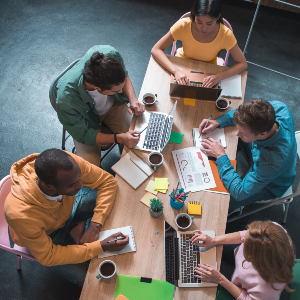
Well, of course, metadata is data. Our standard definition explicitly says that metadata is data describing other data. So why would I even ask this question in the article title? The reason I ask it is because we seem to think about and manage metadata as somehow different than “normal data” such as business operations data, master data, or reference data. Consequently, we often end up managing it differently.
For example, if I were to start talking to you about the principles of data quality management (DQM), would your mind immediately focus on the quality assessment of your business operations data, the data you use to build your data products? When we ask quality questions such as, “Which data elements are most critical?” or “What is the accuracy of the data, its completeness, and its timeliness?” Our tendency is to immediately frame these questions only in terms of business operations data. But if we believe that metadata is data, why don’t ask the same questions about our metadata? Why aren’t metadata assessments simply a part of regular data quality management assessments?
One positive aspect of this conversation is the rising adoption of data governance (DG). In some sense, DG is deeply concerned with metadata management. It asks the fundamental questions of: “What data do we have?” “What is in it?” “How it is being used?” and “Is it being used properly?” Usually, the first step in implementing a DG program is acquiring and populating a data catalog and business glossary. But this is only the beginning. We are quickly moving toward the fulfillment of a prediction I heard in a presentation many years ago. The speaker predicted that in the future, there will be thousands, if not millions, of bytes of metadata associated with each byte of business operations data! We may not be there yet, but I see us clearly moving in that direction as we try to automate data operations through robust metadata. Beyond just technical descriptions of format, structure, and content of a data item, the associated metadata can include data quality measurements, data provenance, data ownership, digital rights, and many other important characteristics.
In the case of DQM, while I agree that many DG metrics are like DQ metrics, such as data catalog completeness and structure, my experience has been that DG quality metrics for metadata are not nearly as comprehensive as those for business operations data under DQM standards. Why should DG metadata have a special set aside and not be incorporated into routine DQM assessment? While DG sets standards for DQM, DG data seems to be exempt from these standards. It begs the question, why do we need separate DQ assessments for business operations data and DG metadata?
Like so many aspects of data management, this dichotomy between business operations data and metadata is a legacy of storage management. In the days when storage was a scarce and expensive resource, operational data had priority over metadata. As a result, metadata was often not documented, and if it was, it was usually quite terse and off-line in paper documents. Metadata was rarely in digital format, much less formulated as intelligent metadata that could guide and automate data operations. Believe me, I clearly remember when the data center at the university where I was working made the agonizing decision to pay the additional lease fees to upgrade its IBM System/3 from 24K core memory to 32K core memory. By the way, we discovered that the 32K was already in the machine, the conversion was just to enable the additional 8K by adding a jumper wire!
While DG has clearly raised the visibility and importance of metadata, metadata has not yet achieved peer status with the business operations data that it describes. Most of the DG metadata standards I have had the opportunity to review mainly focus on the need to collect and store metadata, but lack specific requirements about its content, quality requirements and assessment, or its management. This contrasts with most DG data quality standards that go into great detail about mandating the development of data quality requirements framed in terms of specific data quality dimensions, defect reporting, collections, and remediation, data quality management processes, planning, documentation, training, continuous improvement, and other quality management practices.
Because of the legacy of prioritizing business operations data over metadata, I can see why DG programs see the need to call out metadata for special treatment. However, my hope is that eventually these will go away by simply increasing the scope of the data quality standard to specifically include metadata.
Building parallel standards for business operations data and metadata reminds me of my experience when I first started teaching at the university level years ago. In those days, most universities had a College of Education. If you wanted an education degree in a particular area, say mathematics, then you had to take the education version of each subject like algebra or calculus taught in the College of Education rather than the versions taught in the College of Science and Mathematics. The result was that the college of business grew into a campus empire as they added more and more of these parallel degree programs. Eventually realizing the inefficiency and unsustainability of this approach, most education programs now require students to first get a degree such as mathematics or biology from the Science College, then take some additional course on classroom and teaching methods. Perhaps we will see the relocation of metadata data quality requirements and management under the DQM standard soon as DG and DQM frameworks continue to evolve.