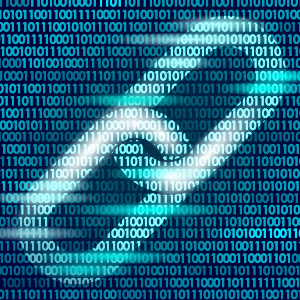
We have heard a lot in the news lately about cost cutting, operational reductions, and the dreaded news of layoffs. If companies hired more than what they anticipated for long term needs can we qualify that decision to be a data driven decision?
Companies that have enabled collaboration, transparency, and accountability by extensive use of data and insights in daily decisions at all levels have survived the need for recent layoffs.
In this article, we will continue with a deeper review of the proposed enterprise data management block framework that visualizes the main building blocks to be data driven.
In December of last year as part of this quarterly column we focused on Data Foundation – Level 3 and Data Enablement – Level 4 of the proposed framework for an organization.
In our ultimate and continuous goal for a data driven organization with Data as an Asset (DaaA) mindset, let’s review the key levels of Data Decisions – Level 5 and Data ROI – Level 6.
Data Program Management is a variant of standard IT, business, or product program management. Prioritization of initiatives, business case creation, SDLC, detailed planning and execution all still apply, but it is a very focused approach on data. Data project/program managers need to understand at high level data governance, data modeling, data infrastructure data privacy principals and deeper understanding of ED-DLC (Enterprise Data Delivery Life Cycle). ED-DLC at its core is a detailed work break down structure for all activities of data project approvals from detailed steps needed for governance, to building and testing of data tables, analytics, and usage of reports and data science in production. In the Data Decisions level of this framework, data program management plays the key role of which data initiatives get prioritized and how these enable the Data as an Asset mindset. This works by tracking the business value generated from implementation and usage.
Data Metrics enable key performance indicators that are part of all decision making at any organization. For company to be data driven, the concept of data metrics play a key role. Awareness, understanding, and enablement of data metrics like, “amount of data being stored in databases for each application,” “amount of data on cloud X vs Y,” “data transfer costs”, “data storage costs per application,” “data ingestion vs data transformation cost for each business process,” “number of reports by application, user, etc.” allow for operational excellence and ease of data return of investment calculations. Data metrics also encompass metrics on data quality, data usage and metrics on database tables, metadata, data protection cost, etc.
Insights Generation is the core analytics process of converting knowledge to actionable insights. Evolution from standard regulatory, compliance, and run the business reports to predictive and prescriptive analytics are all foundational to insights generation. Enablement of faster decisions based on the insights is key to enable data as a strategic advantage. Knowledge as gained from data metrics and data program management will allow accelerated decisions on value of internal data and mapping to potential data products for monetization.
Insights generation includes the management of decision framework which starts from key business questions and ends with key decision based on data enabled alternatives with projected profit/cost simulations as an example.
Data Science is one of the key enabler blocks in the Data Decisions level proposed framework.
This block includes data science talent, organization, frameworks, critical thinking, deep domain knowledge, statistics, and above all, integration of data science models with key operational systems. Selection of the right data science platform and enabling scalability, and stability is often the most critically limiting or accelerating factor towards a data driven organization. A best-in-class data science platform provides integration, ease user interface, feature engineering scalability, DevOps, automation on testing, data, and model drift reports as some key features.
Reporting Technology is the infrastructure that brings the semantic and visualization layers together for data consumers. Selection of the right reporting technology and enabling scalability and stability is often the most critically limiting or accelerating factor towards a data driven organization. A best-in-class analytics platform has the right operational model that balances the speed of development by power users to enterprise and line of business security policies.
Data Innovation, Data Products & Services,in the context of enterprise data management, is the top-level objective of Data Return on Investment Level of the proposed framework. Innovation that can directly be attributed to any of the foundation enterprise data blocks, as discussed in the framework, can be classified as “Data Innovation”. Most of the time this kind of innovation is visible as a monetized internal or external data product of service.
Most of the business value is gained due to a new or non-traditional way of enabling the collection, processing, or usage of data, data technology, or new data sources. In many cases, these data innovations are either envisioned as a product or tend to be usage of data for customer requirements that may never have been defined or analyzed for a need.
Regulatory & Compliance is a non-negotiable block as defined in the proposed data enablement level of the above framework. Business domain defines the local, regional, or geographical level requirements for compliance. These ever-changing requirements in many of the enterprise data management initiatives often drive innovation, collaboration, and accelerated enablement on new policies, processes, and in many cases, new technology.
This proposed block as part of the framework is a domain that connects decision makers at all levels to users that may not even be using the data and are mostly data providers. In many cases, this domain can be seen as an end result or a non- negotiable data product / service that oftentimes required technical implementation at the data field, attributes levels.
Summary
There are 26 proposed Blocks as defined in the framework with 7 levels: Organization Mission, Data Mission, Data Foundation, Data Enablement, Data Decisions, and Data Return on Investment.
The goal for these defined levels is to help set any organization to be Data Driven for Competitive Advantage by enabling growth, productivity, profitability, differentiation in products, services, customer experience or being compliance savvy. We have covered each of these 26 blocks in details as part of this quarterly column. Thanks for reading.