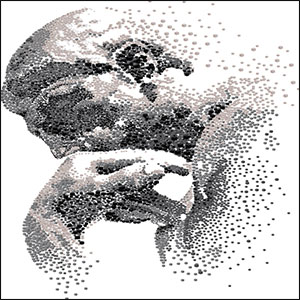
Why Your Organization Suffers
This series of columns addresses an essential function that many organizations neglect, an overlooked set of processes to manage the lifecycle of data acquisition and maintenance.
We’ll make the case for why this function is important, and we’ll define essential concepts and activities inherent in managing and optimizing acquired data– to meet business needs, progress towards a data-driven culture, improve efficiency, and avoid excessive costs.
In subsequent columns, we’ll define the data acquisition lifecycle, discuss what is required from governance, data owners, business sponsors, and procurement, and propose the approaches, policies, processes, and best practices that an organization should implement to obtain the right data for business purposes.
Along the way, we’ll address practical activities that you can perform to help your organization to:
- Define data requirements for data sources
- Select appropriate data sources and compare them
- Govern the data effectively
- Coordinate with procurement
- Develop effective contracts and service level agreements
- Collaborate with organizational partners
- Monitor and manage data providers.
Why You Don’t Know What You Have
Managing data for distribution and use starts with being informed about the company’s data. When starting a requirements effort for data acquisition, you may find that there is a lack of comprehensive knowledge of what data assets are under management. This is primarily due to the collective tendency, over many decades, to emphasize software features and new technologies without devoting commensurate attention to the data itself, without which business processes cannot operate. Nothing can happen without data.
There are many long-term factors that have contributed to this situation. A few of the most important include:
- A dearth of documentation for data stores, data in motion— interface— and data acquired from external sources, due to a lack of defined policies and processes
- A culture of delivery-first, which rewards specific implemented solutions and neglects instituting requirements to control data proliferation and redundancy
- A history of designing and building information systems to solve specific business problems, without appropriate attention to how shared data is managed across the organization
- A lack of effective communication and shared responsibility between information technology and the business lines, causing confusion about who ‘owns’ the data, and therefore, who needs to do something about it.
Therefore, most organizations find that their data is disorganized. For example, in a scenario where their financial solvency depended on compiling a complete list of data assets, they would be at severe risk of bankruptcy. The larger the organization, the longer it has been in business, and the more data assets it manages, the worse the problems are. The typical situation is:
- Data is spread across legacy applications and data stores— for example, five billing systems, with overlapping Client data sets.
- Data is incomplete, inaccurate, and inconsistent— for example, missing zip codes in addresses, values out of range, or conflicting country codes.
- Data is duplicated in multiple data stores— for example, a company acquired another company, and there are now two data warehouses with Product information, with different names, definitions, and codes.
- There is a lack of assigned accountability for data assets— for example, Who is the business owner? Who is responsible for obtaining the data? Who grants access permissions? Who can authorize the creation of new tables?
- The data lacks transparency for stakeholders and consumers— for instance, a consumer may ask: Where do I find Interest Rate data? Which source is more complete? How current is it? What’s the procedure for obtaining access?
This is assuredly a rather bleak picture. Organizations are overwhelmed with data, and unmanaged data assets constrain business performance.
Two important initiatives need to be accomplished to transform the disorganized and poorly managed data layer. Together, they enable delivery of clear and accurate information about the data assets, make them easy to find, establish clear responsibilities, and accelerate access, utilization, and value for consumers. The necessary achievements are:
- Creating a detailed map of the landscape, in effect, making sense out of chaos
- And, establishing sound processes and practices for selecting, managing, monitoring, and documenting data sources.
Managing Acquired Data – Benefits and Challenges
Your organization will realize a number of benefits from improving its policies, processes, and governance for acquiring data and managing data providers effectively and efficiently.
- Capturing knowledge about the data broadens and deepens stakeholder understanding about the data assets, which are often scattered across many projects and organizational units.
- When sufficient information is developed, it allows traceability from sources to destinations, increasing reporting accuracy, and enabling root cause analysis of defects.
- Discovery of redundant or overlapping data leads to better design decisions, and helps determine which are the best sources for the purpose.
- Determination of what organizations produce the data enables assignment of responsibility for data sources.
- Organizing and centralizing a catalog of data assets allows consumers to find the data and know who can authorize its use, increasing agility and effective collaboration.
- Standardizing baseline data acquisition processes for external data increases the accuracy of requirements, and helps ensure that the right data is provided to meet business needs.
- Standardizing the contract review process prevents performance risks and potential legal disputes.
- Standardizing baseline requirements for services provided for a data product increases the reliability of the data for consumers.
- Instituting a regular vendor communication process prevents possible issues.
- And overall, these benefits improve business performance, and reduce risk, re-work, and excessive costs.
Here are some terms we’ll use throughout the series:
- “Acquired Data” means data not currently providedby a destination system or repository, which needs to be obtained.
- “Data Provider” refers to an external or internal organization that produces, collects, or aggregates data and makes it available for distribution
- The provider is also known as the “Supplier.”
- The data provided can be referred to as a “Product,”
- And the receiver of the product can be referred to as the “Consumer.”
- The term “Service” associated with a Product refers to the actions that a Data Provider commits to performing with respect to a Product.
- The term “Procurement,” (aka, “Purchasing”) refers to the organizational unit that manages and executes the purchase of goods and services on behalf of your organization, helps the requestor to navigate policies and standards, and assists in legal review of contracts and service level agreements.
External Data Acquisition Challenges
Let’s explore common challenges to external data sourcing, including selection, comparison, and procurement, and for managing data providers, including contracts, service agreements, communications, and monitoring.
There are many potential issues that can occur when an organization acquires data from external sources. At the front end of the data acquisition life cycle, the need for external data is typically realized in the context of an implementation project such as a new or redesigned application, or when integrating external data with internal sources to create a repository or sandbox for reporting and modeling.
Often, external data requirements are not well-defined at project initiation; instead, they may come to light later in the project. Frequently, insufficient time and resources are allotted in the schedule to verify a precise scope. The business sponsor may express a high-level need, such as, “We need demographic data to integrate with regional product distribution and sales revenue.”
Since there are numerous data vendors offering products within the broad category of “demographic data,” this needs further analysis. For example, is the sponsor looking for statistics by Age and Gender? Geographic areas? Urban or rural? Specific cities? You can see that this situation risks delaying the project for remedial analysis. We’ll address this later in the Source Requirements Definition process.
Let’s say the requirements have been specified adequately, and the next task is to select the best data provider. Most organizations do not have a defined process for performing this efficiently; it tends to be ad hoc, project by project, with no defined plan and little guidance available. We’ll address this in the Source Selection Process.
In many cases, there are multiple data vendors who offer essentially the same product. Are there guidelines for determining value versus cost, comparing the original sources from which the product is constituted, and evaluating diverse contract and licensing terms? These considerations are addressed in Vendor Comparison.
Once the data source has been selected, obstacles may arise in the acquisition process. For instance, who has the authority to authorize the budget for the purchase— or, if a publicly available source has been chosen, such as government data— for the access agreement and ingestion efforts? Who is responsible for the initial data load, and for ensuring that the data continues to be available at the expected frequency? We’ll address this in Provider Management Governance.
If funding is secured, Procurement is involved. As with the selection process, many organizations don’t have a standard process for engaging with the procurement group. For instance, what is the lead time they require? Do they negotiate pricing for you? What are their policies? Is there a checklist for what the purchaser needs to provide to them, and when? We’ll address this in Coordinating with Procurement.
And then there is the matter of contracts, which may be complex. If the data is purchased, each data vendor will have its own standard contract, configured for a one-time purchase or recurring licensing. Contracts can contain surprises. For example, a financial organization purchased credit risk data and utilized it for many years, copying the data as needed for several data marts and the data warehouse. When they wanted to terminate the contract, the vendor informed them of a clause buried in the fine print, requiring them to delete all of the past data, wherever it was stored. This required design modifications and changes to interfaces, an expensive and time-consuming effort. We’ll address this in Developing Contract Terms.
In addition, organizations often haven’t developed a standard service level agreement template. This is important to codify what the data provider agrees to do in the contract— to provide the data at the agreed frequency, to ensure that the data is complete, to promptly resolve issues, to report changes, and so on. However, If the data is publicly available and not purchased, your organization may have minimal control over WHAT data is received, WHEN it is received, and its condition. We’ll address this in Developing Service Level Agreements.
External Governance and Cost Challenges
Most organizations have established formal data governance bodies with core responsibilities and authorities at several levels. Members of governance groups participate in mutual decision-making about shared data, in the areas of: data definition, determination of what information the organization needs to capture about its data assets, data requirements definition, and addressing data quality improvements.
This is a full plate of activities, especially in an organization with many business lines and data domains, so it’s not surprising that for the most part, there has not been a focused effort to define roles and responsibilities for acquired data— this tends to be ‘understood’ rather than explicit.
Since as we’ve stated, developing requirements for external data, and navigating its acquisition are the first steps in obtaining and utilizing external data, role descriptions for business data stewards and subject matter experts need to include process activity responsibilities. For example, responsibilities for these activities are often undefined:
- Who authorizes the requirements and selection process?
- Who leads a vendor comparison task, and who participates in it?
- Who explores the data elements included, the refresh frequency, the sources from which the vendor compiles the data, and so on?
- Who defines the quality characteristics that the data must meet?
- What is the governance role in working with procurement?
Most organizations find that they haven’t defined accountability for governance key roles in the management of data providers and acquired data assets, or assigned responsibilities in sufficient detail. Once we’re into the acquisition and provider management phase, the organization should designate:
- Who is responsible for determining if the data product already exists in the organization
- Who is responsible for authorizing data acquisition
- Who should review and approve service level agreements, licensing terms, and contract terms
- Who is accountable for assuring continued delivery of a data feed?
In general, there tends to be a lack of systematic monitoring for external data. The following questions illustrate this point:
- Does the organization capture delivery failures or information about data gaps?
- Who determines the severity of missing or incomplete data?
- Who performs periodic checks for quality characteristics?
- Who interacts with the vendors?
- Who escalates issues with the product?
- Who receives the notification for product changes?
- Who has the authority to exercise penalty clauses?
We’ll address these topics later in the Provider Management Governance section.
Organizations are advised to develop, over time, a comprehensive financial model of data management costs. This should include the cost of acquiring and maintaining external data products. Although this might seem obvious, it can be excluded, due to a project-centric or business line specific approach to acquiring external data. Cost factors that should be considered include:
- Redundant purchases that may occur in a large, complex organization, or when funding for external data products is controlled by individual business lines. For instance, a Federal agency, while engaged in populating a data asset catalog, found that an expensive data product had been licensed by three separate mission areas over several years. In this case, the data provider had not informed the agency of this fact, preventing it from consolidating costs.
- Organizations may fail to negotiate purchase prices or licensing fees, when multi-year purchases or multi-product discounts could result in product and servicing savings, thus ‘leaving money on the table.’
- Once a contract is finalized and data is received, the organization may keep paying for the product year after year, without determining if the data is still required, or even being used. A financial organization took the time to rationalize its externally purchased products, and discovered that many products were no longer being used by the original requesting business line. As a result, the organization discontinued these products and saved over two million dollars annually— a demonstrably worthwhile exercise!
Careful stewardship and monitoring of external data costs contributes to lower recurring spending, freeing funds for innovation. Executives tend to be enthusiastic about these efforts, because they have a positive impact on profits, and therefore improvement efforts should be greatly appreciated.
Internal Data – Disorganization and Complexity
Many of the same considerations that applied to externally acquired data also apply to data acquired internally. Although you might think that because the data resides within one organization, there would be fewer challenges, the other side of the coin is that acquiring internal data involves other organizational units and their associated management chains. You can hold a data vendor to a contract; it is more difficult to make demands on internal peer organizations.
In addition, internal data assets tend to increase in complexity over time. There are often multiple sources for the same data, and in an organization with a sizable application portfolio, the number of incoming and outgoing interfaces can number in the thousands.
When a project team needs to integrate data from internal sources, they often lack sufficient time to precisely identify what the source options are; the default is a tribal approach to acquiring data. For instance, “I know Mary Jones, the project manager for Data Store A, and I’ll call her to get access.” Data Store A may not be the best source to meet the business need, but it may be the most convenient location identified by this informal approach. It could lead to the project’s settling for data that is not as timely, or without expected quality controls, or simply unsatisfactory.
If data standards, and structured Application Programming Interfaces (or APIs) do not exist, or are not readily available, the project team may have to develop custom scripts to acquire the data. And, if the data is shared among many business lines, there is a significant risk of reinventing the wheel, incurring excessive effort and costs. Not to mention that divergent scripts are likely to have varying selection and quality rules, which could mean that the same data is inconsistent in multiple locations.
A lack of documentation presents a major obstacle in selecting the best source for the purpose. Let’s say Marketing wanted to define a Top Tier of client organizations, and they needed to discover which clients have spent the most to purchase their service offerings in the last calendar year.
In this derived-from-real-life scenario, there were several billing systems, each developed for a distinct service type, designed and implemented at different times, with overlapping Client data sets, varying structures, codes, dates, data elements, and different pricing algorithms. No business terms were defined, and only two of the systems had a documented data dictionary.
How would Marketing define, retrieve, and aggregate the information that meets its criteria for the Top Tier? (That’s a rhetorical question— they had to work with the maintenance project teams, profile the data, laboriously document the relevant data sets, identify discrepancies and duplicates, cleanse the data from each source, and design an integration data set— a Herculean effort.).
Another commonly encountered documentation deficit is a lack of mapping for business terms and attributes to a vendor solution. For instance, when a custom accounting system is converted to a standard commercial accounting system, it might be found that ‘Total Liabilities Amount’ in the original system is recorded in ‘Flex Field 17.’ If this is not defined and mapped, stakeholders may have difficulty finding the data, and reporting may be negatively affected.
As we’ve shown, its’s important to treat metadata— business, technical, and operational information about the data— as a critical asset. It is just as important as the data itself.
It’s impossible to fully automate your way to well-organized, consistently defined, searchable and accessible shared data. And, you can’t push all other business activities aside and enlist an army to document all the data you manage.
Organizations demanded a solution that balances factual reality— a disorganized data layer— with the core objectives of making the data understandable and accessible, thus accounting for complexity and reducing negative impacts in phases. Hence, the Data Asset Catalog. We’ll explore its basic features in more detail shortly. For now, let’s assume your organization has purchased a catalog product, or built a catalog view in an existing metadata repository. If the organization of the catalog has not been well-planned, problems may occur, such as:
- Acquired data sources are not aligned to data domains. If the organization has defined domains, it may have assigned production data stores to domains, but not captured the same categorization for data feeds and interfaces
- The organization hasn’t developed an intuitive taxonomy that enables flexible searches. Catalog users should be able to search by single or combined domains, by business terms, by attribute names, by originating source, by frequency, and other types of queries.
- The sequence of catalog population has not been prioritized by domain, causing some important shared data to be missing. This may cause stakeholders to become discouraged in their initial use, and lead to lack of adoption.
An organization is advised to aim for development and maintenance of metadata for all of its shared data assets, comprising a description of the existing (or As-Is) data architecture. This is definitely a long-term effort, and should coincide with major implementation or integration efforts over several years. There are many challenges with creating a reasonably complete description of the existing data layer.
- Often, interfaces lack descriptions and documentation. Typically, APIs are well documented, but most organizations also maintain hundreds or thousands of legacy point-to-point interfaces. For example, one organization’s large data mart had over 90 incoming interfaces and over 100 outgoing interfaces.
- When the organization set out to redesign the data mart into well-organized components, they found that many interfaces were undocumented. This meant that the business stakeholders had to guess whether the data was still needed, and if the original source was still the best. They also had to determine what information being consumed, and what information being supplied, would be replaced, or redirected to new locations. The transformation into well-designed, streamlined architectural components, not surprisingly, became a much bigger effort.
- There may be few designated ‘authoritative’ data sources. Some organizations have not fully determined the best sources for shared data— whether an internal data store, or an external data product. For example, if an organization needs reference data for client corporation acquisitions, mergers and spin-offs, an external data provider’s product may be the best, most complete source. If authoritative sources are not designated, it leads to increasing complexity and hinders consolidation efforts.
But Don’t Be Discouraged
Looks bleak, doesn’t it? Gets a bit worse before it gets better. The Grateful Dead sometimes used to stave off multiple encores by “ending with something dire.” That was usually ‘Black Peter,’ a derelict death song. In Part 2, we’ll start out with another set of challenges, persistent issues around data governance for acquired data. But then, we’ll transform into ‘Sunshine Daydream,’ as we turn our attention to the programmatic solution to these problems, re-architecting and enhancing the data acquisition lifecycle.