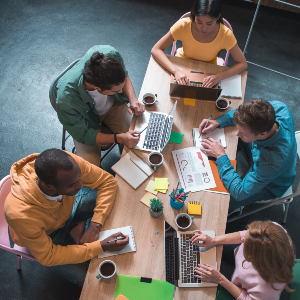
Now that “data” is finally having its day, data topics are blooming like jonquils in March. Data management, data governance, data literacy, data strategy, data analytics, data engineering, data mesh, data fabric, data literacy, and don’t forget data littering. In keeping with this theme, I’d like to propose a couple of new data topics not yet widely discussed: data love and data limerence.
If you are reading this article, then you are likely already experiencing data love. Call us data geeks or data freaks, but there’s a growing community of people who enjoy getting our hands dirty with data. We love the challenge of wrangling and wrestling data into useful information and extracting meaning and creating value out of piles of data. Our love of data is what makes us eager to get back to our computers every day with the hope of discovering something new.
For most people including myself, falling in love with data was experiential. I spent most of my early years studying mathematics, which eventually led me to a career in computer science. When I can find the time, I still enjoy programming. Building something and seeing it work is always fulfilling. But in those days, we just didn’t pay much attention to data. It was viewed as a commodity. Even for me at that time it was just a necessary evil to make my precious programs run.
My first real data job was leaving academia to work for a data brokerage company. Even though the data volumes then pale in comparison to today, at that time it seemed massive to me compared to my teaching exercises. I had never thought about processing millions, even billions, of records. I will never forget one day when I was working on a data quality problem with my boss. I was conjecturing about what I thought the problem might be, and he said to me, “John, always look at the data!”
Although this statement may seem obvious, over the years I have found it to be quite a profound insight. Following his advice has helped me navigate through many knotty data quality issues. Whether it is analyzing a data profile, querying a database for a specific value, or just randomly viewing and scrolling through lines and lines of data, understanding what values are really in your dataset is the foundation, the starting point, for any effective use of the data.
However, over the years, I have discovered that in contrast to data love, many people have a different experience that I like to call “data limerence.” Psychologist Dorothy Tennov coined the term “limerence” in the early ’70s to describe an intense infatuation with a romantic partner where the affection may or may not be reciprocated. At its root, the person experiencing limerence has an unrealistic view of the relationship. Unlike a love relationship where the partners see and accept each other as they really are, a person experiencing limerence often imagines the object of his or her affection as perfect in every way. They have an unrealistic view of the relationship, always imagining the other person as feeling the same way, ignoring any signals or warnings to the contrary.
Over the years, I have met many people who seem to suffer from data limerence. As with romantic limerence, people suffering from data limerence have a mental image of how the data is structured and stored that is not grounded in reality. And like romantic limerence, the data is often imagined to be in perfect conformance with some model or requirements. In many cases, even the model or requirements are imagined, not actually documented. But as we all know, if we take the time to actually look at data, the reality is usually quite different. We are all too familiar with the causes. Data is often not captured correctly, it may be the wrong data, important data is missing, values get misclassified, metadata is missing or incorrect, and even when correctly entered, it immediately begins to decay.
Two groups that seem to me to be more prone to data limerence are business managers and software developers. In the case of managers, this could be more understandable. Perhaps because they have never had the time or the opportunity to even take a quick peek at the data. But most probably it is because their view is that this is someone else’s job. On that note, you might be surprised to know that in my data story, the boss who encouraged me to look at the data was not only quite a data gazer himself, but he was also the CEO of the company!
As technical people, you wouldn’t expect software developers to ever have this condition. The problem is that most programming requirements only describe perfect data. There seems to be an assumption that when the program reads data, each input will satisfy all data requirements. Perhaps the developers assume this will always be done by some pre-processing step that someone else is responsible for. Software requirements rarely address how data that does not meet requirements should be detected and handled in a way that does interrupt or corrupt the entire process. Some of the most difficult system problems I have encountered in my career were the result of the unanticipated interactions between data and software.
In general, data limerence usually occurs when someone’s view of data relies on a secondary source such as a design document or simply what someone else told them. So, as data lovers, you must educate those suffering from data limerence and help them open their eyes to data reality. Nothing helps the students in our courses at the university more than giving them assignments requiring them to wrangle with large, dirty datasets. Companies could include similar exercises in their data literacy programs. Thomas C. Redman has developed a great example of this. He has a great exercise along these lines, called the Friday Afternoon Measurements (FAM), aimed at managers whose job depends on data. But no matter how you go about it, as my CEO once said, look at the data!