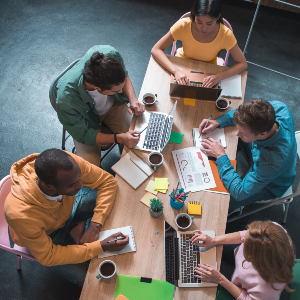
One of the greatest contributions to the understanding of data quality and data quality management happened in the 1980s when Stuart Madnick and Rich Wang at MIT adapted the concept of Total Quality Management (TQM) from manufacturing to Information Systems reframing it as Total Data Quality Management (TDQM). It was based on the principle that the purpose of information systems is to produce information products.
Instead of steel and aluminum, the raw materials for information products are data, instead of machinery, the processes are carried out by software, and instead of producing cars and toasters, you produce reports and data services. By applying this simple analogy, many the quality management practices from manufacturing like quality assurance, quality control, root cause analysis, product management, and product life cycle could be lifted from TQM and directly applied to information systems.
I have found that approaching the management of information as product can inform and improve frameworks for data management, data governance, data quality management, and data analytics. However, in my experience, I often find that organizations, even those trying to undergo digital transformation, are either unaware of this concept or choose to ignore it. They focus on managing their data sources, but don’t practice product management as if the products and service they are building are simply by-products of the process.
We all know how easy it is to confuse the means with the end, to get caught up in the technology and activities and lose sight of the end goal. But the reason we collect data and install software systems it to build information products and services that produce value for the organization and for the users of the products and services. Everyone is beginning to buy into the idea that data represent one of the most important assets of an organization. However, data resting in your database or data lake only has potential value. The value is only realized when it is transformed into an information product or service used to solve a real business problem.
Personally, focusing on information as product has informed my understanding and approach to data and information quality. In manufacturing, one of the most common quality assurance processes is to ensure that the dimensions of the parts are within certain tolerances before assembly. While everyone can agree upon the definitions of physical dimensions and characteristics such as length, diameter, and tensile strength, there is not the same consensus on the dimensions and characteristics of data. They are not as easily understood as physical dimensions.
To solve this problem, much of the early research in data quality centered around defining frameworks of data quality dimensions. The difficulty is that data quality dimensions tend to fall along a continuum from objective dimensions where there is general agreement such as accuracy and completeness to more subjective dimensions such as relevance, manipulability, and objectivity (free of bias) where there is much less agreement as to their exact meaning or even what the dimensions should be. Consequently, many practitioners and academic researchers have proposed different frameworks of data quality dimensions of varying size and shape.
Over time, consensus has evolved around the basic dimensions of data quality making it possible to define requirements for data tolerances. The International Organization for Standardization (ISO) has embodied the concepts of TQM in the ISO 9000 family of standards. The ISO 9000 standard (ISO 900:2005(E) 3.1.1) defines quality as the “degree to which a set of inherent characteristics fulfills requirements.” Therefore, the ISO definition of data quality is the degree to which data meets data requirements (stated in terms of the data quality dimensions). Data quality is about assuring that the components of an information product or service will fit together properly. Data quality is measuring and improving the condition of the data.
Many people like to use “fitness for use” as the definition of data quality, but in my opinion, this is a case where the TQM model has been somewhat misapplied to information systems. While this phrase comes directly from the mouth of Joseph Juran, one of the cherished godfathers of TQM, he was clearly speaking about the products, not the raw materials. While having good quality data going into a production process is necessary, it is not sufficient to ensure the final product meets the expectations of the user, or in Juran’s words, is fit for use.
This is reason why I like to make a distinction between the terms “information quality” and “data quality.” Whereas data quality is about assuring that the components of an information product conform to tolerances, information quality is about measuring and improving the quality of the information product or service. While many people like to use these terms interchangeably, I believe by separating them, it can help bring back lost focus on information product. Data quality for the data components and information quality for the information products.
It reminds me of the engineer’s dilemma, “Am I building the thing right, or am I building the right thing?” Of course, we want to do both, build things that people want (the right thing) and build them the right way (according to requirements). Data quality is about building information products and services right, i.e., according to data requirements, and information quality is about building the right information products and services that create value for both the producers and the users.
Now at this point, we could invoke the ISO definition again and ask whether an information product or service meets user requirements. But in my experience, users don’t always have well-defined requirements. Using this approach would also entail coming up with yet another dimensional framework to express information product and service features.
Instead, I think it is simpler and more in line with the current movement toward recognizing “data as an asset” to frame information quality in terms of value. For example, in our program at the University of Arkansas at Little Rock, we define information quality as “maximizing the value and minimizing the risk of an organizations information assets and assuring the information products produced by the organization create value for the customers who use them.”
This definition embodies both the inward facing view of data producing value for the organization, and the outward facing view of the information products producing value for the users of the products. This moves the units of measurements for information quality from the realm of percentage of conformance to a way of expressing value.
Broadly speaking, the value proposition for most organizations falls into one of three categories – monetary (for-profit), quality of life (medical, non-profit, NGOs), and mission accomplishment (government, military). As an example, take a monthly invoicing as an information product. It would be built from several data sources all of which could have many data quality requirements. For example, one source would likely be the customer master and it could have many data quality requirement related to the completeness, validity, and accuracy of the delivery address components. If the customer is the billing department, then their information quality measurement is how effective the invoices are in collecting the balances due. While we can identify many desired features of the product such as the deliverability of the address or the accuracy of the amount due, ultimately the quality of the product should be valued in terms of its business purpose, to collect dollars owed.
Similarly, the primary information product of a medical research project to study the effectiveness of a treatment intervention is typically a study report, and the report’s information quality should be the measure of its effectiveness in improving the lives of the study subjects. A campaign by an agency charged with reducing homelessness in the city evaluated by how many homeless people are placed in homes.
All too often, there is a disconnect between the producers and custodians of data and users of the information products and services they create. I even see this in organizations launching data analytics units where the data scientists are more focused solving technical problems than business problems. It seems to me that we can’t fully achieve data literacy in an organization until each employee understands how his or her work contributes to business value realized through the organization’s information products and services.
Starting with products can be a useful exercise not only in data quality and data analytics, but data governance as well. For example, starting by populating your data glossary with the items from your data products such as report and KPIs, then tracing back to understand which data sources should go into the data catalog. There are a number of great articles about techniques for creating IP (Information Product) Maps.
Data speaks to us through the information products and services we build. What are they telling you about data quality, data governance, and data analytics in your organization? We should be listening to them. As Covey would say, “Begin with the end in mind!”