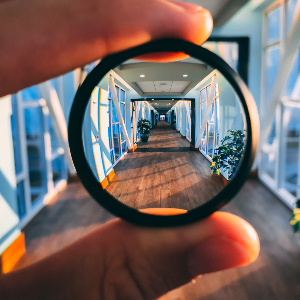
Am I right that you can’t open LinkedIn, read a tech or business blog, or attend a conference for more than 30 seconds without encountering the generative AI hype? It’s been this way ever since last fall when OpenAI unleashed ChatGPT upon a (mostly) unsuspecting world.
Depending on who you read or listen to, generative AI will save or destroy humanity; eliminate, create, or have a modest impact on jobs; be a useful, if limited, tool; or be just one more means for Big Tech to maximize profits. I am not going to venture into this debate, at least in this article, but Paris Marx, Emily Bender, and Timnit Gebru, among many others, provide illuminating commentary.[1]
Many are thoughtfully writing or presenting about the importance of data and data governance to generative AI. Our own founding publisher, Bob Seiner, has written on this subject, and one of his main points is that managing and governing data for generative AI is not too different from managing or governing any data. As Bob writes in his new book, “Non-Invasive Data Governance Strikes Again: Gaining Experience and Perspective,” “the data challenges presented by LLMs are consistent across all approaches to data governance.”[2] These challenges are familiar to any data governance practitioner and include data stewardship, data risk, privacy and security, data quality, and, extremely relevant to this scenario, data documentation.[3]
There is one aspect of data documentation that I have heard paired more prominently with AI than in other settings. That’s data provenance. What is data provenance? Like so many terms in the data governance vocabulary, there are umpteen different definitions. My favorite, and one which is relevant to the theme of this article, is from the Australian Research Data Commons:
“Data provenance is the documentation of where a piece of data comes from and the processes and methodology by which it was produced. Put simply, provenance answers the questions of why and how the data was produced, as well as where, when and by whom. Data provenance is metadata that confirms the authenticity of that data and enables it to be reused.”[4]
Licens.IO’s blog post How Data Provenance Drives Machine Learning Risk + Value, by Jillian Bommarito, gives an excellent overview of the applicability of data governance to the data at the heart of generative AI, the data used to train the large language models. Bommarito also supplies a healthy dose of word-nerdery that I found irresistible:
“Provenance is just knowing where something came from. You might even recognize the veni in its Latin form, provenire, as the ‘veni’ in Julius Caesar’s famous ‘veni, vidi, vici.’ So, while provenance is the technical term, you can substitute ‘origin’ or ‘lineage’ in most conversations.”
My authoritative source for a word’s etymology is the Oxford English Dictionary, and the OED traces provenance back to the Old French word (old as in back to the year 1294!) meaning “origin” or “cause.”[5]
This entire concept of tracing the definition of a word back to its origin is what provenance is all about, and Bommarito explains why this is important to data:
“Data lineage or data provenance has become increasingly important, as technology relies more extensively on previously collected or generated data, which is itself becoming more voluminous. Without information about where data originated, how it was obtained, and what has been done to it, users of said data expose themselves and their organizations to the risk of negative financial, legal, and reputational outcomes.”
The question of the origin of data used to train LLMs, and the rights of the LLM trainers to use that data without compensation creators, is not theoretical. Artists, studios, and programmers are suing open AI and other generative AI leaders. Sheera Frenkel and Stuart Thompson summarize this in a recent New York Times article:
“At least 10 lawsuits have been filed this year against A.I. companies, accusing them of training their systems on artists’ creative work without consent. This past week, Ms. Silverman and the authors Christopher Golden and Richard Kadrey sued OpenAI, the maker of ChatGPT, and others over A.I.’s use of their work.”
With regulators slow to step into the fray, how these lawsuits play out is anyone’s guess. The tech companies will try to claim protection under the Fair Use doctrine, but this is uncharted territory for that space.
But if you are a business keen to use generative AI to create (or co-create, with finishing touches added by human workers) sellable reports, illustrations, and other products, don’t think you can’t be subject to copyright suits. This is the case even if you have no idea what has gone into the training data for these algorithms. Dr. Lance B. Elliot explains this in his marvelous Forbes article, AI Makers Guaranteeing That Your Generative AI Output Is Safe From Copyright Exposures Might Be A Lot Less Filling Than You Think, Says AI Ethics And AI Law. (That’s the title, really.) I think his exhaustive analysis of the applicability of copyright law to user-generated content is a must-read for anyone considering using the technology, but Dr. Elliott sums up the core of his argument in one of his opening paragraphs:
“Yes, just to be clear, you the user of the generative AI can be and most likely are the one on the hook for copyright infringement based on generating and then using outputs that violate someone else’s Intellectual Property (IP) rights.”
If your business can be on the hook for copyright infringement, this takes us back to the concept of data provenance. Bommarito’s article draws a parallel to art provenance: “Historically, provenance has been most famously applied in the context of art. In the world of art, honest mistakes and malicious forgeries have resulted in many famous stories of long-lost masterworks or fortunes ill-gotten. And after World War II, the colossal scale of appropriation on the European continent still echoes in auction houses and private sales.”[6]
Bommarito’s article is excellent, as are any number of articles on this topic. So, what do I have to uniquely contribute to this discussion? When it comes to relating art provenance to data provenance, and considerations users must keep in mind regarding the origin of the data fueling the generated content, I happen to have a brother who is an expert in the field of misappropriated artifacts that find their way to museums and art collections across the globe.
Bradley J. Gordon is an attorney with a law practice in Cambodia. For over a decade, he has worked to locate priceless artifacts stolen from the country leading up to, during, and after the Khmer Rouge rule of terror. Many of these went from looters to unscrupulous art dealers to museums that often conveniently failed to thoroughly inquire about the provenance of these objects. CNN, Bloomberg, the New York Times, and many periodicals have featured Brad and his team’s work. Discovery Channel’s Expedition Unknown and Australian Broadcasting Company’s Treasure Hunters featured Brad’s teams’ efforts in recent episodes. Of course, I think the coolest thing my brother has done is being a consultant for John Oliver’s Last Week Tonight episode on museums and provenance.[7]
I spoke to my brother not long ago and asked him what differentiates museums that do art provenance right vs. those that, well, don’t. In the former case, several museums that have cooperated with Brad and his team have an art provenance expert on staff. These museums had done their homework — one allowed the team to see correspondence back one hundred years documenting the origin of the artifacts. These museums were willing to show everything they had, even allowing 3D imaging to help the team match statues with their bases from which looters had ripped them out.
Most museums, however, were not ready for these inquiries and had no dedicated art provenance specialists, and some notable institutions even responded by stonewalling. The British Museum and the Metropolitan Museum now face withering scrutiny and reputational risk. As Graham Bowley wrote in the New York Times, “Today, many U.S. museums are facing a reckoning for their aggressive tactics of the past. Attitudes have shifted, the Indiana Jones era is over, and there is tremendous pressure on museums to return any looted works acquired during the days when collecting could be careless and trophies at times trumped scruples.”
This pressure is coming from foreign countries like Cambodia, but also from U.S. authorities, which, “both local and federal, have made the return of looted cultural heritage more of a diplomatic and law enforcement priority. U.S. Homeland Security Investigations reports returning more than 20,000 items since 2007, largely seized from dealers and collectors, but also found in many of America’s most prestigious museums.”[8] Museums are subject to legal action over custom laws, foreign countries’ ownership and cultural heritage laws, and the 1970 UNESCO convention, where “nations pledged to cooperate and follow best practices to curb the import of stolen items.[9]” A number of museums in the U.S. are also facing seizures by federal authorities.
So, if you or your business are thinking of using generative AI to create, distribute, and sell content, you might want to hire yourself a data provenance expert (a new career path for data nerds!) And you should be certain that you really understand where the data used to train the generative AI models came from, and under what terms – or else you might find yourself featured on an episode of Last Week Tonight and have copyright lawyers knocking at your door.
Late breaking news! As I prepare this article for my editor, I read Joshua Hawkins’ article, OpenAI May Have to Wipe ChatGPT and Start Over. In it, Hawkins notes that “according to a new report from Ars Technica’s Ashley Belanger, the New York Times is currently discussing suing OpenAI after updating its terms of service to prohibit AI from scraping its articles and images to train language models.” We will see how this plays out, but it reinforces the importance of strong data provenance when working with the output of LLMs like ChatGPT.
[1] Paris Marx, techwontsave.us; Timnit Gebru, twitter.com/timnitGebru; Emily Bender, nymag.com/intelligencer/article/ai-artificial-intelligence-chatbots-emily-m-bender.html.
[2] Seiner, Robert . Non-Invasive Data Governance Strikes Again: Gaining Experience and Perspective (p. 337). Technics Publications. Kindle Edition.
[3] Ibid, pp 337-343.
[4] ardc.edu.au/resource/data-provenance/
[5] provenance, n. meanings, etymology and more | Oxford English Dictionary (oed.com)
[6] Bommarito, How Data Provenance Drives Machine Learning Risk + Value.
[7] youtube.com/watch?v=eJPLiT1kCSM
[8] NYT, Ibid For US Museums With Looted Art, the Indiana Jones Era Is Over – The New York Times (nytimes.com).
[9] NYT Ibid.