] To hold their attention, you have to point out both why it is worthwhile, and why they aren’t realizing these benefits now; hence the value of evaluating current EDM practices and demonstrating how they link to business problems and aspirations.
[4] It is vital to define the scope of the EDM Program, i.e., what ‘enterprise data’ is for your organization – see my previous TDAN column ‘Why Your Organization Can’t Create an EDM Strategy’ for a more detailed discussion.
[5] See my column ‘Coming in from the Cold: The Enterprise Data Management Organization’ for a detailed rationale for instituting a DMO, and a suggested starter DMO organizational structure.
[6] See my column ‘Failing to Plan is Planning to Fail’ for descriptions and topic outlines for a Data Management Strategy, Data Quality Strategy, and Metadata Strategy.
[7] See my column ‘Head
in the Clouds, Feet on the Ground – How to Tackle a Data Quality Strategy.’
[i] Since this question is so frequently encountered, I encourage you to let me know if this analysis has been helpful to you and provide any comments you may have. Please feel free to contact me at melanie@datawise-inc.com
This column was originally published on TDAN.com in January 2020.