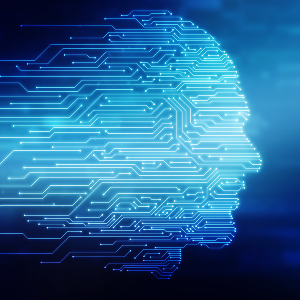
Today’s data-driven economy relies heavily on AI and ML systems that can sift through mountains of information to find actionable insights and power crucial strategic choices. Standardized, freely available data is essential for the widespread use of AI and ML technologies. Data virtualization is appealing because it eliminates the need to store data physically. Business data management is being revolutionized by virtualization, which presents a consolidated view of data from several sources in real time.
This eliminates the need for massive data transfers. It paves the way for more advanced AI and machine learning systems to evaluate and anticipate more nuanced events. There has been a lot of development in ML and AI recently.
In this article, we’ll go further into the part data virtualization plays in this procedure, showing how it helps eliminate data silos, implement real-time processing, boost agility, ensure security, and save expenses. In today’s competitive business market, data virtualization is helping the disruptive impact of AI and ML by lowering barriers between different data types.
Why and When Should You Virtualize Your Data?
Data virtualization allows uniform access, integration, and presentation of data from several sources. In the realm of data management, this approach represents the cutting edge.
The requirement to move or transfer data to a server in a data center is eliminated with data virtualization. Instead, it builds a theoretical wall around the information, making it invisible.
In this way, AI and ML systems can mix data from several sources without being limited by the locations of those sources or the details of their original data formats. Virtualization paves the way for applying AI and machine learning across whole datasets by creating a consistent and real-time representation of the data environment. This allows for enhanced comprehension and productivity.
1. Improving Data Availability
AI and ML projects may need help to obtain enough data. Virtualization’s removal of data silos makes modern algorithms so simple. Data scientists, analysts, and AI-ML development services providers may benefit from less data preparation and more analysis.
Since members of different teams may communicate data to improve an artificial intelligence or machine learning project, cooperation is possible. Businesses that can readily adjust to client preferences will succeed.
2. Processing of Data in Real Time
It is essential that the data be up to date for AI and ML to function correctly. Virtualizing data sources might make them available for near-real-time analysis. Monitoring a customer’s online behavior, processing digital transactions, and assessing sensor data all need this information.
Predictions and suggestions made by AI and ML systems may gain precision as fresh data becomes available. Thanks to data virtualization, businesses can now analyze data in real-time, speeding up the process by which they identify possibilities and overcome obstacles.
3. Future-Proofing Data Integration
New data sources and formats emerge as technology evolves. Data virtualization’s flexible design ensures data integration will adapt to new technologies.
Data virtualization lets firms seamlessly integrate data from IoT devices, social media platforms, and developing databases into AI and ML projects. Adaptability is essential for keeping ahead in technology and using data analytics advances.
4. Leanness and Scalability
Due to their inflexibility, traditional data integration approaches may be harmful in today’s dynamic corporate environment. When adjusting business processes to account for shifting data environments, data virtualization technologies shine. Data virtualization shortens the time it takes to create AI and ML systems by making it simpler to include new data sources and upgrade current ones.
These highly adaptable systems simplify dealing with the massive amounts of data produced by today’s software. Since AI and ML-based systems can be easily scaled horizontally, they may deal with ever-increasing datasets with little performance degradation.
5. Policymaking and Data Verification
AI and ML systems handle sensitive data; hence, data security and governance are essential. Data virtualization solutions protect private data through authentication, authorization, and encryption.
They also enable governance controls and data origin tracking for accurate records and compliance. Businesses that want AI and ML without compromising data security or regulatory compliance must leverage virtualization solutions.
6. Smart Budgeting
Due to hardware, software, and upkeep, traditional data integration methods are expensive. Data virtualization may save costs in redundant storage and time-consuming ETL procedures.
If firms could better utilize and access their data, they might save money. Data virtualization lowers costs and boosts ROI, making AI and ML projects more sustainable.
7. Enhancing Customer Experiences
AI and ML improve customer experiences, personalized suggestions, and preference prediction. Data virtualization helps firms unify client data from several touchpoints.
By understanding consumer behavior holistically, companies may customize goods and services to maximize customer happiness and loyalty. Data virtualization gives AI and ML algorithms access to the customer journey, enabling organizations to make data-driven choices that improve customer experiences.
Conclusion
AI and ML systems optimize internal data use via data virtualization. AI/ML functions better with plenty of real-time data, cheaper expenses, flexibility, and security. Data virtualization is essential in today’s data-driven economy as companies use cutting-edge analytics and AI.