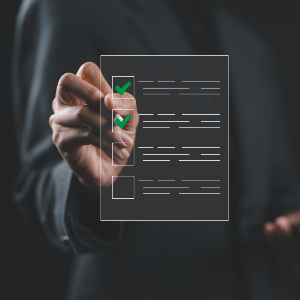
In 2024, our research at Dresner Advisory Services revealed that only 32% of organizations have a formal data governance organization in place. This statistic highlights a critical gap, especially as machine learning (ML) and artificial intelligence (AI) are increasingly integrated into operations, expanding business reliance on data and analytic content. Despite the growing importance of data products, few organizations approach governance with the deliberate, comprehensive strategies required to unlock their full potential.
Without question, the capability to maximize the value of organizational data, analytics, and ML/AI assets while mitigating associated risks depends upon robust governance practices. Moreover, the absence of a data governance framework has tangible consequences — 68% of organizations report challenges in finding reliable data and analytic content. The paradox is striking as businesses increasingly depend on data-driven decision-making; their success hinges on high-quality, trustworthy data and analytics. Without strong governance, this dependency risks becoming a significant liability.
To address these challenges and achieve the promise of becoming data-driven, organizations must establish holistic governance programs. These efforts really need to operate at an enterprise level, supported by technologies that govern data, analytics, and ML/AI assets comprehensively. Modern data architectures, such as data mesh and data fabric can help by logically embedded governance within an objective semantic layer, ensuring accessibility, security, and usability across the organization.
Jonathan Reichental, former CIO and author of “Data Governance for Dummies,” aptly captures the essence of governance in his book, emphasizing its role in managing data effectively, delivering value, and ensuring data remains secure and usable. When data governance succeeds, it doesn’t just safeguard resources, it transforms organizations by enabling a thriving data culture. In an era of rapid technological evolution, robust governance is not optional — it’s essential for sustainable success.
State of Data Governance
Governance for most organizations today is fragmented and siloed, often limited to specific enterprise applications or focus areas. While 31% of organizations report having a formal data governance program, the same percentage also find it easy to locate data and analytical content. This could suggest that in the absence of a formal program, governance practices are inconsistent, spotty, and suboptimal.
Industry trends show significant variation in governance adoption. Healthcare leads with 45% of organizations reporting formal governance programs, while education — ironically, the originator of metadata concepts through libraries — lags. Governance structures also differ: 53% of organizations centralize governance, 31% adopt a hybrid approach, and 16% operate with a distributed model. Interestingly, data governance is most frequently reported to a chief data officer (52%), yet fewer than half of organizations have a CDO, prompting questions about whether having a CDO correlates with governance success. Historically, CIOs in my discussions in the #CIOChat did not want to lead the function.
The primary scope of governance programs centers on data quality with 45% of respondents identifying it as critical and 35% considering it very important. Other key priorities include controlled access to data based on roles (37% critical, 39% very important), documentation and cataloging of data objects and related artifacts (33% critical, 35% very important), and metadata curation (29% critical, 29% very important). These priorities underscore the need for systematic approaches to manage data and analytics effectively.
Effective governance encompasses a broad range of requirements, including security, quality assurance for data and analytics, privacy management, lineage and impact analysis, and life cycle management of data, ML, and AI assets. Specific areas of focus include master data governance, metadata governance, analytical content governance, cataloging, cost monitoring, and optimization of technology-based governance. Advanced areas such as the governance of ML/AI training datasets, models, and algorithms are also emerging as critical priorities.
The state of these governance components varies significantly across organizations. Addressing them holistically requires not only a formal governance program but also an enterprise-wide commitment to ensuring quality, security, and proper stewardship of data and analytic assets. A deeper exploration of these challenges and opportunities is available in the full research report.
Projecting Forward
Our analysts anticipate the emergence of comprehensive enterprise governance platforms that will address the entire spectrum of governance capabilities. These platforms have the potential to transform how organizations manage and govern data and analytics. Vendors who succeed in this space will seamlessly integrate governance requirements within the semantic layer, enabling organizations to align governance with their strategic goals effectively. However, achieving this transformative capability requires more than technology, it necessitates the establishment of a robust, purpose-built governance program.
The cornerstone of a successful governance initiative is a dedicated program supported by resources that align with the required scope and complexity. This program must have strong executive sponsorship and a clear mandate, empowering the governance team to drive meaningful change. Without such support, governance efforts risk being reduced to mere compliance exercises rather than serving as strategic enablers.
To ensure long-term success, data and analytic governance efforts should start with a focused scope, targeting a minimal set of data and analytics content critical to core business or mission objectives. As the program matures, its scope can and should expand, but only in alignment with clearly defined goals, value-driven outcomes, and governance metrics. The Data Governance Institute underscores the importance of foundational elements such as data rules and definitions, decision rights, accountabilities, and tools — rooted in a strategy tied directly to mission-critical priorities.
Governance initiatives must demonstrate measurable value through key performance indicators (KPIs) or metrics. Organizations need to assess the scope of their efforts and show tangible business outcomes. Technology alone is insufficient for governance success; it must be combined with deliberate change management, clear metrics, and a focus on people and behaviors.
Critical to success is aligning the governance program with organizational goals and securing visible advocacy from leaders to champion the effort. Leaders should initiate the change management process early, providing opportunities for stakeholders to learn and adapt. Establishing meaningful metrics to measure progress is essential, as is emphasizing that governance is an ongoing program, not a finite project. By prioritizing the data and analytics that truly matter and fostering a culture of governance, organizations can unlock the full potential of their data assets and drive sustainable value.
Parting Words
The state of data and analytics governance reflects a pressing need for transformation. As reliance on data, analytics, and AI/ML grows, organizations must move beyond fragmented or ad-hoc approaches to establish comprehensive governance frameworks that deliver measurable value. Effective governance is not a one-time initiative but a continuous, enterprise-wide commitment that requires strong leadership, focused resources, and alignment with organizational goals. By embracing modern architecture, fostering a culture of accountability, and focusing on metrics tied to business impact, organizations can turn governance into a strategic advantage — one that ensures trust, unlocks insights, and drives sustainable growth in an increasingly algorithm-driven world.