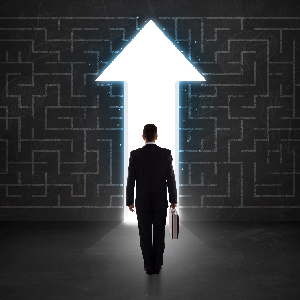
IoT (Internet of Things) incorporates many new and innovative technologies, such as sensors, smart devices, machine-to-machine communication, networking, advanced computing, and data analytics. One of the keys in the success of IoT is the data that flows underneath these technologies. Naturally, the IoT sensors and devices generate a huge amount of data automatically and continuously. What makes the IoT data “big data” is due to its value chain: More and more the IoT raw data is combined with data from other sources, such as personal and business data, to generate new information, especially business intelligence (BI).
The data from IoT can be ranged between low-level raw data and high-level generalized data. Different formats and sources of data introduce the data polymorphism and heterogeneity. The ambiguity of semantics, the error of measurement, and the dynamic change of data further lead to the data uncertainty.
Seven Vs to Describe IoT Data
When talking about the IoT data characteristics, the Seven Vs summarizes everything: They are Volume, Velocity, Variety, Veracity, Validity, Volatility, and Value.
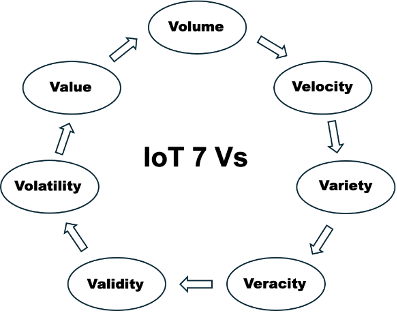
Details of Seven Vs
Volume
The IoT devices can produce real-time data in hundreds of not GB, but ZB per year. Such data needs tremendous storage and powerful system to process, e.g., data centers and the cloud. Imagine a scenario in a department store where millions of merchandises are available daily. If these objects need to be tracked per day, and each tracking generates 100 bytes of data, the total of continuously produced data may reach 100 GB per day and 36.5 TB per year to support object tracing and discovery.
Velocity
For many IoT applications, every millisecond counts. For example, a semi-autonomous vehicle operation requires near real-time data generation, collection, and distribution. A car’s acceleration can be detected from its speed changes. To capture these changes, the IoT data must be sent to the processing node swiftly and regularly.
Variety
The IoT data comes in structured, semi-structured, and unstructured formats. For example, the Komatsu Mining Corp’s JoySmart analytics platform ingests, stores, and processes a wide variety of data collected from mining equipment operating around the globe, often at very remote locations in harsh conditions. This data includes time-series data — machine pressures, temperatures, currents, voltages, and other sensor data — alarm and event data, and other data from third party systems. A single machine can have hundreds to multiple thousand data metrics and generate 30,000 to 50,000 unique time-stamped records in one minute.
Veracity
IoT devices generate data that can be inaccurate, inconsistent, incomplete, deceptive, and model-approximated, i.e., biased, noise, and abnormality. For example, the snow-covered road will make a car’s lane departure warning system less accurate or even cease to function; and if a rain drop covered a camera’s lens, it will produce vague pictures and videos.
Validity
The generated and received IoT data should be correct, accurate, and for intended use, which contrasts with the IoT data veracity.
Volatility
The IoT data tends to be time-bound and has a short time-to-live life span. For example, a proximity sensor detects and converts the presence or the properties of a nearby object into a signal. Then, the signal can be interpreted by a user or an electronic instrument that does not need to come into contact with said object. This IoT data is only valid when the sensor is close to a nearby object and can be erased when the sensor becomes too far apart from the original object.
Value
The abundant and rich IoT data may create tremendous value to businesses and organizations. While new sensor, mobile and wireless technologies are driving the evolution of IoT, the true business value of IoT lies in analytics rather than hardware novelties. The data generated from IoT devices is only valuable if it actually gets analyzed, which brings data analytics into the picture. Data analytics is defined as a process to examine big and small data sets with varying data properties to extract meaningful conclusions and actionable insights. These conclusions are usually in the form of trends, patterns, and statistics that aid business organizations in proactively engaging with data to implement effective decision-making processes.
The seven Vs help us understand what kind of data we are dealing with, so we are prepared when tackling the ever-increasing new IoT devices and applications. However, the data is only one piece of the pie in the entire IoT ecosystem that includes sensors, devices, applications, data, networks, storage, computing services, analytics, security, policies, and even regulation and legislation. Therefore, please stay tuned for the next article in this series about IoT.
About the Author
Dr. Qiang Lin is a Lead Systems Engineer at The MITRE Corporation and is a well-known expert on data architecture, data modeling, data engineering, and data governance; he has assisted DoD, U.S. Army, U.S. Air Force, DHS, VA, and IRS on many data management and data engineering projects for over 30 years. He has been an adjunct faculty at George Mason University since 2001. He is the co-author of the book “Internet of Things Ecosystem: 3rd Edition,” published in March 2022.
©2024 The MITRE Corporation. ALL RIGHTS RESERVED. Approved for Public Release; Distribution Unlimited. Public Release Case Number 24-0346.