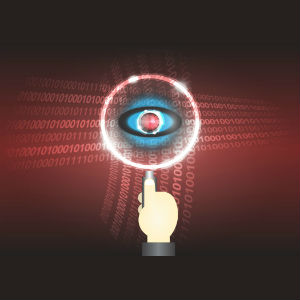
In the last few months, we have seen the wave of Artificial Intelligence break on the shores of wide-scale business adoption and mainstream media coverage of Large Language Models, most famously ChatGPT. While these technologies have immense potential to benefit organisations and society, they also bring with them an equal, if not greater, risk of harms.
There are many articles online discussing the potential future harms and benefits. Indeed, we are very rapidly moving through the stages of the Gartner Hype Cycle, but with added doomsaying on top of the usual over-inflation of actual capabilities. This article won’t add to that pile, but will raise some different questions for consideration from an ethics and governance perspective.
While the EU progresses its AI Regulation (“the AI Act”) in the face of extensive lobbying by ‘Big Tech’ against any form of regulatory controls over the emergent technologies, Sam Altman, the CEO of OpenAI, has recently testified to a Congressional Committee in the United States, advising US lawmakers that he felt regulation of AI was necessary. Geoffrey Hinton, the “godfather of AI”, followed in the footsteps of other ex-Googlers in going public with concerns about the potential risks of AI. In tandem with these developments, the last few months showed that Microsoft has followed in the footsteps of Google and Twitter in laying off their AI Ethics teams.
From an ethics and governance perspective the ‘tone from the top’ seems blaringly discordant. But if we look at these isolated information points through the lens of the E2IM framework that my co-author Katherine and I set out in Data Ethics (2nd edition), we can begin to identify some fundamental problems that we need to address quickly as a society and as an industry before we do the data equivalent of accidentally chopping society’s head off while combing its hair.
Our framework describes three ethical perspectives that we need to consider when thinking about the ethical use of data and data-related technologies such as Large Language Models. The Ethic of Society reflects the broadly agreed upon ethical norms and expectations of Society. These are often codified and expressed in things like Charters of Fundamental Rights or in legislation and other statutory Regulatory frameworks. The Ethic of the Organisation describes the core values of the organisation as reflected in the organisation culture, values, and internal governance structures of the organisation. The Ethic of the Individual relates to the personal ethical maturity and perspectives of the individual worker in the organisation. These personal values are often influenced by social factors in our personal background.
Organisations can influence the Ethic of Society through lobbying on legislation or other forms of regulation. The goal of this lobbying is to influence the regulatory landscape in a way that benefits the interests of organisations. Individuals can seek to influence the Ethic of the Organisation either through boycotting businesses whose values and ethical norms they disagree with or, in the case of people inside organisations, by formalising the support structures for individuals who are trying to influence the values of the organisation.
Altman’s testimony to the Congressional sub-committee in recent weeks is an example of an organisation seeking to influence the Ethic of Society. While it might look like a turkey voting in favour of Christmas, Altman’s call for regulation of AI in the US must be seen for what it is: a request for regulation that, while it may help mitigate future risks“will not impede OpenAI’s ambitions in the near term.” Altman’s call for regulation should be considered in the context of Eric Schmidt’s calls for the regulation of AI to be taken away from legislators and be placed in the hands of technologists.It should also be considered in the context of the extensive lobbying by technology companies against the EU’s forthcoming AI Regulation.
In this context, it’s worth noting that the current wave of AI risks and harms being discussed as requiring regulation are medium to long term harms which might arise in the future. There is limited discussion at the moment of the immediate and present harms and ethical problems AI tools, such as:
- discrimination in automated decision making due to data quality issues or bias in training data
- the processing of personal data in LLM training data
- the treatment and conditions of workers in developing countries who perform important tasks in the Human Reinforcement Learning process (needed to fine-tune the transformer processes that sit behind the generative AI tools that are now entering mainstream use).
These issues are significant challenges in the development and adoption of advanced data processing technologies such as AI. Ironically, addressing many of these issues that are challenges today might actually help mitigate some of the doomsday scenario risks that are the subject of the current frenzy of coverage.
The quest for business-friendly regulation or self-regulation, needs to be scrutinised further. However, in the context of how organisations are developing and nurturing the Ethic of the Organisation (their organisational culture and values) when it comes to the development of these technologies. Sadly, the news here is not good and there is a glaring disconnect between the public concern and the internal controls. A key influencer of ethical outcomes in data management is the nature of the situational moderators of ethical behaviour within the organisation.
As we discuss in the book, the internal data governance structures of the organisation, cascading principles to policies and processes, are important in ensuring that individuals take appropriate and ethical actions in respect of data. The termination of AI ethics oversight functions by Microsoft and others in recent months has resulted in the removal of key governance functions in terms of establishing and translating ethical principles into practice.
So, we find ourselves in a situation where the organisations promoting the development of AI tools are seeking to influence external regulation are, at the same time, are removing their internal regulation and safeguards.
Let’s make no mistake here: the tone at the top is telling everyone in these organisations that it is more important to implement the technologies than consider the legal, social, or ethical consequences. This is a pattern we have seen before in different industries, from Big Tobacco to Big Oil. It seems that Big Data might be heading the same way.
Of course, the way to stop a bad corporation with a gun is with a good person and a placard. Surely, the professionals working on implementing these kinds of systems will act in line with their individual ethical principles or the code of ethics of whatever professional organisations they belong to?
The ACM Code of Ethics sets out some wonderful principles that ACM members are supposedly committed to upholding. Principles like “Avoid Harm,” “Be Honest and Trustworthy,” “Be Fair and Take Action not to Discriminate,” and “Respect the work required to produce new ideas, inventions, creative works, and computing artifacts.” All principles which are fundamentally violated by the way in which much of the training data for LLMs has been obtained. OpenAI and other face lawsuits for infringement of IP, defamation, and more.
So why might people in organisations not follow their professional Code of Ethics in relation to the adoption and use of these powerful new technologies?
A key factor in the practice of ethics in organisations is the design of whether individuals in the organisation can raise concerns about the ethics of the organisation’s actions, such as the adoption and use of AI technologies. These structures can either be formally part of the organisation’s Governance or Data Governance structures or they can arise from peer support within the organisation from colleagues or managers (we call these ‘Individual Moderators of ethical behaviour’). Unfortunately there is a history of lone voices in these firms being dismissed or side-lined when they raised ethical concerns. And people in organisations will have seen in recent months the people whose official job was to raise concerns and provide guidance be downsized, so there is likely a strong disincentive to speak up.
Snitches get stitches after all.
In this context, the recent decision by Geoffrey Hinton to resign from Google so he was free to raise his concerns about the future risks of AI is both welcome and worth of criticism. His resignation highlights the situational moderators of ethical behaviour that exist in large organisations such as Google, which often limit the ability of staff to publicly express concerns about the ethics of organisational initiatives — he had to resign before he felt free to say anything bad.
But it also raises the question of the weakness of individual moderators of ethical behaviour such as peer support in organisation. After all, Geoffrey Hinton doesn’t seem to have spoken out when Tinnit Gebru and others were dismissed for raising concerns about Google’s AI technologies. The rise in unionisation of tech sector workers in the developed and developing world, albeit for different reasons, may yet prove to be an emergent driver of individual moderators of ethical behaviour in the technology sector.
Now is the moment when organisations need to shift from an aspirational stance on data ethics to one that actually starts getting real about the practical challenges of governing data in the emerging age of AI and LLMs. Important lessons need to be learned. The genie won’t be getting put back in the bottle.
- Regulation of AI will happen. The EU is already well advanced on the enacting their AI Regulation, other jurisdictions are examining legislation, and Altman’s call at the Congress will result in the US taking some steps in this direction.
- Regulation will take different forms in different jurisdictions, so organisations will need to focus on their internal data governance controls to translate generic ethical principles into tangible data management practices and to ensure that they can demonstrate compliance with regulatory requirements across different jurisdictions.
- These data management practices will need to include considering data quality issues in source data that is used to train internal LLM systems as well as the data protection and other issues that might arise from automated decision making based on machine learning models. The ethical questions of transparency of the algorithms and models developed will need to be addressed.
- Organisations will also need to address the challenges of data skill and understanding within their organisations to avoid the risk of ‘garbage in/garbage out’ in the development and implementation of AI processes. The ‘secret sauce’ of publicly available LLMs such as ChatGPT has been the Human Reinforced Learning that has fine-tuned the models currently available. Organisations will need to consider how this reinforcement learning will be carried out in their organisations, and by who. After all, one of the present ethical issues with AI is the use of staff in developing nations to carry out human work on AI solutions, a trend which has resulted in a move to unionisation of workers in Africa.
- Issues such as data protection, intellectual property rights, and commercial confidentiality will need to be considered as part of the development of the internal data governance frameworks for AI in your organisation.
- When considering the design of future work in our organisations, we will need to consider what we will want the future of work to be. While Sam Altman has suggested that AI will have an impact on jobs in the future, there will be an uplift in the quality of the jobs available. Altman has also suggested that future is not guaranteed and organisations embracing and implementing AI tools to support workers and accelerate productivity today need to consider what the workplace of the future will be. After all, we need to ensure that the society we deliver through AI is a society that meets the expectations of those living in it and is capable of supporting the dignity of all.
So, what’s a data geek gonna do? The AI hype cycle will continue. The eyes of the media and click-bait online commentary will continue to focus on the extreme ends of the bell curve, the promise of a technology panacea or the peril of a data-driven apocalypse. The reality of the future will, however, sit somewhere in the middle of that curve. The choices we make today as data professionals will affect the skew of that curve.
It is important to pay attention to the man behind the curtain in the debate on regulation. Altman and others seem to be pushing for a model of “regulation for thee, but not for me”, and the choices we make around how we regulate for the safe and ethical use of these technologies will shape the future of their adoption and their impact on society.
The focus on perilous doomsday scenarios that are being set out by the very people who are building the things that might give rise to the perilous doomsday scenarios need to be seen for what they are: a lobbying equivalent of shouting “Look over there! A squirrel” and pointing to the distant bushes to distract a sibling while you steal their picnic sandwiches. There are social and societal impacts of these technologies today. These impacts have been well documented and concerns consistently raised, and largely ignored, for many years. The baked in discriminatory biases of sexism, racism, or simple “you’re not a computer scientist” that have pervaded industrial development and academic research in this field have resulted in critical work by largely researchers who are female, or persons of colour, or from fields other than computer science being ignored, dismissed, or simply pushed aside.
To address the challenges of today, and to secure for everyone the opportunities of tomorrow, we must adopt a perspective on the ethics and governance of not just AI, but on the raw material inputs that feed the machine. For example, we need to recognise data quality as an ethical issue as it can lead to bias, discrimination, or simply crappy outcomes for people as we automate processes and place our trust in machines to ‘think’ for us.
The “tone from the top” needs to align with the recognition of AI’s potential risks and benefits, and it must ensure that critical voices are heard early and safeguards considered from day one. It also means that we need to ensure people are educated about how the technology works, its limitations, and the crucial role of data and data management in making AI magic actually happen. As professionals, we need to look to the ethics codes of organisations like the ACM and remember that we are called upon to implement data management tools and technologies responsibly. By doing so, we can navigate the complexities of AI and data ethics, ensuring that we progress responsibly and avoid unintended consequences that may harm society as a whole.