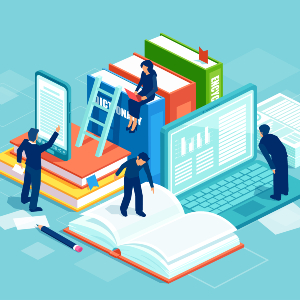
In the era where data powers digital transformation and informs the growing number of data products, it is critical that data practitioners have a common understanding of the things that make up a data governance program. I have found recently that even standard bodies lack a common lexicon for describing data governance artifacts.
The truth is we do not use terminology consistently between organizations. This makes it hard to judge the maturity of a data governance program and to develop continuous improvement plans. As well, it means that the tooling supporting data governance does not provide the functionality needed to improve data governance processes. In order to develop a consistent language between data governance practitioners, I will propose in this article definitions for data governance terms. This includes mission and vision, standards, business policies, data policies, data controls, and data control methods.
Mission and Vision
Let’s start at the top. According to the Data Governance Institute, the mission of every data governance program should be:
- Adding value to the organization’s products, services, processes, capabilities, and assets
- Reducing cost, complexity, and delays
- Reducing risk
According to Jonathan Reichental, author of “Data Governance for Dummies,” the missions for data governance programs should have “one of three flavors: protecting the business, running the business, and growing the business.” Each of these, of course, attaches itself to subgoals such as:
- Governance, compliance, and risk
- Improving the financial bottom line
- Improving business top-line growth
So, the mission and vision should be what the data governance program aims to achieve for the business and the “why” of the data governance program.
Standards
With the mission and vision defined, it is important to define the North Stars for the data governance program. This should include applicable standards, legal and regulatory mandates, and relevant industry best practices. Today, this needs to extend beyond the data itself. “Rewired” authors Eric Lamarre, Kate Smaje, and Rodney W. Zemmel suggest there should be “clear standards and thresholds for AI risk, including transparency and explainability, and bias and fairness for AI models.” Great standards and best practices to consider are “Privacy by Design,” FAIR, ISO 27001, and NIST 800-53.
Business Policies
So, what are business policies? The authors of “The Privacy Engineer’s Manifesto” say “a business policy is a high-level statement about information security and privacy. It lays out the key information and security directives for the organization.” Business policies should codify the mission and vision as well as reflect and comment upon the standards contained in standards.
Data Policies
Data policies should go to the next level of indenture. Data policies, however, should still be written in succinct, plain language. They should consider why data is collected and what purposes it is to be used for. They should also clearly define data retention and suggest what due diligence should be conducted with third-party personnel and/or vendors accessing data that contains sensitive or PII data elements.
Reichental suggests there are four types of policies: data access policies, data usage policies, data provenance policies, and data retention and archival policies. Digging in on the first two areas, the authors of “Rewired” suggest that “comprehensive digital trust policies address the use of data, analytics, and technology. These policies must be broader than traditional data privacy policies, and address topics such as use and handling of personal data, guardrails for use of technology, and fairness of code-based models.”
Data Controls
In contrast to data policies, which can be for a range of subjects including master data management, data quality, data providence, and the list goes on, data controls are where the action takes place in terms of protecting sensitive data or data that contains PII. This is where you control access to data.
This is accomplished traditionally in a database, but today it can increasingly occur in data security governance software. In these systems, controls can be implemented across data systems. Here, data can be administered locally or globally. However, the authors of “Rewired” suggest that we need to think more broadly about data controls. This means they need to do two more things: monitor and control AI models, including establishing bias and fairness checks for these models.
Controls Evaluation
Finally, controls need to operate in a continuous improvement and DataOps fashion. According to the authors of “Rewired,” this means “developing automated tests for security and compliance and refactoring applications to bring them into compliance.” Automating trust, they say, “is the process of turning trust policies into code such as compliance requirements. These automated risk controls are activated whenever anyone submits new code.” So, controls evaluation should determine if controls are working and increasingly doing this in an automated fashion can improve controls and make them work more effectively.
Parting Words
My goal for this piece was to get us all using the language of data governance consistently. It is a problem if one person’s control is really a business policy. Clearly, you are welcome to add your perspective and experience to this piece. We all need a consistent ontology for data governance and the artifacts that come with it.
Image used under license from Shutterstock