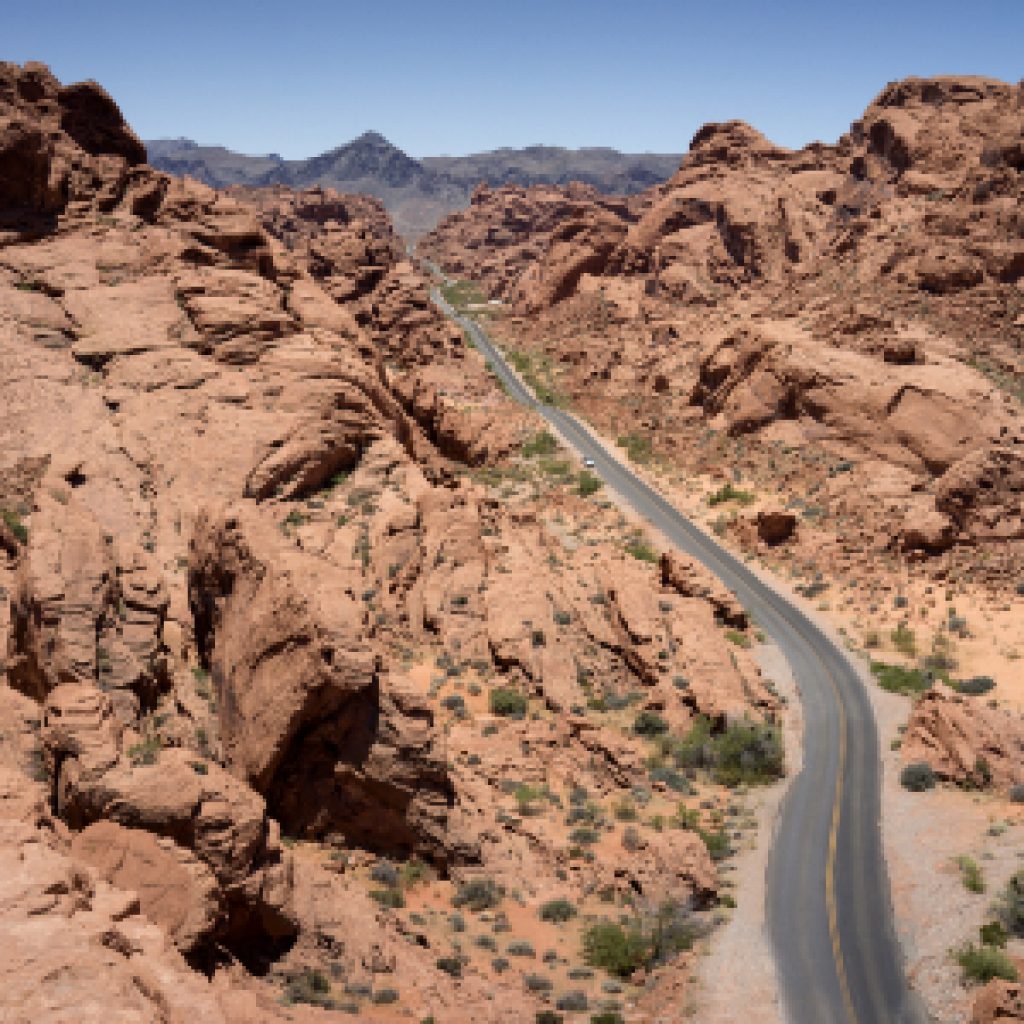
A few months back, our company enjoyed a retreat a few hours north of the city. We bonded, brainstormed, and set visions and goals for the development of our company and our products. We also had bonfires with s’mores, and that’s more relevant than you might think.
We ended up with a surplus of marshmallows – about 4 extra bags. They went untouched for a full week, sitting in a drawer beside the coffee, meaning they were definitely known about, since the coffee can only be described as ‘untouched’ for a maximum of 30 minutes at a time in our office.
But then, one of our staff emptied a bag the marshmallows into a clear container, similar to a few other snacks we’ve got around here.
By the end of the day, there were four left.
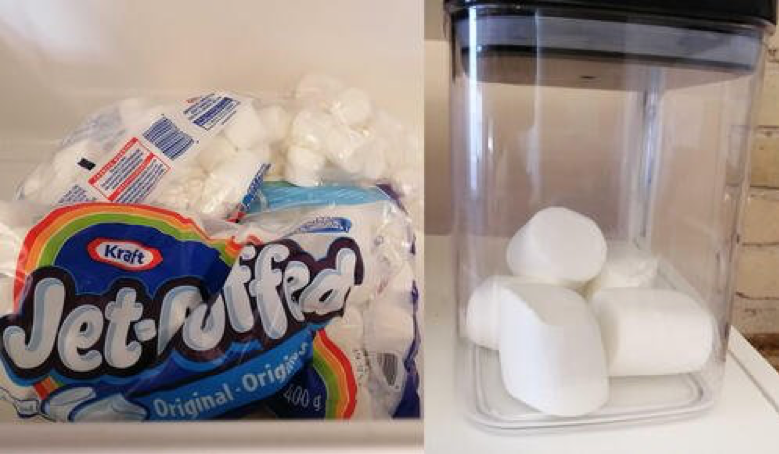
A before and after photo. The container on the right started that day full to the brim.
Why Are You Telling Me This?
Don’t get me wrong, I know this sounds like bad, typo-ridden clickbait up to now:
We moved some marshmallows into a clear container and YOU WON’T BELIEVE what happens next!
But they’ve been going down steadily. And if you didn’t think this was going anywhere worth your time, buckle in, because I am absolutely about to relate this to data.
By moving them, presenting them, and solving a few logistical problems, the marshmallows went from available to accessible, and there’s a world of difference between the two.
Okay, I’m Starting To Get It
There are quantities of data available that are greater than any human can perceive. It’s being generated through and by innumerable smartphones, computers, sensors, IoT devices, and basically anything that plugs in. All that on top of surveys, censuses, natural events, market events, etc. In fact, I think it’s time to update Newton’s third law:
For every action, there is an equal and opposite reaction, and data collected on both.
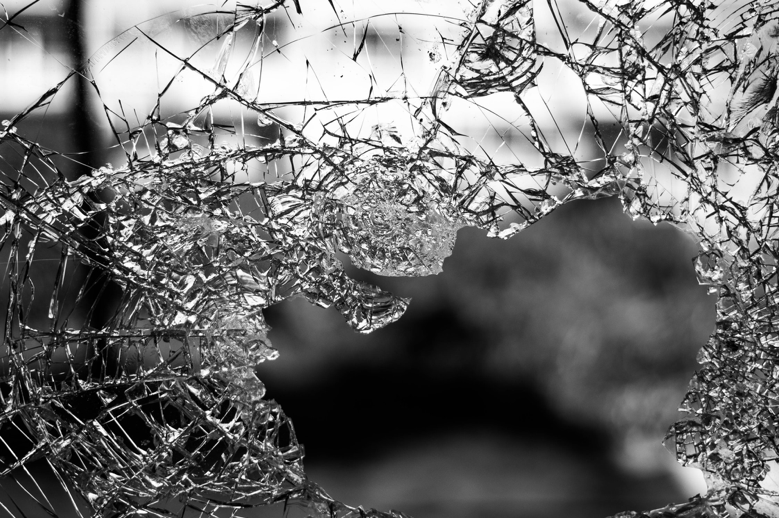
For example, if you defenestrate your computer in frustration,
the window will be replaced with a similar, unbroken window, probably at your expense.
But this data is seldom accessible. It’s hidden away in portals, it comes in obscure formats, the headers are wrong, column types are wrong, the character encoding isn’t what you’re used to – there are all kinds of barriers.
Taking advantage of alternative data means transforming its availability into accessibility. Useful data isn’t easy to find through search engines. Pulling data down once from a clunky web portal isn’t the same as managing scripts to perform scheduled gathers at regular intervals. And once you’ve sorted all that out, you don’t just magically have the data you want, it needs to be reformatted, cleansed, and standardized to integrate with the existing data and infrastructure in place within your organization.
What Can Be Done?
Luckily, data is mutable. There are processes for transforming it, normalizing it, and packaging it into an optimized schema that would make the data easier to access and therefore more valuable.
However, that doesn’t scale when it comes to solving with people power. No matter the size of your organization, there will come a time where the amount of useful-but-unusable data eclipses the capacities of your data science team. In fact, probabilistically, that time is already here; due to the overwhelming and unfathomable amount of data created daily, there’s no way to keep tabs on every source, update, and segment of the market, and the odds dictate that there’s data you aren’t tapping into that could enhance your solutions and deepen your analytical capacities.
How Do I Manage Data Variety at Scale?
We’ve written about how critical a DataOps strategy is if your company wants to be data-driven. Without automation on your side, it’s only a matter of time before any data science team plateaus. Your team needs to be able to offload the ‘dirty work’ to ensure that their time is evenly distributed among all of the moving parts of data science, data engineering, and data analytics.
With data science being one of the most in-demand specialties in the world right now, the value in data is obvious. Investing in DataOps, however, will not only relieve the bottleneck in the sourcing, prep, and processing stages of data, but it will free your data scientists to source new data, uncover new insights, present more meaningful analysis more quickly, and if all goes according to plan, drive new revenue.
A Shift in Focus
It’s easy to pin data science woes on a talent shortage, but growing a data team will only go so far. No matter how deep the talent pool, it doesn’t make business sense to have one-to-one growth on data products and data professionals.
For any company looking to take advantage of the world of available data, the number one priority must be creating the simplest workflows to get data from anywhere into your organization, automating the repetitive and time-consuming tasks that hamper productivity.
That’s how you turn the massive world of available data into accessible data.